bp神经网络回归relu函数初始化模型参数代码
时间: 2023-08-07 08:02:58 浏览: 91
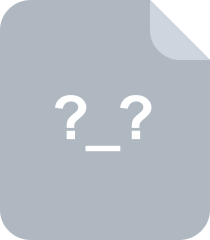
小波神经网络参数初始化函数
以下是使用PyTorch实现BP神经网络回归并使用ReLU函数进行参数初始化的示例代码:
```python
import torch
import torch.nn as nn
# 定义BP神经网络回归模型
class Net(nn.Module):
def __init__(self, input_size, hidden_size, output_size):
super(Net, self).__init__()
self.fc1 = nn.Linear(input_size, hidden_size)
self.fc2 = nn.Linear(hidden_size, output_size)
self.relu = nn.ReLU()
def forward(self, x):
out = self.fc1(x)
out = self.relu(out)
out = self.fc2(out)
return out
# 初始化模型参数
def weights_init(m):
classname = m.__class__.__name__
if classname.find('Linear') != -1:
n = m.in_features + m.out_features
std = np.sqrt(2.0 / n)
m.weight.data.normal_(0, std)
m.bias.data.fill_(0)
# 示例数据
x = torch.randn(10, 5)
y = torch.randn(10, 1)
# 初始化模型
model = Net(5, 10, 1)
model.apply(weights_init)
# 定义损失函数和优化器
criterion = nn.MSELoss()
optimizer = torch.optim.SGD(model.parameters(), lr=0.01)
# 训练模型
for epoch in range(100):
# 前向传播
outputs = model(x)
loss = criterion(outputs, y)
# 反向传播并优化
optimizer.zero_grad()
loss.backward()
optimizer.step()
# 打印损失值
print('Epoch [{}/{}], Loss: {:.4f}'.format(epoch+1, 100, loss.item()))
```
在上述代码中,weights_init函数使用了Xavier初始化方法来初始化模型参数。在初始化Linear层的权重和偏置时,需要根据输入维度和输出维度计算标准差,然后从一个正态分布中随机生成数值作为初值。最后,利用apply函数将初始化函数应用到整个模型上。
阅读全文
相关推荐
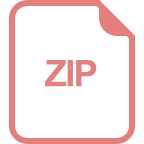















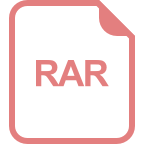