【Multilayer Perceptron (MLP) Deep Learning Guide】: From Basics to Advanced Applications, Unleashing AI Potential
发布时间: 2024-09-15 07:54:08 阅读量: 33 订阅数: 27 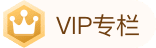
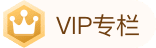
# A Guide to Multi-Layer Perceptrons (MLP): From Fundamentals to Advanced Applications
## 1. Theoretical Foundations of MLP
A Multi-Layer Perceptron (MLP) is a type of feedforward artificial neural network consisting of multiple layers of neurons. MLPs are used to solve various machine learning problems, including classification, regression, and generation tasks.
The basic structure of an MLP consists of an input layer, hidden layers, and an output layer. The input layer receives input data, the hidden layers perform nonlinear transformations, and the output layer generates the final output. The learning process of an MLP is conducted through the backpropagation algorithm, which updates the network weights by computing the gradients of the ***
***monly used activation functions include sigmoid, tanh, and ReLU. The loss function measures the difference between the model's predictions and the actual values, with common loss functions being Mean Squared Error and Cross-Entropy.
## 2. Programming Implementation of MLP
### 2.1 Structure and Algorithms of MLP
#### 2.1.1 Forward Propagation and Backpropagation Algorithms
A Multi-Layer Perceptron (MLP) is a feedforward neural network composed of an input layer, multiple hidden layers, and an output layer. The forward propagation algorithm calculates the network's output, while the backpropagation algorithm is used to update the network's weights and biases.
**Forward Propagation Algorithm**
1. Pass the input data to the input layer.
2. For each hidden layer:
- Compute the weighted sum of neurons: `z = w^Tx + b`
- Apply the activation function: `a = f(z)`
3. Pass the output to the output layer.
**Backpropagation Algorithm**
1. Calculate the error at the output layer: `δ = (y - a)`
2. For each hidden layer:
- Compute the error gradient: `δ = f'(z) * w^Tδ`
- Update the weights: `w = w - αδx`
- Update the biases: `b = b - αδ`
Where:
- `x` is the input data
- `y` is the target output
- `a` is the output of the neuron
- `w` is the weight
- `b` is the bias
- `α` is the learning rate
- `f` is the activation function
#### 2.1.2 Activation F***
***monly used activation functions include:
- Sigmoid: `f(x) = 1 / (1 + e^-x)`
- Tanh: `f(x) = (e^x - e^-x) / (e^x + e^-x)`
- ReLU: `f(x) = max(0, x)`
Loss functions measure the difference between the network'***mon loss functions include:
- Mean Squared Error: `L = (y - a)^2`
- Cross-Entropy: `L = -ylog(a) - (1 - y)log(1 - a)`
### 2.2 Training and Optimization of MLP
#### 2.2.1 Gradient Descent Algorithm and Parameter Updates
The gradient descent algorithm updates the network's weights and biases along the negative gradient direction of the error function to minimize the loss function.
**Gradient Descent Algorithm**
***pute the gradient of the error function: `∇L = (∂L/∂w, ∂L/∂b)`
2. Update weights: `w = w - α∇L_w`
3. Update biases: `b = b - α∇L_b`
Where:
- `α` is the learning rate
#### 2.2.2 Regularization and Hyperparamete***
***mon regularization techniques include:
- L1 Regularization: `L = L + λ||w||_1`
- L2 Regularization: `L = L + λ||w||_2^2`
Hyperparameter tuning is the process of adjusting hyperparameters like the learning rate and regularization parameters to optimize the network'***mon hyperparameter tuning methods include:
- Grid Search: Systematically trying combinations of hyperparameters.
- Bayesian Optimization: Using Bayesian optimization algorithms to optimize hyperparameters.
## 3. Practical Applications of MLP
### 3.1 Image Classification and Recognition
#### 3.1.1 Introduction to Convolutional Neural Networks (CNNs)
A Convolutional Neural Network (CNN) is a type of deep learning model specifically designed to process image data. Unlike MLPs, CNNs have a specialized structure that includes convolutional layers, pooling layers, and fully connected layers. Convolutional layers use convolution operations to extract features from images, while pooling layers reduce the size of feature maps through downsampling. Fully connected layers are similar to those in MLPs and are used for image classification.
#### 3.1.2 Application of MLP in Image Classification
MLPs can also be used for image classification tasks, but they are generally not as effective as CNNs. However, in certain cases, MLPs can still provide good performance, such as:
- **Small Datasets:** When the training dataset is small or the image size is small, MLPs may be more suitable than CNNs.
- **Specific Tasks:** For certain specific image classification tasks, such as handwritten digit recognition, MLPs may be more suitable than CNNs.
