复杂的cart分类树和回归树的构建代码python
时间: 2024-02-03 15:12:31 浏览: 67
下面是一个简单的示例代码,展示了如何使用 sklearn 构建 CART 分类树和回归树:
分类树:
```python
from sklearn.tree import DecisionTreeClassifier
from sklearn.datasets import load_iris
from sklearn.model_selection import train_test_split
# 加载数据集
iris = load_iris()
X_train, X_test, y_train, y_test = train_test_split(iris.data, iris.target, test_size=0.3, random_state=0)
# 构建决策树分类器
clf = DecisionTreeClassifier(criterion='gini', max_depth=3, random_state=0)
clf.fit(X_train, y_train)
# 在测试集上进行预测
y_pred = clf.predict(X_test)
# 计算分类准确率
accuracy = clf.score(X_test, y_test)
print("Classification accuracy:", accuracy)
```
回归树:
```python
from sklearn.tree import DecisionTreeRegressor
from sklearn.datasets import load_boston
from sklearn.model_selection import train_test_split
# 加载数据集
boston = load_boston()
X_train, X_test, y_train, y_test = train_test_split(boston.data, boston.target, test_size=0.3, random_state=0)
# 构建决策树回归器
regressor = DecisionTreeRegressor(criterion='mse', max_depth=3, random_state=0)
regressor.fit(X_train, y_train)
# 在测试集上进行预测
y_pred = regressor.predict(X_test)
# 计算回归性能指标
from sklearn.metrics import mean_squared_error, r2_score
mse = mean_squared_error(y_test, y_pred)
r2 = r2_score(y_test, y_pred)
print("Mean squared error:", mse)
print("R2 score:", r2)
```
阅读全文
相关推荐
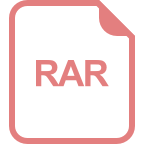
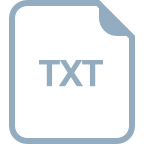
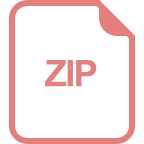















