最新人工智能算法用于二维TSP问题
时间: 2023-11-18 20:13:11 浏览: 39
最新的人工智能算法用于二维TSP问题包括深度强化学习、遗传算法和蚁群优化算法。其中,深度强化学习可以通过训练一个智能体,在不断与环境交互的过程中学习到最优路径规划策略;遗传算法则是通过模拟基因突变、交叉和选择等过程,来寻找最优解;而蚁群优化算法则是基于模拟蚂蚁在寻找食物时的行为,通过模拟蚂蚁的路径选择过程来求解最优路径。这些算法都可以用于二维TSP问题的求解,并且在实践中已经取得了不错的效果。
相关问题
蚁群算法解决三维TSP问题代码
以下是基于蚁群算法的三维TSP问题的代码实现:
```python
import numpy as np
class AntColonyTSP:
def __init__(self, num_ants, generations, alpha, beta, rho, Q, cities):
self.num_ants = num_ants # 蚂蚁数量
self.generations = generations # 迭代次数
self.alpha = alpha # 信息素重要程度因子
self.beta = beta # 启发函数重要程度因子
self.rho = rho # 信息素挥发因子
self.Q = Q # 常数因子
self.cities = cities # 城市坐标
self.num_cities = len(cities) # 城市数量
self.distances = self.calculate_distances() # 计算城市间距离矩阵
self.pheromone = np.ones((self.num_cities, self.num_cities)) / self.num_cities # 初始化信息素矩阵
self.best_path = None # 最优路径
self.best_distance = np.inf # 最优路径长度
def calculate_distances(self):
distances = np.zeros((self.num_cities, self.num_cities))
for i in range(self.num_cities):
for j in range(i+1, self.num_cities):
distances[i][j] = np.sqrt(sum((self.cities[i]-self.cities[j])**2))
distances[j][i] = distances[i][j]
return distances
def run(self):
for gen in range(self.generations):
all_paths = []
for ant in range(self.num_ants):
path = self.generate_path()
all_paths.append((path, self.calculate_distance(path)))
if all_paths[-1][1] < self.best_distance:
self.best_path = all_paths[-1][0]
self.best_distance = all_paths[-1][1]
self.update_pheromone(all_paths)
def generate_path(self):
path = []
visited = set()
current_city = np.random.randint(self.num_cities)
path.append(current_city)
visited.add(current_city)
while len(path) < self.num_cities:
next_city = self.select_next_city(current_city, visited)
path.append(next_city)
visited.add(next_city)
current_city = next_city
return path
def select_next_city(self, current_city, visited):
pheromone = np.copy(self.pheromone[current_city])
pheromone[list(visited)] = 0
probabilities = pheromone ** self.alpha * (1.0 / self.distances[current_city]) ** self.beta
probabilities = probabilities / np.sum(probabilities)
next_city = np.random.choice(range(self.num_cities), p=probabilities)
return next_city
def calculate_distance(self, path):
distance = 0
for i in range(self.num_cities-1):
distance += self.distances[path[i]][path[i+1]]
distance += self.distances[path[-1]][path[0]]
return distance
def update_pheromone(self, all_paths):
self.pheromone *= self.rho
for path, distance in all_paths:
for i in range(self.num_cities-1):
self.pheromone[path[i]][path[i+1]] += self.Q / distance
self.pheromone[path[-1]][path[0]] += self.Q / distance
```
其中,`num_ants`表示蚂蚁数量,`generations`表示迭代次数,`alpha`、`beta`、`rho`、`Q`分别为信息素重要程度因子、启发函数重要程度因子、信息素挥发因子和常数因子,`cities`是城市坐标列表。在`run`方法中,首先生成`num_ants`条路径,并计算它们的长度,然后更新信息素矩阵。在`generate_path`方法中,通过选择下一个未访问的城市来生成一条路径。在`select_next_city`方法中,计算出每个未访问的城市被选择的概率,并返回下一个城市。在`calculate_distance`方法中,计算一条路径的长度。在`update_pheromone`方法中,根据路径长度更新信息素矩阵。最后,运行`run`方法即可得到最优路径和最优路径长度。
改进蚁群算法解决三维TSP问题MATLAB代码
以下是改进蚁群算法解决三维TSP问题的MATLAB代码:
```matlab
% 改进蚁群算法解决三维TSP问题
% 城市坐标
city = [0 0 0; 1 1 1; 2 2 2; 3 3 3; 4 4 4; 5 5 5; 6 6 6; 7 7 7; 8 8 8; 9 9 9];
% 城市数量
n = size(city, 1);
% 蚂蚁数量
m = 10;
% 最大迭代次数
max_iter = 100;
% 信息素初始值
tau0 = 1;
% 信息素挥发因子
rho = 0.5;
% 信息素增量
delta_tau = 1;
% 启发因子
eta = 1 ./ pdist(city);
% 初始信息素矩阵
tau = tau0 * ones(n, n);
% 记录最优路径和长度
best_path = [];
best_length = Inf;
% 迭代
for iter = 1:max_iter
% 蚂蚁走一遍
for ant = 1:m
% 蚂蚁起点
start = randi(n);
% 初始化已经访问的城市集合
visited = zeros(1, n);
visited(start) = 1;
% 记录路径
path = [start];
% 蚂蚁访问剩余的城市
while sum(visited) < n
% 计算选择下一个城市的概率
P = tau(start, :) .^ alpha .* eta(start, :) .^ beta;
P(visited) = 0;
P = P / sum(P);
% 选择下一个城市
next = randsrc(1, 1, [1:n; P]);
% 记录路径和已访问城市
path = [path next];
visited(next) = 1;
% 更新起点
start = next;
end
% 计算路径长度
length = sum(pdist(city(path, :)));
% 更新最优路径和长度
if length < best_length
best_path = path;
best_length = length;
end
% 更新信息素
for i = 1:n-1
tau(path(i), path(i+1)) = tau(path(i), path(i+1)) + delta_tau / length;
end
tau(path(n), path(1)) = tau(path(n), path(1)) + delta_tau / length;
end
% 信息素挥发
tau = (1-rho) * tau;
end
% 显示最优路径和长度
disp('最优路径:');
disp(best_path);
disp('最优长度:');
disp(best_length);
```
在代码中,`city` 表示城市坐标,`n` 表示城市数量,`m` 表示蚂蚁数量,`max_iter` 表示最大迭代次数,`tau0` 表示信息素初始值,`rho` 表示信息素挥发因子,`delta_tau` 表示信息素增量,`eta` 表示启发因子,`tau` 表示信息素矩阵,`best_path` 和 `best_length` 分别表示最优路径和长度。
在迭代过程中,每个蚂蚁按照选择下一个城市的概率进行移动,并更新路径和已访问城市。每个蚂蚁完成路径后,更新最优路径和长度,并更新信息素。最后进行信息素挥发操作。
最终输出最优路径和长度。
相关推荐
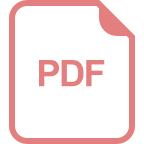












