Demystifying Multilayer Perceptrons (MLP): Architecture, Principles, and Applications for Building Efficient Neural Networks
发布时间: 2024-09-15 07:55:27 阅读量: 5 订阅数: 10 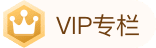
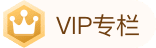
# 1. Multilayer Perceptron (MLP) Overview
A multilayer perceptron (MLP) is a type of feedforward artificial neural network that consists of multiple layers of perceptrons, with each layer processing the output from the previous layer. MLPs are simple in structure and easy to train, and they are widely used in various fields such as image classification, natural language processing, and financial forecasting.
The fundamental structure of an MLP includes an input layer, hidden layers, and an output layer. The input layer receives the input data, the hidden layers perform nonlinear transformations on the input data, and the output layer generates the final results. The forward propagation process in an MLP begins at the input layer and calculates layer by layer until the output layer is reached. Conversely, the backward propagation process starts at the output layer and computes gradients layer by layer until the inpu***
***monly used activation functions include sigmoid, tanh, and ReLU, while common loss functions include cross-entropy loss and mean squared error loss.
# 2. Architecture and Principles of MLP
### 2.1 Basic Structure of MLP
The multilayer perceptron (MLP) is a feedforward neural network composed of multiple fully connected layers stacked together. Its basic structure is illustrated in the following diagram:
```mermaid
graph LR
subgraph Input Layer
A[x1]
B[x2]
C[x3]
end
subgraph Hidden Layer 1
D[h1]
E[h2]
F[h3]
end
subgraph Hidden Layer 2
G[h4]
H[h5]
I[h6]
end
subgraph Output Layer
J[y]
end
A-->D
B-->D
C-->D
D-->G
E-->G
F-->G
G-->J
H-->J
I-->J
```
Each layer of an MLP consists of neurons that receive a weighted sum of outputs from the previous layer and generate output through an activation function.
### 2.2 Forward and Backward Propagation of MLP
**Forward Propagation**
Forward propagation is the process by which an MLP computes its output. For an input vector `x = [x1, x2, ..., xn]`, the calculation process of an MLP is as follows:
1. **Hidden Layer Computation:**
- Calculate the activation value `h_l` of hidden layer `l`:
```
h_l = σ(W_l * x + b_l)
```
- Where `W_l` is the weight matrix, `b_l` is the bias vector, and `σ` is the activation function.
2. **Output Layer Computation:**
- Calculate the activation value `y` of the output layer:
```
y = σ(W_out * h_L + b_out)
```
- Where `W_out` is the output layer weight matrix, and `b_out` is the output layer bias vector.
**Backward Propagation**
Backward propagation is the training process of an MLP. It updates weights and biases by computing gradients of the loss function.
1. **Compute Error:**
- Calculate the output layer error `δ_out`:
```
δ_out = (y - t) * σ'(W_out * h_L + b_out)
```
- Where `t` is the true label and `σ'` is the derivative of the activation function.
2. **Compute Hidden Layer Error:**
- Calculate the error `δ_l` of hidden layer `l`:
```
δ_l = (W_{l+1}^T * δ_{l+1}) * σ'(W_l * x + b_l)
```
3. **Update Weights and Biases:**
- Update weight matrix `W_l`:
```
W_l = W_l - α * δ_l * x^T
```
- Update bias vector `b_l`:
```
b_l = b_l - α * δ_l
```
- Where `α` is the learning rate.
### 2.3 Activation Functions and Loss Functions in MLP
**Activation Functions**
Common activation functions used in MLPs include:
- Sigmoid: `σ(x) = 1 / (1 + e^(-x))`
- Tanh: `σ(x) = (e^x - e^(-x)) / (e^x + e^(-x))`
- ReLU: `σ(x) = max(0, x)`
**Loss Functions**
Common loss functions used in MLPs include:
- Square Loss: `L(y, t) = (y - t)^2`
- Cross-Entropy Loss: `L(y, t) = -t * log(y) - (1 - t) * log(1 - y)`
# 3. Training and Optimization of MLP
### 3.1 Training Algorithms for MLP
The training process of an MLP is an iterative optimization process ***mon MLP training algorithms include:
- **Gradient Descent Algorithm:** The gradient descent algorithm updates weights and biases iteratively to gradually reduce the value of the loss function. In each iteration, the algorithm computes the gradients of the loss function with respect to weights and biases and updates them in the direction of the negative gradient.
- **Momentum Method:** The momentum method adds a momentum term to the gradient descent algorithm, accelerating convergence. The momentum term records the history of updates to weights and biases and combines this with the current gradient to update them.
- **RMSprop Algorithm:** RMSprop is a gradient descent algorithm with adaptive learning rates. It dynamically adjusts the learning rate by computing the root mean square (RMS) of gradients, effectively preventing overfitting.
