用pytorch实现logistic回归,要有数据准备、线性方程、激活函数、损失函数、优化算法、模型可视化,写出代码
时间: 2023-05-19 09:05:52 浏览: 100
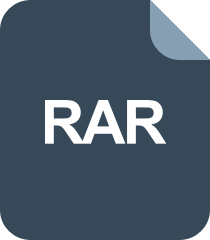
机器学习原理之logistic回归算法与代码实现 .rar_logistic回归_原理与实现_回归_机器学习;_深度学习

以下是使用 PyTorch 实现 Logistic 回归的代码,包括数据准备、线性方程、激活函数、损失函数、优化算法和模型可视化:
```python
import torch
import torch.nn as nn
import torch.optim as optim
import matplotlib.pyplot as plt
# 准备数据
x_train = torch.randn(100, 2)
y_train = torch.zeros(100)
y_train[(x_train[:, 0] + x_train[:, 1]) > 0] = 1
# 定义模型
class LogisticRegression(nn.Module):
def __init__(self):
super(LogisticRegression, self).__init__()
self.linear = nn.Linear(2, 1)
self.sigmoid = nn.Sigmoid()
def forward(self, x):
x = self.linear(x)
x = self.sigmoid(x)
return x
model = LogisticRegression()
# 定义损失函数和优化器
criterion = nn.BCELoss()
optimizer = optim.SGD(model.parameters(), lr=0.1)
# 训练模型
for epoch in range(1000):
optimizer.zero_grad()
y_pred = model(x_train)
loss = criterion(y_pred.squeeze(), y_train)
loss.backward()
optimizer.step()
# 可视化模型
w, b = model.linear.weight.squeeze().detach().numpy(), model.linear.bias.detach().numpy()
plt.scatter(x_train[:, 0], x_train[:, 1], c=y_train)
x_min, x_max = plt.xlim()
y_min, y_max = plt.ylim()
xx, yy = torch.meshgrid(torch.linspace(x_min, x_max, 100), torch.linspace(y_min, y_max, 100))
z = model(torch.cat([xx.reshape(-1, 1), yy.reshape(-1, 1)], dim=1)).detach().numpy().reshape(xx.shape)
plt.contour(xx, yy, z, levels=[0.5])
plt.plot([-b/w[0], 0], [0, -b/w[1]])
plt.show()
```
这段代码实现了一个简单的 Logistic 回归模型,使用 PyTorch 实现。其中,数据准备部分生成了一个二维的随机数据集,并根据数据的和是否大于 0 来生成标签;模型部分定义了一个包含线性层和 Sigmoid 激活函数的 Logistic 回归模型;损失函数使用了二元交叉熵损失函数;优化器使用了随机梯度下降算法。在训练模型后,使用 matplotlib 库可视化了模型的决策边界。
阅读全文
相关推荐
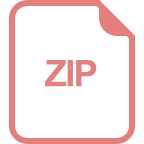


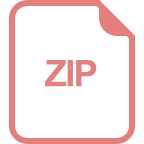
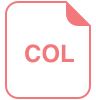
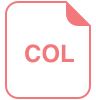
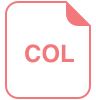
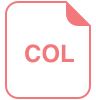
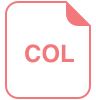
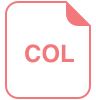
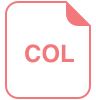
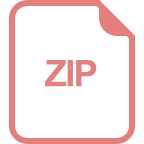
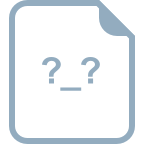
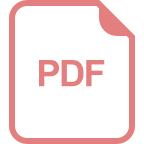
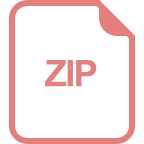