Ensemble Learning and Multilayer Perceptrons (MLP): New Approaches for Model Fusion, Enhancing Predictive Accuracy, and Building Robust Models
发布时间: 2024-09-15 08:14:19 阅读量: 23 订阅数: 31 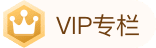
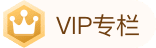
# Introduction to Ensemble Learning and Multilayer Perceptrons (MLP): New Approaches for Model Fusion to Enhance Predictive Accuracy and Build Robust Models
Ensemble learning is a machine learning technique that improves predictive performance by combining multiple models. A Multilayer Perceptron (MLP) is a type of feedforward neural network composed of multiple hidden layers.
The combination of ensemble learning and MLP leverages the strengths of both. Ensemble learning can reduce the variance of the model, while MLP enhances the model's ability to fit. By combining ensemble learning and MLP, we can build models with high predictive performance.
# Ensemble Learning Theory and Practice
### Principles and Types of Ensemble Learning
#### The Concept of Ensemble Learning
Ensemble learning is a machine learning technique that improves a model's performance by combining multiple base learners. The fundamental idea is that multiple base learners are trained on different subsets of data or different subsets of features, and then their predictions are combined to obtain the final prediction. Ensemble learning can effectively reduce the model's variance and bias, thus improving the model's generalization ability.
#### Types of Ensemble Learning
There are various types of ensemble learning algorithms, primarily divided into the following three categories:
- **Bagging (Bootstrap Aggregating):** The Bagging algorithm generates multiple different data subsets through resampling the original dataset with replacement. Then, a base learner is trained on each data subset, and the predictions of these base learners are averaged or voted on to obtain the final prediction.
- **Boosting (Adaptive Boosting):** The Boosting algorithm trains multiple base learners iteratively, with each base learner focusing on the samples that the previous base learner predicted incorrectly. In this way, the Boosting algorithm can focus on difficult samples and improve the model's predictive accuracy on these samples.
- **Stacking:** The Stacking algorithm takes the predictions of multiple base learners as input and trains a new learner (called the meta-learner) for the final prediction. The meta-learner can be any type of learner, such as linear regression, decision trees, or neural networks.
### Ensemble Learning Algorithms
#### The Bagging Algorithm
The Bagging algorithm is a simple ensemble learning algorithm, and its process is as follows:
1. Generate multiple data subsets by sampling with replacement from the original dataset.
2. Train a base learner on each data subset.
3. Average or vote on the predictions of all base learners to obtain the final prediction.
**Code Block:**
```python
from sklearn.ensemble import BaggingClassifier
# Create a Bagging classifier
bagging_classifier = BaggingClassifier(n_estimators=10)
# Train the Bagging classifier
bagging_classifier.fit(X_train, y_train)
# Predict using the Bagging classifier
y_pred = bagging_classifier.predict(X_test)
```
**Logical Analysis:**
This code block uses the `BaggingClassifier` class from the `scikit-learn` library to implement the Bagging algorithm. The `n_estimators` parameter specifies the number of base learners. The `fit` method is used to train the Bagging classifier, which divides the original dataset into multiple subsets and trains a decision tree base learner on each subset. The `predict` method is used to make predictions on the test data using the trained Bagging classifier.
#### The Boosting Algorithm
The Boosting algorithm is an iterative ensemble learning algorithm, and its process is as follows:
1. Initialize sample weights to a uniform distribution.
2. Iteratively train base learners:
- Sample the original dataset according to the current weight distribution.
- Train a base learner on the sampled data subset.
- Calculate the weight of the base learner, which is inversely proportional to the base learner's predic***
***
***bine the predictions of all base learners with weighted averages to obtain the final prediction.
**Code Block:**
```python
from sklearn.ensemble import AdaBoostClassifier
# Create an AdaBoost classifier
adaboost_classifier = AdaBoostClassifier(n_estimators=10)
# Train the AdaBoost classifier
adaboost_classifier.fit(X_train, y_train)
# Predict using the AdaBoost classifier
y_pred = adaboost_classifier.predict(X_test)
```
**Logical Analysis:**
This code block uses the `AdaBoostClassifier` class from the `scikit-learn` library to implement the AdaBoost algorithm. The `n_estimators` parameter specifies the number of base learners. The `fit` method is used to train the AdaBoost classifier, which iteratively trains decision tree base learners and updates sample we
0
0
相关推荐
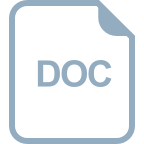
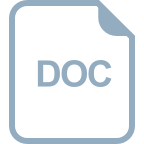
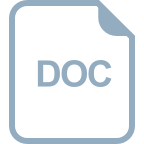
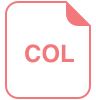
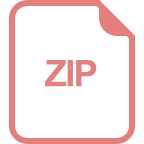
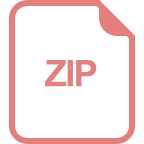
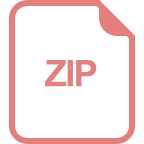
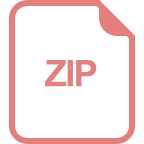