transformer图像分割代码
时间: 2023-07-08 12:04:58 浏览: 73
以下是一个基于transformer的图像分割代码示例:
```python
import torch
import torch.nn as nn
import torch.nn.functional as F
class Transformer(nn.Module):
def __init__(self, in_channels, out_channels, num_heads, hidden_dim, num_layers):
super(Transformer, self).__init__()
self.in_channels = in_channels
self.out_channels = out_channels
self.num_heads = num_heads
self.hidden_dim = hidden_dim
self.num_layers = num_layers
self.transformer_encoder = nn.TransformerEncoder(
nn.TransformerEncoderLayer(d_model=in_channels, nhead=num_heads, dim_feedforward=hidden_dim),
num_layers=num_layers)
self.conv1 = nn.Conv2d(in_channels, hidden_dim, kernel_size=3, padding=1)
self.conv2 = nn.Conv2d(hidden_dim, out_channels, kernel_size=1)
def forward(self, x):
# input shape: [batch_size, in_channels, height, width]
# apply 3x3 convolution
x = F.relu(self.conv1(x))
# reshape to fit transformer input shape
x = x.permute(2, 3, 0, 1) # [height, width, batch_size, hidden_dim]
x = x.reshape(x.shape[0]*x.shape[1], x.shape[2], x.shape[3]) # [height*width, batch_size, hidden_dim]
# apply transformer
x = self.transformer_encoder(x)
# reshape to fit convolutional output shape
x = x.reshape(x.shape[0]//self.num_heads, self.num_heads, x.shape[1], x.shape[2]) # [height*width, num_heads, batch_size//num_heads, hidden_dim//num_heads]
x = x.permute(2, 3, 0, 1) # [batch_size//num_heads, hidden_dim//num_heads, height, width, num_heads]
x = x.reshape(x.shape[0], x.shape[1], x.shape[2], x.shape[3]*x.shape[4]) # [batch_size//num_heads, hidden_dim//num_heads, height, width*num_heads]
# apply 1x1 convolution
x = self.conv2(x)
return x
```
该模型包含一个Transformer编码器和两个卷积层,以将输入图像转换为分割掩码。在前向传递期间,输入图像首先通过一个3x3卷积层,然后转换为适合Transformer输入形状的张量。接下来,将该张量输入Transformer编码器进行变换。最后,将输出张量转换为适合卷积层的形状,并通过1x1卷积层生成分割掩码。
使用此代码示例时,您应该将其与其他训练代码和数据集集成,并根据您的实际需求进行调整。
相关推荐
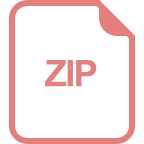














