np.flatten举例
时间: 2023-10-30 20:02:57 浏览: 40
Sure! np.flatten() is a NumPy function which returns a 1D array by flattening a given array. Here's an example:
```
import numpy as np
arr = np.array([[1, 2],
[3, 4]])
flattened_arr = arr.flatten()
print(flattened_arr) # Output: [1 2 3 4]
```
相关问题
np.flatten
np.flatten是numpy库中的一个函数,它用于将多维数组展平成一维数组。在使用np.flatten函数时,可以省略参数或者指定参数'C'或'F',其中'C'表示以C语言的行优先顺序展平数组,'F'表示以Fortran语言的列优先顺序展平数组。对于二维数组来说,默认情况下,np.flatten是按行优先顺序展平数组的,即按照行的顺序将数组的元素依次放入一维数组中。 <span class="em">1</span><span class="em">2</span><span class="em">3</span>
#### 引用[.reference_title]
- *1* *2* [torch.flatten、np.flatten 详解](https://blog.csdn.net/qq_28949847/article/details/128568723)[target="_blank" data-report-click={"spm":"1018.2226.3001.9630","extra":{"utm_source":"vip_chatgpt_common_search_pc_result","utm_medium":"distribute.pc_search_result.none-task-cask-2~all~insert_cask~default-1-null.142^v93^chatsearchT3_2"}}] [.reference_item style="max-width: 50%"]
- *3* [numpy数组](https://download.csdn.net/download/weixin_38674883/13741656)[target="_blank" data-report-click={"spm":"1018.2226.3001.9630","extra":{"utm_source":"vip_chatgpt_common_search_pc_result","utm_medium":"distribute.pc_search_result.none-task-cask-2~all~insert_cask~default-1-null.142^v93^chatsearchT3_2"}}] [.reference_item style="max-width: 50%"]
[ .reference_list ]
layers.flatten举例
layers.flatten是一个常用的神经网络层,它用于将输入数据展平为一维向量。举个例子,假设我们有一个输入张量的形状为(2, 3, 4),即有2个样本,每个样本有3行4列的数据。使用layers.flatten层后,输入张量将被展平为一维向量,形状为(2, 12)。
相关问题:
1. layers.flatten层的作用是什么?
2. 如何在Keras中使用layers.flatten层?
3. layers.flatten层对于图像数据有什么应用?
相关推荐
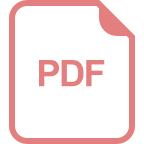
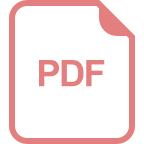