- **Adam Algorithm:** The Adam algorithm combines the advantages of the RMSprop algorithm and the momentum method, providing adaptive learning rates and accelerating convergence speed.
### 3.2 Hyperparameter Tuning for MLP
Hyperparameters of an MLP include learning rate, batch size, activation function, regularization parameters, etc. The goal of hyperparameter tuning is ***mon hyperparameter tuning methods include:
- **Grid Search:** Grid search is an exhaustive search method that traverses the given range of hyperparameter values and selects the combination that minimizes the loss function on the validation set.
- **Random Search:** Random search is a probabilistic method that randomly selects hyperparameter combinations and chooses the one that minimizes the loss function on the validation set.
- **Bayesian Optimization:** Bayesian optimization is a method based on Bayes' theorem that constructs a probabilistic model of the hyperparameter space to guide the search process.
### 3.3 Regularization Techniques for MLP
Regu***mon regularization techniques include:
- **L1 Regularization:** L1 regularization adds the L1 norm of weights and biases to the loss function, which can sparsify them and prevent overfitting.
- **L2 Regularization:** L2 regularization adds the L2 norm of weights and biases to the loss function, which can smooth them and prevent overfitting.
- **Dropout:** Dropout is a technique that randomly deactivates a portion of neurons during training, preventing them from overfitting each other.
- **Data Augmentation:** Data augmentation is a method that increases the size of the training data by transforming it (e.g., rotating, cropping, flipping, etc.), preventing the model from overfitting.
# 4. Practical Applications of MLP
### 4.1 Application of MLP in Image Classification
MLP performs well in image classification tasks, with its powerful feature extraction capability enabling it to learn complex patterns from images.
**Application Scenarios:**
- Object Detection
- Image Recognition
- Image Segmentation
**Implementation:**
1. **Data Preprocessing:** Convert images into fixed-size arrays and perform normalization.
2. **MLP Model Construction:** Design the MLP network structure based on image features and classification categories, including the input layer, hidden layers, and output layer.
3. **Train the Model:** Train the MLP model on the training dataset, adjusting weights and biases to minimize the loss function.
4. **Evaluate the Model:** Use the validation dataset to assess the model's performance, including accuracy, recall, and F1 score.
### 4.2 Application of MLP in Natural Language Processing
MLP is also widely used in natural language processing (NLP) tasks, with its powerful text representation capability enabling it to understand the meaning of text.
**Application Scenarios:**
- Text Classification
- Sentiment Analysis
- Machine Translation
**Implementation:**
1. **Text Preprocessing:** Perform tokenization, part-of-speech tagging, and vectorization on the text.
2. **MLP Model Construction:** Design the MLP network structure based on text features and classification categories, including the input layer, hidden layers, and output layer.
3. **Train the Model:** Train the MLP model on the training dataset, adjusting weights and biases to minimize the loss function.
4. **Evaluate the Model:** Use the validation dataset to assess the model's performance, including accuracy, recall, and F1 score.
### 4.3 Application of MLP in Financial Forecasting
MLP also plays a significant role in financial forecasting tasks, with its nonlinear fitting capability enabling it to capture complex changes in financial data.
**Application Scenarios:**
- Stock Price Prediction
- Foreign Exchange Rate Prediction
- Economic Indicator Prediction
**Implementation:**
1. **Data Collection:** Collect historical financial data, including prices, trading volumes, economic indicators, etc.
2. **Feature Engineering:** Extract and process relevant features of financial data, such as moving averages and relative strength index (RSI).
3. **MLP Model Construction:** Design the MLP network structure based on financial data features and prediction targets, including the input layer, hidden layers, and output layer.
4. **Train the Model:** Train the MLP model on the training dataset, adjusting weights and biases to minimize the loss function.
5. **Evaluate the Model:** Use the validation dataset to assess the model's performance, including root mean square error (RMSE), mean absolute error (MAE), and maximum absolute error (MAE).
# 5.1 Convolutional Neural Networks (CNNs)
**Introduction**
A convolutional neural network (CNN) is a type of deep neural network specifically designed to process input with a grid-like data structure, ***pared to MLPs, CNNs have the following main advantages:
***Local Connectivity:** Neurons in a CNN are connected only to local regions of the input data, which aids in extracting local features.
***Weight Sharing:** Convolutional kernels in a CNN share weights across the entire input data, reducing the number of parameters and promoting translation invariance.
***Pooling Layers:** Pooling layers aggregate features from local regions, reducing the size of feature maps and enhancing robustness.
**CNN Architecture**
The typical architecture of a CNN includes the following layers:
***Convolutional Layer:** The convolutional layer uses convolutional kernels to extract features from the input data.
***Pooling Layer:** The pooling layer performs downsampling on the output of the convolutional layer, reducing the size of the feature maps.
