self.src_emb = nn.Linear(5, d_model)
时间: 2024-05-31 09:10:58 浏览: 201
这行代码是在一个 PyTorch 模型中定义了一个线性层(`nn.Linear`),它的输入维度是 5,输出维度是 `d_model`。这个线性层可以用来将一个维度为 5 的输入向量转换为一个维度为 `d_model` 的向量。在模型的前向传播过程中,输入向量会经过这个线性层,生成一个新的向量作为模型的输入。
相关问题
Traceback (most recent call last): File "/home/yjy/Documents/code/MATP-with-HEAT/it_all_train.py", line 171, in <module> train_loss_ep = train_a_model(train_net, num_ep=ep) File "/home/yjy/Documents/code/MATP-with-HEAT/it_all_train.py", line 37, in train_a_model fut_pred = model_to_tr(data.to(args['device'])) File "/home/yjy/anaconda3/envs/MATP/lib/python3.7/site-packages/torch/nn/modules/module.py", line 889, in _call_impl result = self.forward(*input, **kwargs) File "/home/yjy/Documents/code/MATP-with-HEAT/it_heat_g_model.py", line 53, in forward fwd_Hist_Enc = self.RNN_Encoder(data_pyg.x, data_pyg.veh_mask, data_pyg.ped_mask) # Encode File "/home/yjy/Documents/code/MATP-with-HEAT/it_base_model.py", line 39, in RNN_Encoder _, veh_Hist_Enc = self.veh_enc_rnn(self.leaky_relu(self.ip_emb(Hist))) File "/home/yjy/anaconda3/envs/MATP/lib/python3.7/site-packages/torch/nn/modules/module.py", line 889, in _call_impl result = self.forward(*input, **kwargs) File "/home/yjy/anaconda3/envs/MATP/lib/python3.7/site-packages/torch/nn/modules/linear.py", line 94, in forward return F.linear(input, self.weight, self.bias) File "/home/yjy/anaconda3/envs/MATP/lib/python3.7/site-packages/torch/nn/functional.py", line 1753, in linear return torch._C._nn.linear(input, weight, bias) RuntimeError: mat1 and mat2 shapes cannot be multiplied (2320x4 and 128x32)
这个错误是由于你的代码中进行了矩阵乘法操作,但是两个矩阵的维度不匹配。根据错误信息,第一个矩阵的大小是2320x4,而第二个矩阵的大小是128x32。矩阵乘法要求第一个矩阵的列数与第二个矩阵的行数相等。
要解决这个问题,你需要检查你的代码中涉及到矩阵乘法的部分,确保两个矩阵的维度是匹配的。你可以使用`torch.Size`或者`shape`属性来检查矩阵的维度,并且根据需要进行调整。
另外,你还可以使用一些其他的矩阵操作,如`torch.matmul()`或者`torch.mm()`来执行矩阵乘法操作,这些函数会自动进行维度匹配检查。
如果你需要更具体的帮助,请提供相关的代码片段和更多细节信息。
填写以下代码,并给出详细解释Hypothesis = namedtuple('Hypothesis', ['value', 'score']) class NMT(nn.Module): """ Simple Neural Machine Translation Model: - Bidrectional LSTM Encoder - Unidirection LSTM Decoder - Global Attention Model (Luon
The code you provided defines a named tuple `Hypothesis` with two fields, `value` and `score`. This is a convenient way to store and manipulate hypotheses in the context of sequence-to-sequence models.
The `NMT` class is a PyTorch module that implements a simple neural machine translation model. It consists of a bidirectional LSTM encoder, a unidirectional LSTM decoder, and a global attention mechanism based on Luong et al. (2015).
Here's a breakdown of the code:
```python
from collections import namedtuple
import torch
import torch.nn as nn
import torch.nn.functional as F
Hypothesis = namedtuple('Hypothesis', ['value', 'score'])
class NMT(nn.Module):
def __init__(self, src_vocab_size, tgt_vocab_size, emb_size, hidden_size):
super(NMT, self).__init__()
self.src_embed = nn.Embedding(src_vocab_size, emb_size)
self.tgt_embed = nn.Embedding(tgt_vocab_size, emb_size)
self.encoder = nn.LSTM(emb_size, hidden_size, bidirectional=True)
self.decoder = nn.LSTMCell(emb_size + hidden_size, hidden_size)
self.attention = nn.Linear(hidden_size * 2, hidden_size)
self.out = nn.Linear(hidden_size, tgt_vocab_size)
self.hidden_size = hidden_size
def forward(self, src, tgt):
batch_size = src.size(0)
src_len = src.size(1)
tgt_len = tgt.size(1)
# Encode the source sentence
src_embedded = self.src_embed(src)
encoder_outputs, (last_hidden, last_cell) = self.encoder(src_embedded)
# Initialize the decoder states
decoder_hidden = last_hidden.view(batch_size, self.hidden_size)
decoder_cell = last_cell.view(batch_size, self.hidden_size)
# Initialize the attention context vector
context = torch.zeros(batch_size, self.hidden_size, device=src.device)
# Initialize the output scores
outputs = torch.zeros(batch_size, tgt_len, self.hidden_size, device=src.device)
# Decode the target sentence
for t in range(tgt_len):
tgt_embedded = self.tgt_embed(tgt[:, t])
decoder_input = torch.cat([tgt_embedded, context], dim=1)
decoder_hidden, decoder_cell = self.decoder(decoder_input, (decoder_hidden, decoder_cell))
attention_scores = self.attention(encoder_outputs)
attention_weights = F.softmax(torch.bmm(attention_scores, decoder_hidden.unsqueeze(2)).squeeze(2), dim=1)
context = torch.bmm(attention_weights.unsqueeze(1), encoder_outputs).squeeze(1)
output = self.out(decoder_hidden)
outputs[:, t] = output
return outputs
```
The `__init__` method initializes the model parameters and layers. It takes four arguments:
- `src_vocab_size`: the size of the source vocabulary
- `tgt_vocab_size`: the size of the target vocabulary
- `emb_size`: the size of the word embeddings
- `hidden_size`: the size of the encoder and decoder hidden states
The model has four main components:
- `src_embed`: an embedding layer for the source sentence
- `tgt_embed`: an embedding layer for the target sentence
- `encoder`: a bidirectional LSTM encoder that encodes the source sentence
- `decoder`: a unidirectional LSTM decoder that generates the target sentence
The attention mechanism is implemented in the `forward` method. It takes two arguments:
- `src`: the source sentence tensor of shape `(batch_size, src_len)`
- `tgt`: the target sentence tensor of shape `(batch_size, tgt_len)`
The method first encodes the source sentence using the bidirectional LSTM encoder. The encoder outputs and final hidden and cell states are stored in `encoder_outputs`, `last_hidden`, and `last_cell`, respectively.
The decoder is initialized with the final hidden and cell states of the encoder. At each time step, the decoder takes as input the embedded target word and the context vector, which is a weighted sum of the encoder outputs based on the attention scores. The decoder output and hidden and cell states are updated using the LSTMCell module.
The attention scores are calculated by applying a linear transform to the concatenated decoder hidden state and encoder outputs, followed by a softmax activation. The attention weights are used to compute the context vector as a weighted sum of the encoder outputs.
Finally, the decoder hidden state is passed through a linear layer to produce the output scores for each target word in the sequence. The output scores are stored in the `outputs` tensor and returned by the method.
阅读全文
相关推荐







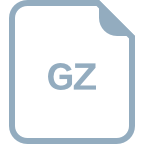


