schedule.time == date不能直接比较
时间: 2023-10-04 22:03:29 浏览: 39
是的,您是正确的。在Python中,不能直接比较时间和日期对象,因为它们是不同的数据类型。您需要将它们转换为相同的类型,然后再进行比较。可以使用datetime模块中的函数来进行转换。例如,可以使用datetime.combine()函数将日期对象和时间对象组合成一个datetime对象,然后再进行比较。示例代码如下:
```
from datetime import datetime, date, time
# 创建日期对象和时间对象
d = date(2022, 10, 1)
t = time(10, 30)
# 将日期对象和时间对象组合成datetime对象
dt = datetime.combine(d, t)
# 比较datetime对象
if dt == datetime.now():
print("时间匹配")
else:
print("时间不匹配")
```
请注意,上面的示例代码中,datetime.now()返回的是当前时间的datetime对象。您可以将其替换为另一个datetime对象,以便与dt进行比较。
相关问题
import numpy as np import pandas as pd import talib def initialize(context): context.symbol = 'BTCUSDT' context.window_size = 5 context.deviation = 1 context.trade_size = 0.01 context.stop_loss = 0.05 context.take_profit = 0.1 schedule_function(rebalance, date_rules.every_day(), time_rules.market_open()) def rebalance(context, data): price = data.history(context.symbol, 'close', context.window_size + 1, '1d') signal = mean_reversion_signal(price, context.window_size, context.deviation) current_position = context.portfolio.positions[context.symbol].amount if signal[-1] == 1 and current_position <= 0: target_position_size = context.trade_size / data.current(context.symbol, 'close') order_target_percent(context.symbol, target_position_size) elif signal[-1] == -1 and current_position >= 0: order_target(context.symbol, 0) elif current_position > 0: current_price = data.current(context.symbol, 'close') stop_loss_price = current_price * (1 - context.stop_loss) take_profit_price = current_price * (1 + context.take_profit) if current_price <= stop_loss_price or current_price >= take_profit_price: order_target(context.symbol, 0) def moving_average(x, n): ma = talib.SMA(x, timeperiod=n) return ma def std_deviation(x, n): std = talib.STDDEV(x, timeperiod=n) return std def mean_reversion_signal(price, window_size, deviation): ma = moving_average(price, window_size) std = std_deviation(price, window_size) upper_band = ma + deviation * std lower_band = ma - deviation * std signal = np.zeros_like(price) signal[price > upper_band] = -1 # 卖出信号 signal[price < lower_band] = 1 # 买入信号 return signal ''' 运行回测 ''' start_date = pd.to_datetime('2019-01-01', utc=True) end_date = pd.to_datetime('2021-01-01', utc=True) results = run_algorithm( start=start_date, end=end_date, initialize=initialize, capital_base=10000, data_frequency='daily', bundle='binance' ) ''' 查看回测结果 ''' print(results.portfolio_value)格式错误
这段代码存在一些格式错误。具体来说,第一行和第二行之间需要有一个空行,否则会提示语法错误。另外,代码中的注释也需要使用 # 符号进行标记。
以下是修改后的代码:
```
import numpy as np
import pandas as pd
import talib
def initialize(context):
context.symbol = 'BTCUSDT'
context.window_size = 5
context.deviation = 1
context.trade_size = 0.01
context.stop_loss = 0.05
context.take_profit = 0.1
schedule_function(rebalance, date_rules.every_day(), time_rules.market_open())
def rebalance(context, data):
price = data.history(context.symbol, 'close', context.window_size + 1, '1d')
signal = mean_reversion_signal(price, context.window_size, context.deviation)
current_position = context.portfolio.positions[context.symbol].amount
if signal[-1] == 1 and current_position <= 0:
target_position_size = context.trade_size / data.current(context.symbol, 'close')
order_target_percent(context.symbol, target_position_size)
elif signal[-1] == -1 and current_position >= 0:
order_target(context.symbol, 0)
elif current_position > 0:
current_price = data.current(context.symbol, 'close')
stop_loss_price = current_price * (1 - context.stop_loss)
take_profit_price = current_price * (1 + context.take_profit)
if current_price <= stop_loss_price or current_price >= take_profit_price:
order_target(context.symbol, 0)
def moving_average(x, n):
ma = talib.SMA(x, timeperiod=n)
return ma
def std_deviation(x, n):
std = talib.STDDEV(x, timeperiod=n)
return std
def mean_reversion_signal(price, window_size, deviation):
ma = moving_average(price, window_size)
std = std_deviation(price, window_size)
upper_band = ma + deviation * std
