gui界面遗传算法求解流水车间调度问题设计 python
时间: 2023-08-07 13:35:04 浏览: 107
遗传算法是一种常用的优化算法,可以用于求解流水车间调度问题。下面是一个使用 Python 的 Tkinter 模块设计的 GUI 界面,可以通过遗传算法求解流水车间调度问题:
```python
import tkinter as tk
import random
import numpy as np
def init_population(pop_size, job_count, machine_count):
# 初始化种群
population = []
for i in range(pop_size):
chromosome = np.zeros((job_count, machine_count), dtype=int)
for j in range(job_count):
chromosome[j] = np.random.permutation(machine_count)
population.append(chromosome)
return population
def fitness(chromosome, processing_times):
# 计算适应度
makespan = np.zeros(len(chromosome), dtype=int)
for i in range(len(chromosome)):
job_times = np.zeros(len(chromosome[i]), dtype=int)
for j in range(len(chromosome[i])):
machine = chromosome[i][j]
job_times[machine] += processing_times[i][machine]
if job_times[machine] > makespan[i]:
makespan[i] = job_times[machine]
return 1.0 / makespan
def selection(population, fitness_values):
# 选择操作
fitness_sum = sum(fitness_values)
probabilities = [fitness_values[i] / fitness_sum for i in range(len(fitness_values))]
selected_indices = np.random.choice(len(population), size=len(population), p=probabilities)
selected_population = [population[i] for i in selected_indices]
return selected_population
def crossover(parent1, parent2):
# 交叉操作
child1 = parent1.copy()
child2 = parent2.copy()
crossover_point = int(len(parent1) / 2)
child1[crossover_point:] = parent2[crossover_point:]
child2[crossover_point:] = parent1[crossover_point:]
return child1, child2
def mutation(chromosome, mutation_prob):
# 变异操作
for i in range(len(chromosome)):
for j in range(len(chromosome[i])):
if random.random() < mutation_prob:
chromosome[i][j] = np.random.randint(len(chromosome[i]))
return chromosome
def genetic_algorithm(pop_size, job_count, machine_count, processing_times, max_generations):
# 遗传算法求解流水车间调度问题
population = init_population(pop_size, job_count, machine_count)
for generation in range(max_generations):
fitness_values = [fitness(chromosome, processing_times) for chromosome in population]
selected_population = selection(population, fitness_values)
new_population = []
for i in range(int(pop_size / 2)):
parent1 = random.choice(selected_population)
parent2 = random.choice(selected_population)
child1, child2 = crossover(parent1, parent2)
child1 = mutation(child1, 0.1)
child2 = mutation(child2, 0.1)
new_population.append(child1)
new_population.append(child2)
population = new_population
best_chromosome = max(population, key=lambda c: fitness(c, processing_times))
return best_chromosome
class Application(tk.Frame):
def __init__(self, master=None):
super().__init__(master)
self.master = master
self.pack()
self.create_widgets()
def create_widgets(self):
# 创建控件
self.job_count_label = tk.Label(self, text="作业数量")
self.job_count_label.grid(row=0, column=0)
self.job_count_entry = tk.Entry(self)
self.job_count_entry.grid(row=0, column=1)
self.machine_count_label = tk.Label(self, text="机器数量")
self.machine_count_label.grid(row=1, column=0)
self.machine_count_entry = tk.Entry(self)
self.machine_count_entry.grid(row=1, column=1)
self.processing_times_label = tk.Label(self, text="加工时间矩阵")
self.processing_times_label.grid(row=2, column=0)
self.processing_times_text = tk.Text(self, height=10, width=30)
self.processing_times_text.grid(row=2, column=1)
self.run_button = tk.Button(self, text="运行", command=self.run)
self.run_button.grid(row=3, column=0)
self.result_label = tk.Label(self, text="结果")
self.result_label.grid(row=3, column=1)
self.quit_button = tk.Button(self, text="退出", command=self.master.destroy)
self.quit_button.grid(row=4, column=1)
def run(self):
# 运行遗传算法求解流水车间调度问题
job_count = int(self.job_count_entry.get())
machine_count = int(self.machine_count_entry.get())
processing_times = [list(map(int, row.split())) for row in self.processing_times_text.get("1.0", "end").split("\n") if row.strip()]
best_chromosome = genetic_algorithm(50, job_count, machine_count, processing_times, 100)
self.result_label.configure(text=str(best_chromosome))
# 创建主窗口
root = tk.Tk()
# 设置窗口标题
root.title("流水车间调度问题求解")
# 创建应用程序
app = Application(master=root)
# 进入消息循环
app.mainloop()
```
这个程序创建了一个 GUI 界面,包含作业数量、机器数量和加工时间矩阵三个输入框,以及一个运行按钮和一个结果标签。你可以输入作业数量、机器数量和加工时间矩阵,然后点击运行按钮,程序将使用遗传算法求解流水车间调度问题,并在结果标签中显示最优解的染色体。
阅读全文
相关推荐
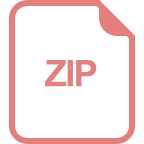
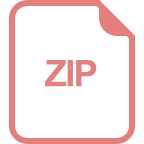
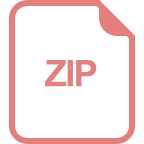
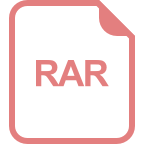
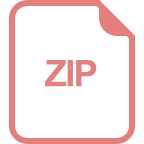
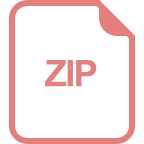
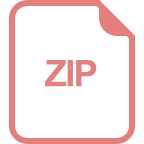
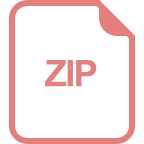
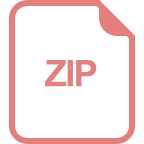
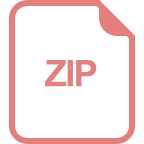
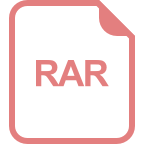
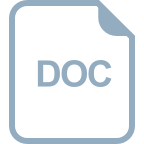
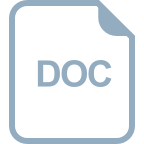
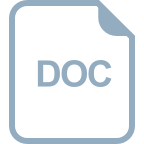
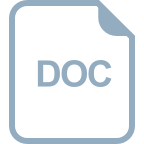
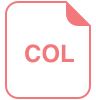
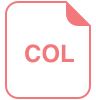
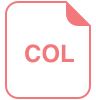