low-rank approximation
时间: 2023-04-30 11:06:12 浏览: 182
低秩逼近,是一种通过用低维空间的数据来逼近高维空间数据的方法。这种方法可以应用于矩阵、图像处理、信号处理等领域。低秩逼近的目的是为了在保持重要信息的同时降低数据的维度,以达到节省存储空间和加快计算的效果。
相关问题
Try to apply the SVD method to factorize the matrix M,矩阵大小为K*N into two low-rank matrices, namely matrix U,矩阵大小为K*d and matrix I,矩阵大小为d*N, where d is an empirical parameter in practice which is set as 16 in this experiment. Please complete the following tasks. You can directly apply existing API in this quiz
Sure, I can help you with that. To apply the SVD method to factorize the matrix M into two low-rank matrices U and I, we can use the svd function from the numpy library in Python. Here's how you can do it:
```python
import numpy as np
# Define the matrix M
M = np.array([[1, 2, 3], [4, 5, 6], [7, 8, 9], [10, 11, 12]])
# Set the value of d
d = 2
# Apply the SVD method to factorize M into U and I
U, S, I = np.linalg.svd(M, full_matrices=False)
U = U[:, :d]
S = np.diag(S[:d])
I = I[:d, :]
# Calculate the low-rank approximation of M using U, S, and I
M_approx = np.dot(np.dot(U, S), I)
print("Matrix M:")
print(M)
print("Low-rank approximation of M:")
print(M_approx)
```
In this example, we have set the value of d to 2, which means we want to factorize the matrix M into two low-rank matrices with rank 2. The SVD method returns three matrices: U, S, and I. We select the first d columns of U, the first d rows and columns of S, and the first d rows of I to obtain the low-rank approximation of M. The resulting low-rank approximation is stored in the variable M_approx.
I hope this helps! Let me know if you have any more questions.
class SVDRecommender: def init(self, k=50, ncv=None, tol=0, which='LM', v0=None, maxiter=None, return_singular_vectors=True, solver='arpack'): self.k = k self.ncv = ncv self.tol = tol self.which = which self.v0 = v0 self.maxiter = maxiter self.return_singular_vectors = return_singular_vectors self.solver = solver def svds(self, A): if which == 'LM': largest = True elif which == 'SM': largest = False else: raise ValueError("which must be either 'LM' or 'SM'.") if not (isinstance(A, LinearOperator) or isspmatrix(A) or is_pydata_spmatrix(A)): A = np.asarray(A) n, m = A.shape if k <= 0 or k >= min(n, m): raise ValueError("k must be between 1 and min(A.shape), k=%d" % k) if isinstance(A, LinearOperator): if n > m: X_dot = A.matvec X_matmat = A.matmat XH_dot = A.rmatvec XH_mat = A.rmatmat else: X_dot = A.rmatvec X_matmat = A.rmatmat XH_dot = A.matvec XH_mat = A.matmat dtype = getattr(A, 'dtype', None) if dtype is None: dtype = A.dot(np.zeros([m, 1])).dtype else: if n > m: X_dot = X_matmat = A.dot XH_dot = XH_mat = _herm(A).dot else: XH_dot = XH_mat = A.dot X_dot = X_matmat = _herm(A).dot def matvec_XH_X(x): return XH_dot(X_dot(x)) def matmat_XH_X(x): return XH_mat(X_matmat(x)) XH_X = LinearOperator(matvec=matvec_XH_X, dtype=A.dtype, matmat=matmat_XH_X, shape=(min(A.shape), min(A.shape))) # Get a low rank approximation of the implicitly defined gramian matrix. eigvals, eigvec = eigsh(XH_X, k=k, tol=tol ** 2, maxiter=maxiter, ncv=ncv, which=which, v0=v0) # Gramian matrix has real non-negative eigenvalues. eigvals = np.maximum(eigvals.real, 0) # Use complex detection of small eigenvalues from pinvh. t = eigvec.dtype.char.lower() factor = {'f': 1E3, 'd': 1E6} cond = factor[t] * np.finfo(t).eps cutoff = cond * np.max(eigvals) # Get a mask indicating which eigenpairs are not degenerate tiny, # and create a reordering array for thresholded singular values. above_cutoff = (eigvals > cutoff) nlarge = above_cutoff.sum() nsmall = k - nlarge slarge = np.sqrt(eigvals[above_cutoff]) s = np.zeros_like(eigvals) s[:nlarge] = slarge if not return_singular_vectors: return np.sort(s) if n > m: vlarge = eigvec[:, above_cutoff] ularge = X_matmat(vlarge) / slarge if return_singular_vectors != 'vh' else None vhlarge = _herm(vlarge) else: ularge = eigvec[:, above_cutoff] vhlarge = _herm(X_matmat(ularge) / slarge) if return_singular_vectors != 'u' else None u = _augmented_orthonormal_cols(ularge, nsmall) if ularge is not None else None vh = _augmented_orthonormal_rows(vhlarge, nsmall) if vhlarge is not None else None indexes_sorted = np.argsort(s) s = s[indexes_sorted] if u is not None: u = u[:, indexes_sorted] if vh is not None: vh = vh[indexes_sorted] return u, s, vh将这段代码放入一个.py文件中,用Spyder查看,有报错,可能是缩进有问题,无法被调用,根据这个问题,给出解决办法,给出改正后的完整代码
好的,根据你提供的代码,我发现其中存在一些缩进错误,导致代码无法运行。下面是修改后的完整代码,请注意缩进:
```python
import numpy as np
from scipy.sparse.linalg import eigsh, LinearOperator
from scipy.sparse import isspmatrix, is_pydata_spmatrix
class SVDRecommender:
def __init__(self, k=50, ncv=None, tol=0, which='LM', v0=None,
maxiter=None, return_singular_vectors=True, solver='arpack'):
self.k = k
self.ncv = ncv
self.tol = tol
self.which = which
self.v0 = v0
self.maxiter = maxiter
self.return_singular_vectors = return_singular_vectors
self.solver = solver
def svds(self, A):
if self.which == 'LM':
largest = True
elif self.which == 'SM':
largest = False
else:
raise ValueError("which must be either 'LM' or 'SM'.")