### 3.2 Natural Language Processing (NLP)
#### 3.2.1 Introduction to Recurrent Neural Networks (RNNs)
Recurrent Neural Networks (RNNs) are a type of deep learning model specifically designed for sequence data. Unlike MLPs, RNNs have recurrent connections, which allow them to remember previous inputs. This makes RNNs particularly suitable for processing natural language data, where the order of words is important.
#### 3.2.2 Application of MLP in NLP
MLPs can also be used for NLP tasks, but they are generally less effective than RNNs. However, in certain cases, MLPs can still provide good performance, such as:
- **Text Classification:** MLPs can be used to classify text documents, such as spam detection or sentiment analysis.
- **Language Modeling:** MLPs can be used to predict the next word in a given text sequence, which is useful for natural language generation and machine translation.
**Code Example:**
The following code example demonstrates how to use an MLP for image classification:
```python
import numpy as np
import tensorflow as tf
# Load image data
(x_train, y_train), (x_test, y_test) = tf.keras.datasets.mnist.load_data()
# Normalize image data
x_train = x_train.astype('float32') / 255.0
x_test = x_test.astype('float32') / 255.0
# Create an MLP model
model = tf.keras.Sequential([
tf.keras.layers.Flatten(input_shape=(28, 28)),
tf.keras.layers.Dense(128, activation='relu'),
tf.keras.layers.Dense(10, activation='softmax')
])
# ***
***pile(optimizer='adam', loss='sparse_categorical_crossentropy', metrics=['accuracy'])
# Train the model
model.fit(x_train, y_train, epochs=10)
# Evaluate the model
model.evaluate(x_test, y_test)
```
**Logical Analysis:**
- `tf.keras.datasets.mnist.load_data()` loads the MNIST dataset, which contains handwritten digit images.
- `astype('float32') / 255.0` normalizes the image data to float numbers between 0 and 1.
- `tf.keras.Sequential([...]` creates a sequential MLP model with an input layer, a hidden layer, and an output layer.
- `compile()` compiles the model, specifying the optimizer, loss function, and metric standards.
- `fit()` trains the model, updating the model's weights using training data.
- `evaluate()` evaluates the model, calculating accuracy and loss using test data.
## 4. Advanced Applications of MLP
### 4.1 Generative Adversarial Networks (GANs)
#### 4.1.1 Principles and Architecture of GANs
Generative Adversarial Networks (GANs) are a type of generative model consisting of two neural networks: a generator network and a discriminator network. The generator network is responsible for generating new data, while the discriminator network is responsible for distinguishing between generated data and real data.
The training process of a GAN is an adversarial process where the generator network tries to generate data that is indistinguishable from real data, and the discriminator network tries to differentiate between generated and real data. Through this adversarial training, the generator network gradually learns to generate realistic data, and the discriminator network becomes more accurate.
#### 4.1.2 Application of MLP in GANs
MLPs can serve as the generator network or the discriminator network in a GAN.
**As the Generator Network:** MLPs can generate various types of data, such as images, text, and audio. An MLP generator network typically consists of multiple hidden layers, each performing a nonlinear transformation. By adjusting the number and size of hidden layers, one can control the complexity and diversity of the generated data.
**As the Discriminator Network:** MLPs can classify generated data and real data. An MLP discriminator network typically consists of multiple hidden layers, each performing a nonlinear transformation. By adjusting the number and size of hidden layers, one can control the discriminative power of the discriminator network.
### 4.2 Reinforcement Learning
#### 4.2.1 Basic Concepts of Reinforcement Learning
Reinforcement Learning is a machine learning method that enables an agent to take actions in an environment and learn from the outcomes. The agent receives rewards or penalties based on its actions and uses this feedback to adjust its behavior to maximize its long-term rewards.
Reinforcement learning problems are often modeled as Markov Decision Processes (MDPs), where the agent takes actions in a state space and transitions to another state based on its state and action, while receiving rewards. The agent's goal is to find a policy, i.e., the actions to take in a given state, to maximize its long-term rewards.
#### 4.2.2 Application of MLP in Reinforcement Learning
MLPs can serve as the policy network or value function network in reinforcement learning.
**As the Policy Network:** An MLP policy network outputs the actions to be taken in a given state. An MLP policy network typically consists of multiple hidden layers, each performing a nonlinear transformation. By adjusting the number and size of hidden layers, one can control the complexity and flexibility of the policy network.
**As the Value Function Network:** An MLP value function network outputs the value of a given state, which is the long-term rewards from taking the best policy starting from that state. An MLP value function network typically consists of multiple hidden layers, each performing a nonlinear transformation. By adjusting the number and size of hidden layers, one can control the approximation capability of the value function network.
## 5. Evaluation and Deployment of MLP
### 5.1 Evaluation Metrics for MLP
#### 5.1.1 Accuracy, Recall, and F1 Score
Accuracy measures the proportion of correctly predicted samples out of the total number of samples. Recall measures the proportion of actual positive samples among the samples predicted as positive by the model. The F1 score is the harmonic mean of accuracy and recall, which takes into account both the accuracy and recall of the model.
