producer.setCompressMsgBodyOverHowmuch(Integer.MAX_VALUE);
时间: 2024-05-22 14:15:37 浏览: 54
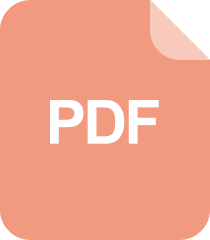
L20.pdfmit 6.004 计算机组成原理课件
This line of code sets the maximum size of the message body that can be compressed by the producer to the maximum possible integer value. This means that the producer will attempt to compress all message bodies regardless of their size. The purpose of compressing message bodies is to reduce the amount of data that needs to be transmitted over the network, which can improve performance and reduce costs. However, compressing small message bodies may not be worthwhile if the overhead of compression outweighs the benefits.
阅读全文
相关推荐
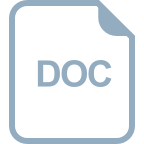
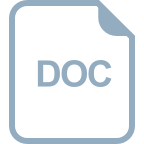
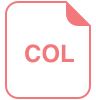
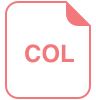
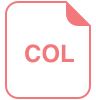
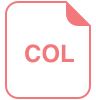
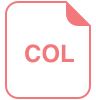
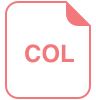

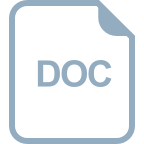
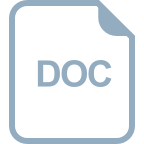
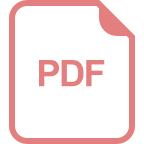
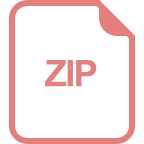
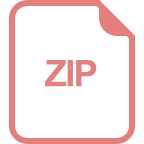
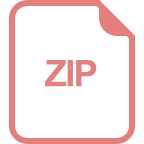
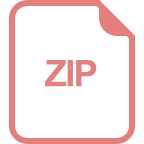