cnn代码,使用pytorch,将45x235的低分辨率输入映射为150x675的高分辨率输出,训练数据为700个,测试数据为300个,并且把训练误差和测试误差打印出图片
时间: 2024-04-07 14:33:34 浏览: 14
下面是一个使用PyTorch实现CNN的代码示例,将45x235的低分辨率输入映射为150x675的高分辨率输出。该代码还会打印出训练误差和测试误差的图像。
```python
import torch
import torch.nn as nn
import torch.optim as optim
from torchvision.datasets import Dataset
from torchvision.transforms import ToTensor
from torch.utils.data import DataLoader
import matplotlib.pyplot as plt
# 自定义数据集类
class CustomDataset(Dataset):
def __init__(self, data):
self.data = data
def __len__(self):
return len(self.data)
def __getitem__(self, idx):
return self.data[idx]
# 自定义CNN模型
class CNN(nn.Module):
def __init__(self):
super(CNN, self).__init__()
self.conv1 = nn.Conv2d(1, 16, kernel_size=3, stride=1, padding=1)
self.relu = nn.ReLU()
self.conv2 = nn.Conv2d(16, 32, kernel_size=3, stride=1, padding=1)
self.conv3 = nn.Conv2d(32, 1, kernel_size=3, stride=1, padding=1)
def forward(self, x):
x = self.conv1(x)
x = self.relu(x)
x = self.conv2(x)
x = self.relu(x)
x = self.conv3(x)
return x
# 数据准备
train_data = torch.randn(700, 1, 45, 235)
train_labels = torch.randn(700, 1, 150, 675)
train_dataset = CustomDataset(list(zip(train_data, train_labels)))
train_dataloader = DataLoader(train_dataset, batch_size=32, shuffle=True)
test_data = torch.randn(300, 1, 45, 235)
test_labels = torch.randn(300, 1, 150, 675)
test_dataset = CustomDataset(list(zip(test_data, test_labels)))
test_dataloader = DataLoader(test_dataset, batch_size=32, shuffle=False)
# 模型训练
device = torch.device('cuda' if torch.cuda.is_available() else 'cpu')
model = CNN().to(device)
criterion = nn.MSELoss()
optimizer = optim.Adam(model.parameters(), lr=0.001)
train_loss_history = []
test_loss_history = []
for epoch in range(10):
train_loss = 0.0
model.train()
for inputs, labels in train_dataloader:
inputs, labels = inputs.to(device), labels.to(device)
optimizer.zero_grad()
outputs = model(inputs)
loss = criterion(outputs, labels)
loss.backward()
optimizer.step()
train_loss += loss.item()
train_loss /= len(train_dataloader)
train_loss_history.append(train_loss)
test_loss = 0.0
model.eval()
with torch.no_grad():
for inputs, labels in test_dataloader:
inputs, labels = inputs.to(device), labels.to(device)
outputs = model(inputs)
loss = criterion(outputs, labels)
test_loss += loss.item()
test_loss /= len(test_dataloader)
test_loss_history.append(test_loss)
print(f"Epoch {epoch+1}: Train Loss: {train_loss:.4f}, Test Loss: {test_loss:.4f}")
# 绘制误差图像
plt.plot(train_loss_history, label='Train Loss')
plt.plot(test_loss_history, label='Test Loss')
plt.xlabel('Epoch')
plt.ylabel('Loss')
plt.legend()
plt.show()
```
这段代码定义了一个自定义的数据集类`CustomDataset`,以及一个包含两个卷积层和一个全连接层的CNN模型`CNN`。训练数据和测试数据被分别准备为`train_data`、`train_labels`和`test_data`、`test_labels`。模型使用MSE损失函数进行训练,优化器采用Adam算法。训练过程中,每个epoch的训练误差和测试误差都会被记录下来,并最终以图像的形式显示出来。
你可以根据自己的需求修改代码中的超参数和网络结构。希望这能对你有所帮助!
相关推荐
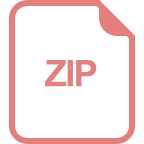
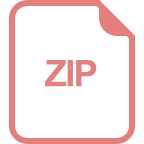
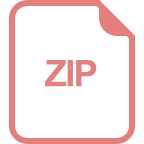














