旋转拉伸矩阵的matlab代码
时间: 2023-06-13 16:02:32 浏览: 65
旋转拉伸矩阵可以表示为由旋转和拉伸构成的线性变换矩阵,可以使用MATLAB代码实现。以下是一个示例代码:
```matlab
% 旋转角度(弧度)
theta = pi/4;
% 拉伸因子
s1 = 2;
s2 = 0.5;
% 构造旋转矩阵
R = [cos(theta) -sin(theta) 0; sin(theta) cos(theta) 0; 0 0 1];
% 构造拉伸矩阵
S = [s1 0 0; 0 s2 0; 0 0 1];
% 旋转拉伸矩阵
M = R*S;
% 测试向量
v = [1; 1; 1];
% 变换后的向量
v_transformed = M*v;
```
在示例代码中,我们首先定义旋转角度和拉伸因子,然后使用MATLAB内置的cos和sin函数计算旋转矩阵,并使用拉伸因子构造拉伸矩阵。最后将旋转矩阵和拉伸矩阵相乘,得到旋转拉伸矩阵。我们使用一个测试向量来进行变换,并输出变换后的向量。
相关问题
matlab绘制绕中心旋转任意角度的椭圆的代码
以下是 Matlab 绘制绕中心旋转任意角度的椭圆的代码:
```matlab
% 定义椭圆的中心和半轴长度
x0 = 0;
y0 = 0;
a = 2;
b = 1;
% 定义旋转角度
theta = pi/4;
% 生成椭圆上的点
t = linspace(0, 2*pi);
x = x0 + a*cos(t);
y = y0 + b*sin(t);
% 构造旋转矩阵
R = [cos(theta) -sin(theta); sin(theta) cos(theta)];
% 将椭圆上的点绕中心旋转
p = R*[x;y];
% 绘制旋转后的椭圆
plot(p(1,:), p(2,:));
axis equal;
```
其中,`x0` 和 `y0` 分别为椭圆的中心坐标,`a` 和 `b` 分别为椭圆的长半轴和短半轴长度,`theta` 为旋转角度。代码中,我们先生成椭圆上的点,再构造一个旋转矩阵,最后将椭圆上的点绕中心旋转,并绘制出旋转后的椭圆。需要注意的是,绘制时应该将坐标轴设置为等比例缩放,以保证椭圆不会被拉伸或压缩。
用MATLAB写一段代码,将一张倾斜拍摄的图像拉伸成正常视角的比例
可以使用MATLAB中的几何变换函数来将一张倾斜拍摄的图像拉伸成正常视角的比例。具体的步骤如下:
1. 读取倾斜拍摄的图像,并确定需要拉伸到的目标图像大小。
2. 根据倾斜拍摄的图像中的特征点,估计出图像的旋转角度和变换矩阵。
3. 使用MATLAB中的仿射变换函数affine2d,将图像进行旋转和变换,以实现倾斜的矫正。
4. 将变换后的图像进行缩放,使其达到目标大小。
下面是一段示例代码,用于将一张倾斜的图像进行矫正:
```matlab
% 读取倾斜的图像
im = imread('skewed_image.jpg');
% 确定目标图像大小
targetSize = [600, 800];
% 通过检测特征点估计旋转角度和变换矩阵
points = detectSURFFeatures(rgb2gray(im));
[features, valid_points] = extractFeatures(rgb2gray(im), points);
indexPairs = matchFeatures(features1,features2);
matchedPoints1 = valid_points(indexPairs(:,1));
matchedPoints2 = valid_points(indexPairs(:,2));
[tform, inlierPoints1, inlierPoints2] = ...
estimateGeometricTransform(matchedPoints1, matchedPoints2, 'affine');
% 对图像进行仿射变换,使其旋转并矫正倾斜
im2 = imwarp(im, tform);
% 缩放图像至目标大小
im3 = imresize(im2, targetSize);
% 显示矫正后的图像
imshow(im3);
```
这段代码通过检测图像中的特征点,并使用SURF算法进行特征点匹配,从而估计出了图像的旋转角度和变换矩阵。然后使用MATLAB中的仿射变换函数affine2d对图像进行旋转和变换,以实现倾斜的矫正。最后将变换后的图像进行缩放,使其达到目标大小。
相关推荐
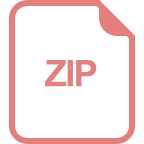
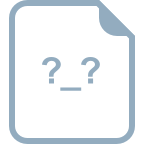







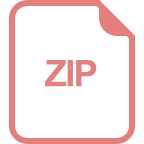
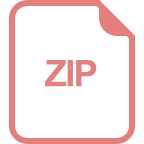
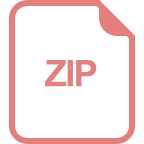
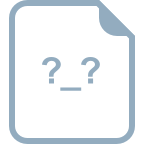