python将文本转化为特征向量
时间: 2023-08-09 12:05:00 浏览: 268
在Python中,我们可以使用`sklearn`库中的`CountVectorizer`或`TfidfVectorizer`来将文本转换为特征向量。以下是一个简单的示例:
```python
from sklearn.feature_extraction.text import CountVectorizer, TfidfVectorizer
# 创建一个文本列表
text = ["This is an example sentence.", "Another example sentence.", "I love Python!"]
# 使用CountVectorizer将文本转换为特征向量
count_vectorizer = CountVectorizer()
count_vectorizer.fit_transform(text)
# 使用TfidfVectorizer将文本转换为特征向量
tfidf_vectorizer = TfidfVectorizer()
tfidf_vectorizer.fit_transform(text)
```
在上面的代码中,我们首先创建了一个包含三个文本字符串的列表。然后,我们使用`CountVectorizer`和`TfidfVectorizer`将文本转换为特征向量。在这种情况下,我们只需调用`fit_transform()`方法即可完成转换。特征向量可以使用`toarray()`方法转换为Numpy数组。
相关问题
jupyter 使用TF-IDF特征提取方法将文本转化为特征向量
好的,针对您的问题,我可以给您一些思路和建议。TF-IDF 是一种常用的文本特征提取方法,可以将文本转化为特征向量,用于机器学习算法的训练。下面简单介绍一下如何使用 TF-IDF 特征提取方法将文本转化为特征向量。
1. 准备数据集:将需要进行情感分析的文本进行分词处理,并将其和对应的情感标签构建成数据集。
2. 数据预处理:对数据进行预处理,如去除停用词、分词、词性标注等。
3. 特征提取:使用 TF-IDF 特征提取方法将文本转化为特征向量。可以使用 Python 中的 sklearn.feature_extraction.text.TfidfVectorizer 类来实现。具体代码示例如下:
``` python
from sklearn.feature_extraction.text import TfidfVectorizer
# 构建 TF-IDF 特征提取器
tfidf_vec = TfidfVectorizer()
# 训练 TF-IDF 特征提取器,并转化为稀疏矩阵
tfidf_matrix = tfidf_vec.fit_transform(corpus)
# 将稀疏矩阵转化为数组
tfidf_array = tfidf_matrix.toarray()
```
其中,corpus 表示分词后的文本列表,tfidf_array 表示转化后的特征向量数组。
4. 数据划分:将数据集划分为训练集和测试集。
5. 训练模型:使用机器学习算法对训练集进行训练,并得到模型。
6. 模型评估:使用测试集对训练好的模型进行评估,计算准确率、召回率、F1 值等指标。
7. 应用模型:使用模型对新的文本进行情感分析,得到相应的情感标签。
需要注意的是,TF-IDF 特征提取方法可以有效地减少高频词和低频词对模型的干扰,提高特征的区分度。但是,在使用过程中也需要注意调整词频阈值和 IDF 阈值等参数。
希望这些信息对您有所帮助。如果您有其他问题,欢迎继续提问。
python将文本字符串转化为向量
在Python中将文本字符串转换为向量的一种常见方法是使用词袋模型。词袋模型将文本表示为词语出现频率的向量。具体步骤如下:
1. 分词:将文本字符串分解为单词或短语。
2. 构建词汇表:将所有单词或短语收集到一个词汇表中。
3. 编码文本:使用词汇表将文本转换为向量,其中每个元素表示对应单词或短语在文本中出现的次数。
下面是一个简单的Python代码示例,演示将文本字符串转换为向量:
``` python
# 导入必要的库
from sklearn.feature_extraction.text import CountVectorizer
# 定义文本字符串
text = ["This is an example sentence.",
"Another example sentence.",
"I love Python!"]
# 创建CountVectorizer对象
vectorizer = CountVectorizer()
# 将文本字符串转换为向量
vector = vectorizer.fit_transform(text)
# 打印向量
print(vector.toarray())
```
输出:
```
[[0 1 1 1 0 0 1 0]
[0 1 0 1 0 1 1 0]
[1 0 0 0 1 0 0 1]]
```
这里的每行表示一个文本字符串,每列表示词汇表中的一个单词或短语。每个元素表示对应单词或短语在文本中出现的次数。
阅读全文
相关推荐
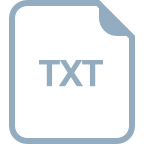

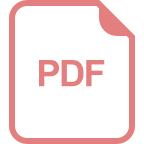
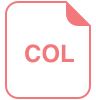









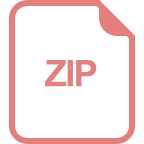
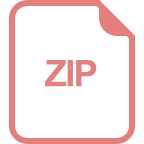