Multi-layer Perceptrons (MLP) in the Medical Field: Applications and Practice, Empowering Medical Diagnostics, Enhancing Medical Standards
发布时间: 2024-09-15 08:17:16 阅读量: 18 订阅数: 27 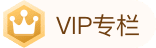
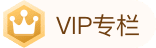
# Multilayer Perceptron (MLP) in the Medical Field: Applications and Practices, Empowering Medical Diagnostics, Enhancing Medical Quality
## 1. Fundamentals of Multilayer Perceptron (MLP)
Multilayer Perceptron (MLP) is a feedforward neural network consisting of an input layer, an output layer, and multiple hidden layers. Neurons in each hidden layer are connected to neurons in the preceding layer through weights and biases. MLP is capable of learning complex nonlinear relationships, making it a powerful tool for various tasks in the medical field.
The training process of MLP involves using the backpropagation algorithm to minimize the loss function. The loss function measures the difference between the model's predictions and the true labels. Through iterative adjustments to the weights and biases, MLP can gradually reduce the loss and improve its predictive accuracy.
The architecture and hyperparameters of MLP, such as the number of layers, the number of neurons, and the activation function, are crucial to the model's performance. Optimizing these parameters requires careful hyperparameter tuning and cross-validation to find the best configuration.
## 2. Applications of MLP in Medical Diagnostics
### 2.1 Disease Diagnosis
Multilayer Perceptron (MLP) plays a crucial role in medical diagnostics by analyzing clinical data and medical images of patients to assist doctors in making more accurate diagnoses.
### 2.1.1 Cardiovascular Disease Diagnosis
MLP has been widely applied in the diagnosis of cardiovascular diseases, such as heart attacks, heart failure, and arrhythmias. By analyzing patient data, including electrocardiograms (ECG) and echocardiograms (ECHO), MLP can identify abnormal cardiac patterns and predict the risk of diseases.
```python
import numpy as np
import pandas as pd
from sklearn.model_selection import train_test_split
from sklearn.neural_network import MLPClassifier
# Load cardiovascular disease dataset
data = pd.read_csv('heart_disease.csv')
# Split dataset into training set and test set
X_train, X_test, y_train, y_test = train_test_split(data.drop('target', axis=1), data['target'], test_size=0.2)
# Create MLP classifier
mlp = MLPClassifier(hidden_layer_sizes=(128, 64), max_iter=1000)
# Train MLP classifier
mlp.fit(X_train, y_train)
# Evaluate MLP classifier
score = mlp.score(X_test, y_test)
print('MLP classifier accuracy:', score)
```
### 2.1.2 Cancer Diagnosis
MLP can also be used for cancer diagnosis, such as breast cancer, lung cancer, and colorectal cancer. By analyzing patient pathology images, genomic data, and clinical features, MLP can help doctors distinguish between benign and malignant tumors and predict the prognosis of the disease.
### 2.2 Medical Image Analysis
MLP has a wide range of applications in medical image analysis, including medical image classification and segmentation.
### 2.2.1 Medical Image Classification
MLP can be used to classify medical images, such as X-rays, CT scans, and MRI images. By extracting features from the images, MLP can identify different anatomical structures, lesions, and abnormalities.
```python
import tensorflow as tf
from tensorflow.keras.preprocessing.image import ImageDataGenerator
from tensorflow.keras.applications import VGG16
from tensorflow.keras.layers import Dense, GlobalAveragePooling2D
from tensorflow.keras.models import Model
# Load VGG16 pretrained model
base_model = VGG16(include_top=False, weights='imagenet', input_shape=(224, 224, 3))
# Add fully connected layers and global average pooling layer
x = base_model.output
x = GlobalAveragePooling2D()(x)
x = Dense(256, activation='relu')(x)
predictions = Dense(1, activation='sigmoid')(x)
# Create model
model = Model(inputs=base_model.input, outputs=predictions)
# ***
***pile(optimizer='adam', loss='binary_crossentropy', metrics=['accuracy'])
# Load medical image dataset
train_datagen = ImageDataGenerator(rescale=1./255, shear_range=0.2, zoom_range=0.2, horizontal_flip=True)
train_generator = train_datagen.flow_from_directory('medical_images/train', target_size=(224, 224), batch_size=32, class_mode='binary')
# Train model
model.fit(train_generator, epochs=10)
```
### 2.2.2 Medical Image Segmentation
MLP can also be used for medical image segmentation, such as segmenting tumors, organs, and blood vessels. By learning the spatial relationships within the images, MLP can generate precise segmentation masks to assist doctors in diagnostics and treatment planning.
```python
import torch
from torch import nn
from torch.nn import functional
```
0
0
相关推荐
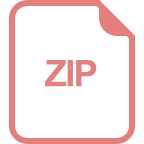
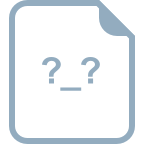
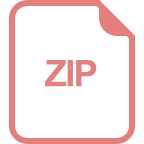





