error_factor=np.random.rand(2**cluster_num, 1) * 2*error + 1-error psi_temp=psig_HA_gBg*error_factor psig_HA_gBg=psi_temp*1.0 / np.sqrt(np.dot(psi_temp.conj().T, psi_temp)[0][0])物理意义是什么?
时间: 2023-04-07 08:01:59 浏览: 162
这段代码是在进行量子态的生成和调整,其中error_factor是一个随机数矩阵,用于引入一定的误差,psi_temp是通过将psig_HA_gBg与error_factor相乘得到的新的量子态,最后通过归一化得到了新的psig_HA_gBg。具体的物理意义需要根据具体的背景和应用场景来确定。
相关问题
逐句解释error_factor=np.random.rand(2**cluster_num, 1) * 2*error + 1-error psi_temp=psig_HA_gBg*error_factor psig_HA_gBg=psi_temp*1.0 / np.sqrt(np.dot(psi_temp.conj().T, psi_temp)[0][0])
这是一段 Python 代码,其中使用了 numpy 库中的随机数生成函数 np.random.rand(),生成了一个大小为 2 的 cluster_num 次方乘以 1 的矩阵 error_factor,并将其乘以 2*error-1,得到一个大小相同的矩阵。接着,使用 psig_HA_gBg 与 error_factor 相乘,得到一个新的矩阵 psi_temp。最后,将 psi_temp 除以其本身的模长,得到一个单位向量 psig_HA_gBg。
阅读全文
相关推荐
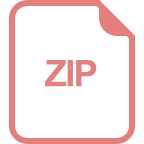
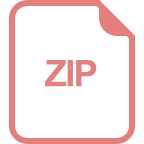
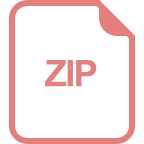
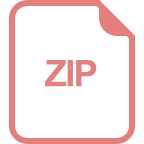
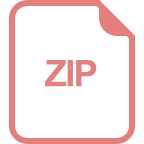
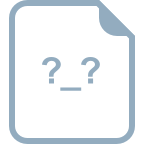
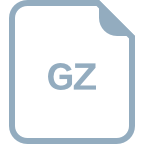
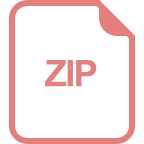
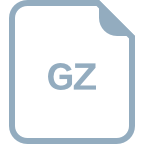
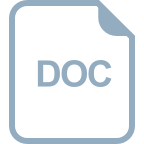
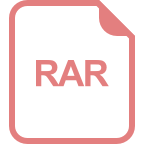
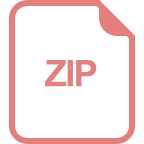
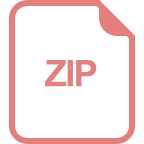
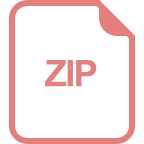
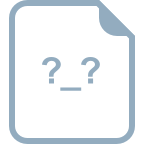
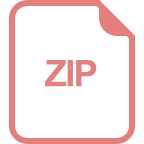
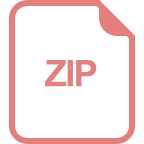
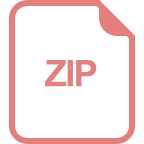
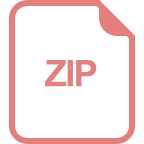