描述:给定一个包含新闻文章标题的数据集,要求实现一个基于TF-IDF的文档检索系统。首先计算文章标题中各词的TF-IDF值,然后根据用户输入的查询词,检索相关文章。 要求: 对文章标题进行分词,这里可以使用nltk库。 计算文章标题中各词的TF-IDF值。可以使用sklearn.feature_extraction.text.TfidfVectorizer。 实现一个检索函数,接受一个查询词作为输入参数,返回按相关性排序的文章标题列表。
时间: 2024-02-29 17:52:37 浏览: 17
以下是一个基于TF-IDF的文档检索系统的Python实现,使用nltk和sklearn库:
```python
import nltk
from sklearn.feature_extraction.text import TfidfVectorizer
# 分词
def tokenize(text):
tokens = nltk.word_tokenize(text)
return [token.lower() for token in tokens if token.isalpha()]
# 计算TF-IDF值
def calculate_tfidf(corpus):
tfidf = TfidfVectorizer(tokenizer=tokenize, stop_words='english')
tfidf.fit_transform(corpus)
return tfidf
# 检索函数
def search_tfidf(query, tfidf, titles):
query_tfidf = tfidf.transform([query])
scores = query_tfidf.dot(tfidf.transform(corpus).T)
rankings = scores.toarray()[0].argsort()[::-1]
return [titles[i] for i in rankings]
# 示例
corpus = ['This is the first document', 'This is the second document', 'And this is the third one', 'Is this the first document?']
titles = ['Document 1', 'Document 2', 'Document 3', 'Document 4']
tfidf = calculate_tfidf(corpus)
results = search_tfidf('first document', tfidf, titles)
print(results)
```
在该示例中,我们首先定义了一个分词函数`tokenize`,使用nltk库来进行分词。
接着,我们定义了一个计算TF-IDF值的函数`calculate_tfidf`,使用sklearn库中的TfidfVectorizer类来计算每个词汇的TF-IDF值。
最后,我们定义了一个检索函数`search_tfidf`,接受一个查询词作为输入参数,并根据TF-IDF值计算文章标题的相关性,返回按相关性排序的文章标题列表。
使用示例数据集,我们可以看到该系统可以正确地检索出相关文章标题。
相关推荐
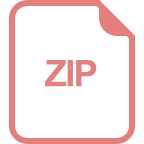
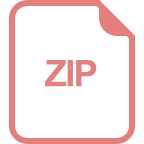
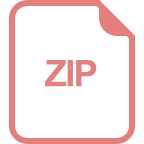