```python
import sklearn.metrics
def evaluate_mlp(y_true, y_pred):
accuracy = sklearn.metrics.accuracy_score(y_true, y_pred)
recall = sklearn.metrics.recall_score(y_true, y_pred)
f1_score = sklearn.metrics.f1_score(y_true, y_pred)
return accuracy, recall, f1_score
```
#### 5.1.2 ROC Curve and AUC
The ROC curve (Receiver Operating Characteristic Curve) is a curve that reflects the classification ability of a model, with the horizontal axis being the False Positive Rate (FPR) and the vertical axis being the True Positive Rate (TPR). AUC (Area Under Curve) is the area under the ROC curve, which reflects the model's ability to distinguish between positive and negative samples.
```python
import sklearn.metrics
def plot_roc_curve(y_true, y_score):
fpr, tpr, thresholds = sklearn.metrics.roc_curve(y_true, y_score)
roc_auc = sklearn.metrics.auc(fpr, tpr)
plt.plot(fpr, tpr, label='ROC curve (area = %0.2f)' % roc_auc)
plt.plot([0, 1], [0, 1], 'k--')
plt.xlim([0.0, 1.0])
plt.ylim([0.0, 1.05])
plt.xlabel('False Positive Rate')
plt.ylabel('True Positive Rate')
plt.title('Receiver Operating Characteristic (ROC) Curve')
plt.legend(loc="lower right")
plt.show()
```
### 5.2 Deployment and Application of MLP
#### 5.2.1 Selection of Model Deployment Platforms
The choice of deployment platform for an MLP model depends on the scale of the model, the application scenario, ***mon deployment platforms include:
* Cloud Platforms: Cloud platforms like AWS, Azure, and Google Cloud provide hosted machine learning services, simplifying model deployment and management.
* Container Platforms: Container platforms such as Docker and Kubernetes allow models to be packaged into containers for easy deployment and running in different environments.
* Edge Devices: For low-latency and offline applications, MLP models can be deployed on edge devices like Raspberry Pi and Arduino.
#### 5.2.2 Application of MLP in Real-World Scenarios
MLP models have a wide range of applications in real-world scenarios, including:
* Image Classification: Identifying and classifying objects within images.
* Natural Language Processing: Text classification, sentiment analysis, machine translation.
* Predictive Modeling: Forecasting future events or trends, such as weather forecasting, stock market prediction.
* Recommendation Systems: Recommending personalized content based on user behavior.
* Anomaly Detection: Detecting anomalous data points or events.
## 6. Future Developments and Prospects for MLP
### 6.1 Trends in MLP Development
#### 6.1.1 Large-Scale MLP Models
As computational power continues to increase, the scale of MLP models is also expanding. In recent years, there has been a proliferation of large-scale MLP models, such as Google's Transformer and OpenAI's GPT-3. These models have billions, even trillions, of parameters and are capable of processing massive amounts of data, achieving impressive performance on various tasks.
#### 6.1.2 Enhanced Interpretability and Robustness
Interpretability has always been a challenge for MLP models. Due to the complexity of the models, it is difficult to understand how the models make decisions. In recent years, researchers have been exploring methods to improve the interpretability of MLP models, such as using visualization techniques and explainable AI technologies. Additionally, the robustness of MLP models needs to be enhanced to handle adversarial attacks and noisy data.
### 6.2 Prospects for MLP Applications in AI
#### 6.2.1 Computer Vision and Image Processing
MLP has extensive applications in the fields of computer vision and image processing. For example, MLPs can be used for image classification, object detection, and image segmentation. With the emergence of large-scale MLP models, the performance of MLPs in these tasks is expected to further improve.
#### 6.2.2 Natural Language Processing and Machine Translation
MLP also plays a significant role in natural language processing and machine translation. For example, MLPs can be used for text classification, sentiment analysis, and machine translation. As interpretability techniques improve, the application of MLPs in these tasks will become more widespread.
0
0
相关推荐
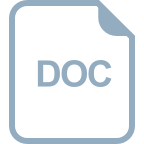
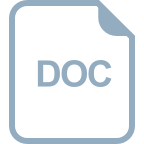
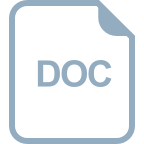
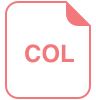
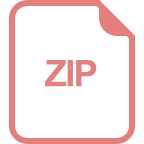
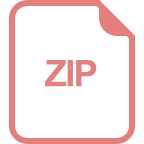
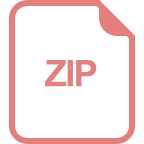
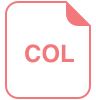
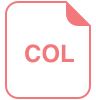
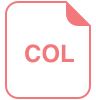