soft Dice loss使用pytorch实现
时间: 2023-09-18 15:13:57 浏览: 74
Soft Dice Loss 是一种常用的分割损失函数,可以用于医学图像分割、目标检测等任务。PyTorch中可以使用以下代码实现 Soft Dice Loss:
```python
import torch
def soft_dice_loss(y_true, y_pred, epsilon=1e-6):
intersection = torch.sum(y_true * y_pred)
union = torch.sum(y_true) + torch.sum(y_pred)
dice_score = (2 * intersection + epsilon) / (union + epsilon)
dice_loss = 1 - dice_score
return dice_loss
```
其中,y_true 是真实标签,y_pred 是模型的预测结果。epsilon 是平滑因子,防止分母为零。函数首先计算交集和并集,然后计算 Dice 分数和 Dice 损失。最后返回 Dice 损失。
使用时,可以将其作为 PyTorch 的损失函数使用:
```python
loss_fn = soft_dice_loss
loss = loss_fn(y_true, y_pred)
```
相关问题
diceloss的pytorch代码
### 回答1:
Dice Loss是一种常用的图像分割损失函数,其代码实现如下:
```python
import torch
class DiceLoss(torch.nn.Module):
def __init__(self):
super(DiceLoss, self).__init__()
def forward(self, input, target):
smooth = 1.
iflat = input.view(-1)
tflat = target.view(-1)
intersection = (iflat * tflat).sum()
return 1 - ((2. * intersection + smooth) /
(iflat.sum() + tflat.sum() + smooth))
```
其中,`input`为模型输出的预测结果,`target`为真实标签。`smooth`是一个平滑项,用于避免分母为的情况。`iflat`和`tflat`将`input`和`target`展平成一维向量,方便计算交集和并集。最后返回的是Dice Loss的值。
### 回答2:
Dice Loss是一种常用的分割模型的损失函数,它的主要优点是能够提高模型在边缘处的预测准确度,并且能够缓解类别不平衡问题,因此在实际应用中得到了广泛的应用。下面我们来介绍一下如何在Pytorch中实现Dice Loss。
首先,我们需要导入相关的库:
```python
import torch
import torch.nn.functional as F
```
然后,我们定义Dice Loss的计算方式。Dice Loss的计算方式是将预测值和真实值的重合部分除以它们的总和,最后将得到的结果乘以2。我们可以定义如下的函数来实现:
```python
def dice_loss(output, target, smooth=1):
output = torch.sigmoid(output)
tp = torch.sum(output * target, dim=(2, 3))
fp = torch.sum(output * (1 - target), dim=(2, 3))
fn = torch.sum((1 - output) * target, dim=(2, 3))
dice = (2 * tp + smooth) / (2 * tp + fp + fn + smooth)
return 1 - dice.mean()
```
其中,output是模型的输出,target是真实标签。smooth是一个平滑参数,用于避免分母为0的情况。在计算中,我们首先对输出值进行二值化,然后计算重合部分的True Positive(tp)、False Positive(fp)和False Negative(fn),最后根据Dice Loss的计算公式得到损失值。
这里我们使用了torch.sum函数来计算tp、fp和fn,dim参数指定对哪些维度求和。在这里,我们将维度2和3作为batch内的每张图片的高和宽维度,具体来说,我们将分别对这两个维度进行求和,得到的结果将是每张图片的tp、fp和fn的总和。最后,我们将求得的dice值取平均值,得到最终的损失值。
最后,我们将Dice Loss加入到我们的训练过程中即可:
```python
loss_fn = dice_loss
optimizer = torch.optim.Adam(model.parameters(), lr=0.001)
for epoch in range(num_epochs):
for i, (images, labels) in enumerate(train_loader):
images = images.to(device)
labels = labels.to(device)
optimizer.zero_grad()
outputs = model(images)
loss = loss_fn(outputs, labels)
loss.backward()
optimizer.step()
if (i+1) % 10 == 0:
print (f'Epoch [{epoch+1}/{num_epochs}], Step [{i+1}/{total_step}], Loss: {loss.item():.4f}')
```
在此处,我们使用Adam优化器进行模型参数的更新,同时将dice_loss函数作为损失函数,通过反向传播自动计算梯度并更新参数。在训练过程中,我们可以打印出目前的损失值,以便于观察训练效果。
### 回答3:
Dice Loss是一种在图像分割领域广泛应用的一种损失函数,可以度量模型的精度,用于优化模型。在PyTorch中,通过自定义损失函数,可以实现Dice Loss。下面是一个Dice Loss的PyTorch代码作为参考:
```python
import torch
def dice_loss(output, target):
smooth = 1
output = output.flatten()
target = target.flatten()
intersection = (output * target).sum()
dice_score = (2 * intersection + smooth) / (output.sum() + target.sum() + smooth)
return 1 - dice_score
# 使用方法
output = torch.randn(1, 3, 256, 256)
target = torch.randn(1, 3, 256, 256)
loss = dice_loss(output, target)
print('Dice Loss:', loss)
```
在上面的代码中,首先定义了一个`dice_loss`函数,输入为两个张量`output`和`target`,输出为Dice Loss的值。然后在函数内部,计算两个张量的交集和并集,计算Dice Score,并返回1减去Dice Score的值,即为Dice Loss。
为了方便使用,我们还可以将`dice_loss`函数加入到训练模型之中,如下所示:
```python
import torch
import torch.nn as nn
class MyModel(nn.Module):
def __init__(self):
super(MyModel, self).__init__()
self.conv1 = nn.Conv2d(3, 64, 3, padding=1)
self.conv2 = nn.Conv2d(64, 64, 3, padding=1)
self.conv3 = nn.Conv2d(64, 3, 3, padding=1)
def forward(self, x):
x = F.relu(self.conv1(x))
x = F.relu(self.conv2(x))
x = torch.sigmoid(self.conv3(x))
return x
model = MyModel()
criterion = dice_loss
# 使用方法
output = model(input)
target = target
loss = criterion(output, target)
print('Dice Loss:', loss)
```
在这个例子中,我们定义了一个MyModel类,包含三个2D卷积层。在训练过程中,我们使用Dice Loss,将其赋值给criterion变量。然后,在模型MyModel中,我们将最后的输出结果通过`sigmoid`函数作用,使其变为0到1之间的概率值。最后,我们在训练过程中,调用criterion函数,获得Dice Loss值。
以上就是一个Dice Loss的PyTorch代码,可以根据需求进行修改和使用。这里提供参考,希望对你有所帮助。
Dice系数的pytorch实现
以下是Dice系数的PyTorch实现:
```python
import torch
def dice_coefficient(y_pred, y_true, smooth=1):
y_pred = torch.sigmoid(y_pred)
y_true = y_true.float()
intersection = torch.sum(y_pred * y_true)
dice = (2 * intersection + smooth) / (torch.sum(y_pred) + torch.sum(y_true) + smooth)
return dice
```
其中,y_pred表示模型预测的输出,y_true表示真实的标签,smooth是一个平滑因子,用于避免除以0的情况。在函数内部,首先将y_pred经过sigmoid函数转换为0到1之间的概率值,然后计算交集、并集和Dice系数。
使用时,可以将上述代码放入自己的PyTorch模型中,然后在训练过程中调用该函数计算Dice系数作为评估指标。
相关推荐
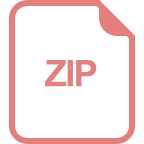
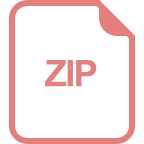
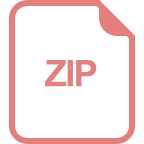












