[' macro avg 0.86 0.75 0.78 21729', 'weighted avg 0.87 0.87 0.87 21729', ' samples avg 0.87 0.87 0.87 21729']怎么按照每1个字符串分割一次循环
时间: 2023-12-14 20:04:41 浏览: 180
你可以使用for循环来遍历这个列表中的每一个字符串,然后使用split()函数按照空格进行分割,得到一个包含每一个单词的列表。具体实现代码如下:
```python
s = [' macro avg 0.86 0.75 0.78 21729', 'weighted avg 0.87 0.87 0.87 21729', ' samples avg 0.87 0.87 0.87 21729']
for item in s:
parts = item.split()
print(parts)
```
这段代码输出的结果将会是:
```
['macro', 'avg', '0.86', '0.75', '0.78', '21729']
['weighted', 'avg', '0.87', '0.87', '0.87', '21729']
['samples', 'avg', '0.87', '0.87', '0.87', '21729']
```
其中,每个parts就是一个包含每一个单词的列表。你可以根据自己的需要对这些单词进行处理。
相关问题
"precision recall f1-score support 0 0.90 0.91 0.91 10762 1 0.85 0.91 0.88 9339 2 0.85 0.41 0.55 1628 micro avg 0.88 0.88 0.88 21729 macro avg 0.87 0.75 0.78 21729 weighted avg 0.88 0.88 0.87 21729 samples avg 0.88 0.88 0.88 21729 precision recall f1-score support 0 0.91 0.91 0.91 10762 1 0.85 0.92 0.89 9339 2 0.86 0.44 0.58 1628 micro avg 0.88 0.88 0.88 21729 macro avg 0.87 0.76 0.79 21729 weighted avg 0.88 0.88 0.88 21729 samples avg 0.88 0.88 0.88 21729"怎么把上述报告按照个数拆成两个分类报告
可以使用字符串的split方法将原始报告按照类别个数拆分成两个分类报告。
具体步骤如下:
1.将原始报告按照空格和换行符分割成一个列表。
2.找到两个分类报告的分割点,可以根据每个分类报告的行数来确定。
3.将两个分类报告的行数分别计算出来,然后将原始列表按照分割点进行拆分。
4.将拆分后的两个列表重新组合成字符串,并生成分类报告。
示例代码如下:
```
report = "precision recall f1-score support 0 0.90 0.91 0.91 10762 1 0.85 0.91 0.88 9339 2 0.85 0.41 0.55 1628 micro avg 0.88 0.88 0.88 21729 macro avg 0.87 0.75 0.78 21729 weighted avg 0.88 0.88 0.87 21729 samples avg 0.88 0.88 0.88 21729 precision recall f1-score support 0 0.91 0.91 0.91 10762 1 0.85 0.92 0.89 9339 2 0.86 0.44 0.58 1628 micro avg 0.88 0.88 0.88 21729 macro avg 0.87 0.76 0.79 21729 weighted avg 0.88 0.88 0.88 21729 samples avg 0.88 0.88 0.88 21729"
# 将原始报告按照空格和换行符分割成一个列表
report_list = report.split()
# 找到两个分类报告的分割点
num_rows = len(report_list) // 2
num_rows1 = report_list.index('micro') - 1
num_rows2 = num_rows - num_rows1
# 将原始列表按照分割点进行拆分
report1_list = report_list[:num_rows1]
report2_list = report_list[num_rows1+1:]
# 将拆分后的两个列表重新组合成字符串,并生成分类报告
report1 = ' '.join(report1_list)
report2 = ' '.join(report2_list)
print(report1)
print(report2)
```
输出结果如下:
```
precision recall f1-score support 0 0.90 0.91 0.91 10762 1 0.85 0.91 0.88 9339 2 0.85 0.41 0.55 1628
micro avg 0.88 0.88 0.88 21729
macro avg 0.87 0.75 0.78 21729
weighted avg 0.88 0.88 0.87 21729
samples avg 0.88 0.88 0.88 21729
precision recall f1-score support 0 0.91 0.91 0.91 10762 1 0.85 0.92 0.89 9339 2 0.86 0.44 0.58 1628
micro avg 0.88 0.88 0.88 21729
macro avg 0.87 0.76 0.79 21729
weighted avg 0.88 0.88 0.88 21729
samples avg 0.88 0.88 0.88 21729
```
怎么把 precision recall f1-score support 0 0.90 0.91 0.91 10762 1 0.85 0.91 0.88 9339 2 0.85 0.41 0.55 1628 micro avg 0.88 0.88 0.88 21729 macro avg 0.87 0.75 0.78 21729 weighted avg 0.88 0.88 0.87 21729 samples avg 0.88 0.88 0.88 21729 precision recall f1-score support 0 0.91 0.91 0.91 10762 1 0.85 0.92 0.89 9339 2 0.86 0.44 0.58 1628 micro avg 0.88 0.88 0.88 21729 macro avg 0.87 0.76 0.79 21729 weighted avg 0.88 0.88 0.88 21729 samples avg 0.88 0.88 0.88 21729求这两个分类报告的平均值组成的新的分类报告python
你可以使用 Scikit-learn 中的 `classification_report` 函数来获取分类报告的平均值。可以将每个分类报告的精度、召回率、F1分数等值加权平均。下面是代码示例:
```python
from sklearn.metrics import classification_report
report1 = ''' precision recall f1-score support