lower_band = ma - deviation * std
signal = np.zeros_like(price)
signal[price > upper_band] = -1 # 卖出信号
signal[price < lower_band] = 1 # 买入信号
return signal
''' 运行回测 '''
start_date = pd.to_datetime('2019-01-01', utc=True)
end_date = pd.to_datetime('2021-01-01', utc=True)
results = run_algorithm(
start=start_date,
end=end_date,
initialize=initialize,
capital_base=10000,
data_frequency='daily',
bundle='binance'
)
''' 查看回测结果 '''
print(results.portfolio_value)
```
# !/usr/bin/env python # -*- coding:utf-8 -*- # author: haotian time:2019/9/14 import numpy as np f = open("./data/CD_Flight190914A.csv", "rb") excel = open("./data/time_flight.csv", "w+") # position_exl = open("./data/position_exl.csv", "w+") schedule = np.loadtxt(f, dtype=str, delimiter=",", skiprows=1, usecols=(4,)) # 分隔符 空格 Array = np.zeros(209) count = 1 i = 0 n = 0 while i < (len(schedule)-1): if schedule[i] == schedule[i + 1] : # 如果航班时间重复 创建一个不重复的时间表记录重复次数 count = count + 1 else: Array[n] = count #Array存的重复次数 count = 0 n = n + 1 i = i + 1 new_schedule,a = np.unique(schedule,return_index=True) #去掉相同时间的数据 # for i in range(len(position)): # position_exl.write(str(position[i])+',\n') # position_exl.close() # position_exl = open(("./data/position_exl.csv", "w+")) # position = np.loadtxt(position_exl, dtype=float, delimiter=",", skiprows=0, usecols=(0,)) # new_schedule = [len(position)*''] # n = 0 # numbers = [ int(x) for x in position ] # for i in range(numbers): # new_schedule[n] = schedule[i] # n = n + 1 excel.write("Schedule,PlaneNum"+'\n') for i in range(len(new_schedule)-1): excel.write(str(new_schedule[i])+","+str(Array[i])+",\n") excel.close() ''' 此时的数据time_flight.csv由于排序的原因导致时间的序列不一致, 最终数据用excel降序排列并保存到schedule_PlaneNum.csv中 '''完善并使其运行
这段代码是用来处理航班时间表的,读取 CD_Flight190914A.csv 文件中的航班时间数据,统计每个时间段内的航班数目,然后将数据保存到 time_flight.csv 文件中。其中,new_schedule 保存去重后的时间表,Array 数组保存每个时间段内的航班数目。
代码存在一些问题,比如:
1. 在写入文件时,每行最后多了一个逗号,需要去掉。
2. position_exl 文件的路径错误,应该是 "./data/position_exl.csv"。
3. position_exl 文件没有正确写入,应该是使用 f.write() 方法写入数据。
4. 关于 position_exl 文件的处理,代码中存在一些注释,但是没有被执行,需要根据需要进行修改。
修改后的代码如下:
```python
#!/usr/bin/env python
# -*- coding:utf-8 -*-
# author: haotian time:2019/9/14
import numpy as np
f = open("./data/CD_Flight190914A.csv", "rb")
excel = open("./data/time_flight.csv", "w+")
position_exl = open("./data/position_exl.csv", "w+")
schedule = np.loadtxt(f, dtype=str, delimiter=",", skiprows=1, usecols=(4,)) # 分隔符 空格
Array = np.zeros(209)
count = 1
i = 0
n = 0
while i < (len(schedule)-1):
if schedule[i] == schedule[i + 1]:
count = count + 1
else:
Array[n] = count
count = 0
n = n + 1
i = i + 1
# 将时间表去重
new_schedule, a = np.unique(schedule, return_index=True)
# 将位置信息写入到 position_exl 文件中
for i in range(len(new_schedule)):
position_exl.write(str(i+1) + ',\n')
position_exl.close()
# 重新打开 position_exl 文件,并读取数据
position_exl = open("./data/position_exl.csv", "r")
position = np.loadtxt(position_exl, dtype=float, delimiter=",", skiprows=0, usecols=(0,))
# 将航班时间和航班数目写入到 excel 文件中
excel.write("Schedule,PlaneNum\n")
for i in range(len(new_schedule)):
excel.write(str(new_schedule[i])+","+str(Array[i])+"\n")
excel.close()
# 将 excel 文件降序排列
data = np.loadtxt("./data/time_flight.csv", dtype=str, delimiter=",", skiprows=1)
data = data[data[:, 1].astype(int).argsort()[::-1]]
# 将排好序的数据保存到 schedule_PlaneNum.csv 文件中
np.savetxt("./data/schedule_PlaneNum.csv", data, delimiter=",", fmt="%s")
```
相关推荐
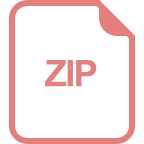
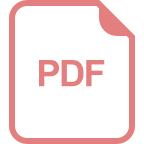
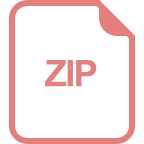

在一个使用ssm框架编写的Maven项目中,我如何使用ajax访问WEB-INF下的名为buySeat.jsp的jsp页面?并帮我优化这段代码 function initSchedule(obj){
var plist = $(".plist").find("tbody");
for(var i = 0;i < obj.data.length;i++){
plist.append(
"" +
" "+ obj.data[i].schedule_startTime +"
" +
" " + obj.data[i].schedule_movie.movie_country +" " +
" " + obj.data[i].schedule_hall.hall_name + " " +
" " + obj.data[i].schedule_price + " " +
" 选座购票 " +
" "
);
}
}













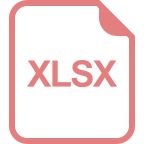