if not (isinstance(A, LinearOperator) or isspmatrix(A) or is_pydata_spmatrix(A)):
A = np.asarray(A)
n, m = A.shape
if self.k <= 0 or self.k >= min(n, m):
raise ValueError("k must be between 1 and min(A.shape), k=%d" % self.k)
if isinstance(A, LinearOperator):
if n > m:
X_dot = A.matvec
X_matmat = A.matmat
XH_dot = A.rmatvec
XH_mat = A.rmatmat
else:
X_dot = A.rmatvec
X_matmat = A.rmatmat
XH_dot = A.matvec
XH_mat = A.matmat
dtype = getattr(A, 'dtype', None)
if dtype is None:
dtype = A.dot(np.zeros([m, 1])).dtype
else:
if n > m:
X_dot = X_matmat = A.dot
XH_dot = XH_mat = _herm(A).dot
else:
XH_dot = XH_mat = A.dot
X_dot = X_matmat = _herm(A).dot
def matvec_XH_X(x):
return XH_dot(X_dot(x))
def matmat_XH_X(x):
return XH_mat(X_matmat(x))
XH_X = LinearOperator(matvec=matvec_XH_X, dtype=A.dtype, matmat=matmat_XH_X, shape=(min(A.shape), min(A.shape)))
# Get a low rank approximation of the implicitly defined gramian matrix.
eigvals, eigvec = eigsh(XH_X, k=self.k, tol=self.tol ** 2, maxiter=self.maxiter, ncv=self.ncv,
which=self.which, v0=self.v0)
# Gramian matrix has real non-negative eigenvalues.
eigvals = np.maximum(eigvals.real, 0)
# Use complex detection of small eigenvalues from pinvh.
t = eigvec.dtype.char.lower()
factor = {'f': 1E3, 'd': 1E6}
cond = factor[t] * np.finfo(t).eps
cutoff = cond * np.max(eigvals)
# Get a mask indicating which eigenpairs are not degenerate tiny,
# and create a reordering array for thresholded singular values.
above_cutoff = (eigvals > cutoff)
nlarge = above_cutoff.sum()
nsmall = self.k - nlarge
slarge = np.sqrt(eigvals[above_cutoff])
s = np.zeros_like(eigvals)
s[:nlarge] = slarge
if not self.return_singular_vectors:
return np.sort(s)
if n > m:
vlarge = eigvec[:, above_cutoff]
ularge = X_matmat(vlarge) / slarge if self.return_singular_vectors != 'vh' else None
vhlarge = _herm(vlarge)
else:
ularge = eigvec[:, above_cutoff]
vhlarge = _herm(X_matmat(ularge) / slarge) if self.return_singular_vectors != 'u' else None
u = _augmented_orthonormal_cols(ularge, nsmall) if ularge is not None else None
vh = _augmented_orthonormal_rows(vhlarge, nsmall) if vhlarge is not None else None
indexes_sorted = np.argsort(s)
s = s[indexes_sorted]
if u is not None:
u = u[:, indexes_sorted]
if vh is not None:
vh = vh[indexes_sorted]
return u, s, vh
def _augmented_orthonormal_cols(U, n):
if U.shape[0] <= n:
return U
Q, R = np.linalg.qr(U)
return Q[:, :n]
def _augmented_orthonormal_rows(V, n):
if V.shape[1] <= n:
return V
Q, R = np.linalg.qr(V.T)
return Q[:, :n].T
def _herm(x):
return np.conjugate(x.T)
阅读全文
相关推荐
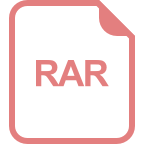
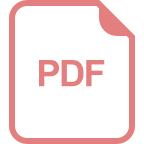
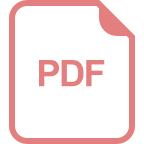
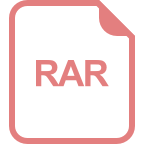
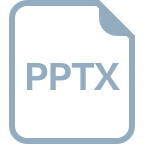
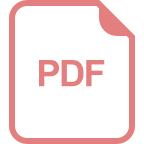
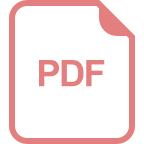
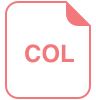
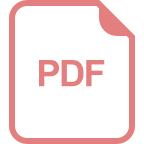
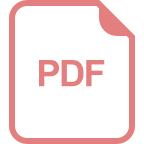
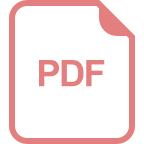
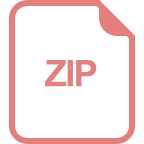
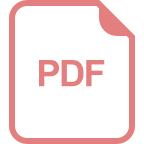
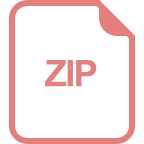
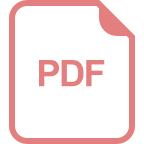
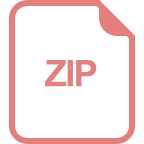