Attention Mechanism and Multilayer Perceptrons (MLP): A New Perspective on Feature Extraction, Unearthing Data Value, and Enhancing Model Comprehension
发布时间: 2024-09-15 08:09:57 阅读量: 21 订阅数: 27 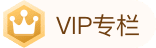
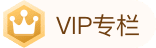
# 1. Overview of Attention Mechanism**
The attention mechanism is a neural network technique that allows the model to focus on specific parts of the input data. By assigning weights, the attention mechanism can highlight important features while suppressing irrelevant information.
The benefits of the attention mechanism include:
* Improving the accuracy and efficiency of feature extraction
* Enhancing the model's understanding of relevance in the input data
* Increasing the model's interpretability, allowing researchers to understand the areas that the model focuses on
# 2. Applications of Attention Mechanism in Feature Extraction
The attention mechanism is a neural network technique that allows the model to concentrate on the most important parts of the input data. In feature extraction, the attention mechanism can help the model identify and extract key features relevant to specific tasks from the data.
### 2.1 Self-Attention Mechanism
The self-attention mechanism is a type of attention mechanism that allows the model to focus on different parts of an input sequence. It works by calculating the similarity between each element and all other elements in the sequence. Elements with higher similarity scores are given higher weights, while those with lower similarity scores are given lower weights.
**2.1.1 Principles of Self-Attention Mechanism**
The principles of the self-attention mechanism can be represented by the following formula:
```
Q = W_qX
K = W_kX
V = W_vX
A = softmax(Q^T K / sqrt(d_k))
Output = AV
```
Where:
* X is the input sequence
* Q, K, V are the query, key, and value matrices
* W_q, W_k, W_v are weight matrices
* d_k is the dimension of the key vector
* A is the attention weight matrix
* Output is the weighted sum output
**2.1.2 Applications of Self-Attention Mechanism**
The self-attention mechanism has been successfully applied to various feature extraction tasks, including:
* Text feature extraction: The self-attention mechanism can identify important words and phrases in text sequences.
* Image feature extraction: The self-attention mechanism can identify important regions and objects in images.
* Audio feature extraction: The self-attention mechanism can identify important phonemes and rhythms in audio sequences.
### 2.2 Heterogeneous Attention Mechanism
The heterogeneous attention mechanism is a type of attention mechanism that allows the model to focus on the relationship between an input sequence and another sequence. It works by calculating the similarity between each element in the input sequence and each element in another sequence. Elements with higher similarity scores are given higher weights, while those with lower similarity scores are given lower weights.
**2.2.1 Principles of Heterogeneous Attention Mechanism**
The principles of the heterogeneous attention mechanism can be represented by the following formula:
```
Q = W_qX
K = W_kY
V = W_vY
A = softmax(Q^T K / sqrt(d_k))
Output = AV
```
Where:
* X is the input sequence
* Y is another sequence
* Q, K, V are the query, key, and value matrices
* W_q, W_k, W_v are weight matrices
* d_k is the dimension of the key vector
* A is the attention weight matrix
* Output is the weighted sum output
**2.2.2 Applications of Heterogeneous Attention Mechanism**
The heterogeneous attention mechanism has been successfully applied to various feature extraction tasks, including:
* Machine translation: The heterogeneous attention mechanism can help the model focus on the relationship between the source language sequence and the target language sequence.
* Image caption generation: The heterogeneous attention mechanism can help the model focus on the relationship between images and text descriptions.
* Speech recognition: The heterogeneous attention mechanism can help the model focus on the relationship between audio sequences and text transcripts.
# 3. Overview of Multilayer Perceptrons (MLPs)
**3.1 Architecture of MLPs**
A multilayer perceptron (MLP) is a feedforward neural network composed of multiple fully connected layers. Each fully connected layer consists of a linear transformation followed by a nonlinear activation function. The typical architecture of an MLP is as follows:
```
Input layer -> Hidden layer 1 -> Hidden layer 2 -> ... -> Output layer
```
Where the input layer receives the input data and the output layer produces the final prediction. The hidden layers are responsible for extracting features from the input data and performing nonlinear transformations.
**3.2 Principles of MLPs**
The working principles of MLPs can be summarized as follows:
1. Input data enters the network through the input layer.
2. Each hidden layer performs a linear transformation on the input data, i.e., calculates the weighted sum.
3. The result of the linear transformation goes through a nonlinear activation function, introducing nonlinearity.
4. The output of the nonlinear activation function serves as the input for the next layer.
5. Repeat steps 2-4 until reaching the output layer.
6. The output layer produces the final prediction, usually a probability distribution or continuous values.
**3.3 Activation Functions in MLPs**
Common activation functions used in MLPs include:
***ReLU (Rectified Linear Unit)**: `max(0, x)`
***Sigmoid**: `1 / (1 + exp(-x))`
***Tanh**: `(exp(x) - exp(-x)) / (exp(x) + exp(-x))`
**3.4 Advantages of MLPs**
MLPs have the following advantages:
***Simplicity and ease of use**: The architecture of MLPs is simple and easy to understand and implement.
***Strong generalization ability**: MLPs are capable of learning complex relationships from data, exhibiting strong generalization.
***Good scalability**: MLPs can add or remove hidden layers as needed to accommodate different task complexities.
**3.5 Limitations of MLPs**
MLPs also have some limitations:
***High computational requirements**: The computational load of MLPs increases with the number of hidden layers and neurons.
***Prone to overfitting**: MLPs are prone to overfitting and require careful hyperparameter tuning a
0
0
相关推荐
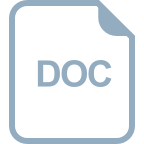
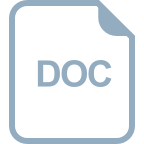
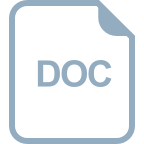
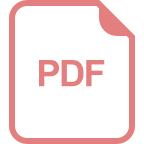
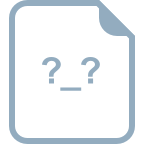
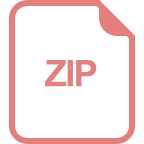
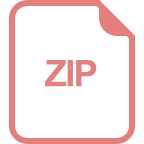
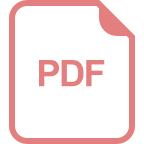
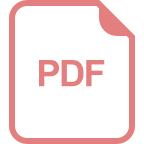