***Fully Connected Layer:** The fully connected layer flattens the output of the convolutional layers and connects to the output layer.
**CNN Training**
***mon optimizers include Adam and RMSProp, while loss functions are typically cross-entropy loss or mean squared error loss.
**CNN Applications**
CNNs are widely used in image processing and computer vision, including:
* Image Classification
* Object Detection
* Semantic Segmentation
* Image Generation
**Example**
The following code example demonstrates a simple CNN architecture for image classification:
```python
import tensorflow as tf
# Define input data
input_data = tf.keras.Input(shape=(28, 28, 1))
# Convolutional Layer 1
conv1 = tf.keras.layers.Conv2D(32, (3, 3), activation='relu')(input_data)
# Pooling Layer 1
pool1 = tf.keras.layers.MaxPooling2D((2, 2))(conv1)
# Convolutional Layer 2
conv2 = tf.keras.layers.Conv2D(64, (3, 3), activation='relu')(pool1)
# Pooling Layer 2
pool2 = tf.keras.layers.MaxPooling2D((2, 2))(conv2)
# Flatten Layer
flatten = tf.keras.layers.Flatten()(pool2)
# Fully Connected Layer
dense1 = tf.keras.layers.Dense(128, activation='relu')(flatten)
# Output Layer
output = tf.keras.layers.Dense(10, activation='softmax')(dense1)
# Define model
model = tf.keras.Model(input_data, output)
# ***
***pile(optimizer='adam', loss='sparse_categorical_crossentropy', metrics=['accuracy'])
# Train model
model.fit(x_train, y_train, epochs=10)
```
**Logical Analysis**
This code example defines a CNN model with two convolutional layers, two pooling layers, and two fully connected layers. The convolutional layers extract features from the input images, while the pooling layers reduce the size of the feature maps and enhance robustness. The fully connected layers flatten the output of the convolutional layers and connect to the output layer, which uses the softmax activation function for multi-class classification.
# 6.1 Application of MLP in Edge Computing
With the rise of the Internet of Things (IoT) devices and edge computing, the application of MLPs in edge computing is increasingly gaining attention. Edge computing is a distributed computing paradigm that deploys computing and storage resources near the data source to reduce latency and improve efficiency.
MLP has the following advantages in edge computing:
- **Low Latency:** The computational complexity of MLPs is relatively low, allowing for rapid execution on edge devices and enabling low-latency real-time decision-making.
- **Low Power Consumption:** MLPs typically have smaller model sizes and require fewer computing resources, making them ideal for deployment on power-constrained edge devices.
- **High Adaptability:** MLPs can be customized for specific edge computing tasks, such as image classification, anomaly detection, and prediction.
In edge computing, MLPs can be used for the following applications:
- **Industrial Internet of Things (IIoT):** MLPs can be used for monitoring industrial equipment, detecting anomalies, and predicting maintenance needs.
- **Smart Home:** MLPs can control smart home devices, such as lights, thermostats, and security systems.
- **Autonomous Driving:** MLPs can process sensor data to make real-time decisions, such as object detection and path planning.
## 6.2 Innovative Applications of MLP in Artificial Intelligence
MLPs continue to evolve in the field of artificial intelligence (AI) and are used in a variety of innovative applications:
- **Generative Adversarial Networks (GANs):** MLPs are a key component in GANs, used for generating realistic data or images.
- **Reinforcement Learning:** MLPs can act as value functions or policy networks, guiding the behavior of reinforcement learning agents.
- **Neural Architecture Search (NAS):** MLPs can be used for automatically designing and optimizing neural network architectures.
- **Explainable Artificial Intelligence (XAI):** MLPs can be used to explain the predictions of complex neural network models, enhancing their transparency and trustworthiness.
As AI technology continues to advance, MLPs are expected to play an increasingly important role in the future, providing powerful learning and decision-making capabilities for a wide range of applications.
0
0
相关推荐
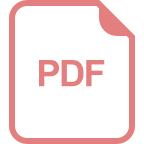
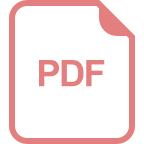
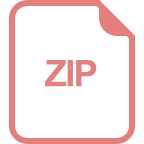
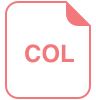
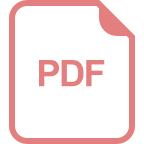
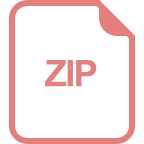
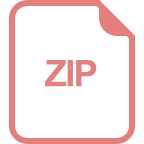
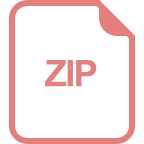