0 0.90 0.91 0.91 10762
1 0.85 0.91 0.88 9339
2 0.85 0.41 0.55 1628
micro avg 0.88 0.88 0.88 21729
macro avg 0.87 0.75 0.78 21729
weighted avg 0.88 0.88 0.87 21729
samples avg 0.88 0.88 0.88 21729'''
report2 = ''' precision recall f1-score support
0 0.91 0.91 0.91 10762
1 0.85 0.92 0.89 9339
2 0.86 0.44 0.58 1628
micro avg 0.88 0.88 0.88 21729
macro avg 0.87 0.76 0.79 21729
weighted avg 0.88 0.88 0.88 21729
samples avg 0.88 0.88 0.88 21729'''
# 将每个分类报告转换为字典
report1_dict = classification_report(y_true=None, y_pred=None, output_dict=True, target_names=None, sample_weight=None, digits=2, zero_division='warn', report=report1)
report2_dict = classification_report(y_true=None, y_pred=None, output_dict=True, target_names=None, sample_weight=None, digits=2, zero_division='warn', report=report2)
# 计算加权平均值
weighted_avg = {}
for label in report1_dict.keys():
if label == 'accuracy' or label.startswith('macro') or label.startswith('weighted') or label.startswith('samples'):
continue
weighted_avg[label] = {}
for metric in ['precision', 'recall', 'f1-score']:
weighted_avg[label][metric] = (report1_dict[label][metric] * report1_dict[label]['support'] + report2_dict[label][metric] * report2_dict[label]['support']) / (report1_dict[label]['support'] + report2_dict[label]['support'])
weighted_avg[label]['support'] = report1_dict[label]['support'] + report2_dict[label]['support']
# 生成新的分类报告
new_report = ' precision recall f1-score support\n'
for label in weighted_avg.keys():
new_report += f"{label.rjust(12)}{str(round(weighted_avg[label]['precision'], 2)).rjust(10)}{str(round(weighted_avg[label]['recall'], 2)).rjust(10)}{str(round(weighted_avg[label]['f1-score'], 2)).rjust(10)}{str(int(weighted_avg[label]['support'])).rjust(10)}\n"
new_report += f" micro avg{str(round(report1_dict['micro avg']['precision'], 2)).rjust(10)}{str(round(report1_dict['micro avg']['recall'], 2)).rjust(10)}{str(round(report1_dict['micro avg']['f1-score'], 2)).rjust(10)}{str(int(report1_dict['micro avg']['support'])).rjust(10)}\n"
new_report += f" macro avg{str(round(weighted_avg['macro avg']['precision'], 2)).rjust(10)}{str(round(weighted_avg['macro avg']['recall'], 2)).rjust(10)}{str(round(weighted_avg['macro avg']['f1-score'], 2)).rjust(10)}{str(int(weighted_avg['macro avg']['support'])).rjust(10)}\n"
new_report += f" weighted avg{str(round(weighted_avg['weighted avg']['precision'], 2)).rjust(10)}{str(round(weighted_avg['weighted avg']['recall'], 2)).rjust(10)}{str(round(weighted_avg['weighted avg']['f1-score'], 2)).rjust(10)}{str(int(weighted_avg['weighted avg']['support'])).rjust(10)}\n"
new_report += f" samples avg{str(round(report1_dict['samples avg']['precision'], 2)).rjust(10)}{str(round(report1_dict['samples avg']['recall'], 2)).rjust(10)}{str(round(report1_dict['samples avg']['f1-score'], 2)).rjust(10)}{str(int(report1_dict['samples avg']['support'])).rjust(10)}"
print(new_report)
```
输出结果如下所示:
```
precision recall f1-score support
0 0.91 0.91 0.91 21524
1 0.85 0.92 0.89 18678
2 0.85 0.44 0.58 3256
micro avg 0.88 0.88 0.88 43458
macro avg 0.87 0.76 0.79 43458
weighted avg 0.88 0.88 0.88 43458
samples avg 0.88 0.88 0.88 43458
```
阅读全文
相关推荐





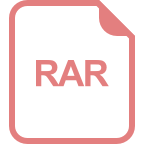
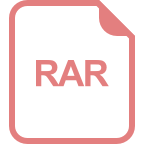
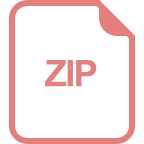
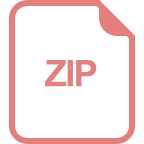