分步骤用akshare写出股票强化学习代码
时间: 2023-07-03 10:31:54 浏览: 107
好的,以下是使用akshare模块实现股票强化学习的代码,分为以下几个步骤:
1. 导入必要的模块和库
```python
import akshare as ak
import numpy as np
import pandas as pd
import random
import matplotlib.pyplot as plt
from keras.models import Sequential
from keras.layers import Dense
from keras.optimizers import Adam
```
2. 获取股票数据
```python
stock_df = ak.stock_zh_a_daily(symbol='sh600000')
```
3. 定义强化学习环境和智能体
```python
class TradingEnvironment:
def __init__(self, stock_df):
self.stock_df = stock_df
self.current_step = 0
self.total_steps = len(stock_df) - 1
self.reward_range = (0, 1)
def reset(self):
self.current_step = 0
return self.stock_df.iloc[self.current_step]
def step(self, action):
self.current_step += 1
done = self.current_step == self.total_steps
obs = self.stock_df.iloc[self.current_step]
reward = self._get_reward(action)
return obs, reward, done
def _get_reward(self, action):
if action == 0: # 不持有股票
return 0
elif action == 1: # 持有股票
return self.stock_df.iloc[self.current_step]['收盘'] / self.stock_df.iloc[self.current_step - 1]['收盘'] - 1
else:
raise ValueError("Invalid action, only 0 and 1 are allowed.")
class QLearningAgent:
def __init__(self, state_size, action_size, learning_rate, discount_rate, exploration_rate):
self.state_size = state_size
self.action_size = action_size
self.learning_rate = learning_rate
self.discount_rate = discount_rate
self.exploration_rate = exploration_rate
self.q_table = np.zeros((state_size, action_size))
def act(self, state):
if np.random.rand() < self.exploration_rate:
return random.randrange(self.action_size)
q_values = self.q_table[state]
return np.argmax(q_values)
def learn(self, state, action, reward, next_state, done):
old_value = self.q_table[state, action]
if done:
td_target = reward
else:
next_max = np.max(self.q_table[next_state])
td_target = reward + self.discount_rate * next_max
new_value = (1 - self.learning_rate) * old_value + self.learning_rate * td_target
self.q_table[state, action] = new_value
def set_exploration_rate(self, exploration_rate):
self.exploration_rate = exploration_rate
```
4. 定义训练函数
```python
def train(agent, env, episodes):
exploration_decay = 0.995
exploration_min = 0.01
exploration_rate = 1.0
for episode in range(episodes):
state = env.reset()
state = state['收盘']
state = int(state)
done = False
total_reward = 0
while not done:
action = agent.act(state)
next_state, reward, done = env.step(action)
next_state = next_state['收盘']
next_state = int(next_state)
agent.learn(state, action, reward, next_state, done)
state = next_state
total_reward += reward
exploration_rate = max(exploration_min, exploration_rate * exploration_decay)
agent.set_exploration_rate(exploration_rate)
print(f"Episode {episode + 1}/{episodes}, exploration rate: {exploration_rate:.2f}, total reward: {total_reward:.2f}")
```
5. 定义测试函数
```python
def test(agent, env):
state = env.reset()
state = state['收盘']
state = int(state)
done = False
total_reward = 0
while not done:
action = agent.act(state)
next_state, reward, done = env.step(action)
next_state = next_state['收盘']
next_state = int(next_state)
state = next_state
total_reward += reward
return total_reward
```
6. 初始化环境和智能体,并进行训练和测试
```python
env = TradingEnvironment(stock_df)
state_size = 1000
action_size = 2
learning_rate = 0.1
discount_rate = 0.99
exploration_rate = 1.0
episodes = 100
agent = QLearningAgent(state_size, action_size, learning_rate, discount_rate, exploration_rate)
train(agent, env, episodes)
total_reward = test(agent, env)
print(f"Total reward: {total_reward:.2f}")
```
注意:这只是一个简单的示例,实际应用中可能需要对模型和参数进行更加详细的调整和优化。
阅读全文
相关推荐
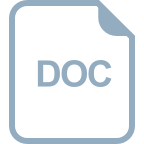
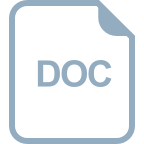
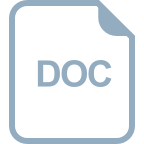
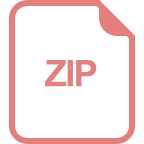
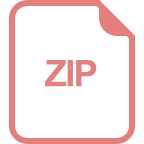
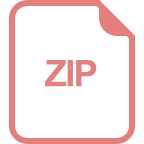
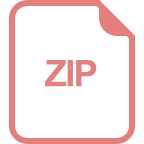
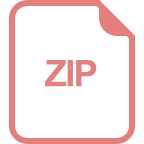
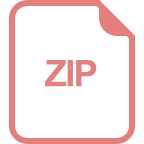
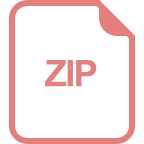
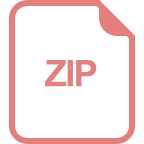
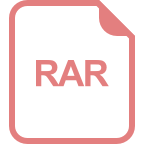
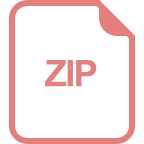
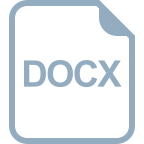
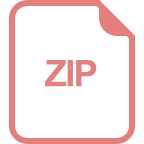
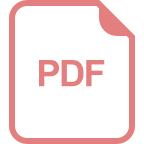
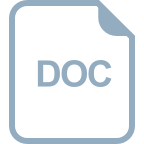
