优化代码 IS_DYLHZDYSZ = ((string.IsNullOrWhiteSpace(e.EDT_ITEM1) ? "0" : "1")=="1" || (string.IsNullOrWhiteSpace(f.EDT_ITEM2) ? "0" : "1")=="1")? "1" : "0"
时间: 2023-07-02 15:21:09 浏览: 141
可以将代码进行优化,简化为以下形式:
```
IS_DYLHZDYSZ = (!string.IsNullOrWhiteSpace(e.EDT_ITEM1) || !string.IsNullOrWhiteSpace(f.EDT_ITEM2)) ? "1" : "0";
```
这样可以减少代码行数,也更加清晰易懂。
相关问题
def cell_counter(image, min_area=20): """细胞计数""" # for s in image: df = pd.DataFrame() image =cv2.imread(image) gray = cv2.cvtColor(image, cv2.COLOR_BGR2GRAY) ret, thresh = cv2.threshold(gray, 100, 255, cv2.THRESH_BINARY + cv2.THRESH_OTSU) kernel = cv2.getStructuringElement(cv2.MORPH_RECT, (3, 3)) opening = cv2.morphologyEx(thresh, cv2.MORPH_OPEN, kernel, iterations=2) distance = ndi.distance_transform_edt(opening) coords = peak_local_max(distance, min_distance=9, footprint=np.ones((7, 7)), labels=opening) mask = np.zeros(distance.shape, dtype=bool) mask[tuple(coords.T)] = True markers, _ = ndi.label(mask) labels = watershed(-distance, markers, mask=opening, watershed_line=True) labels_area = [region.area for region in regionprops(labels) if region.area > min_area] cell_num = len(labels_area) print(cell_num) df = df.append(pd.DataFrame({(file_path,cell_num)}, index=[0]), ignore_index=True) print(df) # return cell_num # df.to_excel('1.xlsx', index=False) if __name__ == '__main__': path = r'D:\0531test' slide_path = os.listdir(path) # df =pd.DataFrame(slide_path) # df.to_excel('1.xlsx',index=False) for i in slide_path: slide_name = os.path.basename(i) #slide_name 样本名称 file_path = os.path.join(path,slide_name) images = os.listdir(file_path) f = glob.glob(os.path.join(file_path, '*.jpg')) for image in f: # print(s) # for s in images: # image_name = os.path.basename(s) # name = image_name.replace('.jpg','') # df = df.append(pd.DataFrame({(file_path,name[:-8])}, index=[0]), ignore_index=True) cell_counter(image) # df.to_excel('1.xlsx',index=False)
这段代码定义了一个名为 `cell_counter` 的函数,它接受一个参数 `image` 以及一个可选参数 `min_area`,默认值为 20。该函数的作用是对传入的图像进行细胞计数,并将计数结果存储在一个 Pandas DataFrame 中。具体的实现过程如下:
1. 创建一个空的 DataFrame `df`。
2. 使用 OpenCV 中的 `cv2.imread()` 函数读取图像。
3. 将图像从 BGR 色彩空间转换为灰度色彩空间,使用 `cv2.cvtColor()` 函数实现。
4. 对灰度图像进行二值化处理,使用 `cv2.threshold()` 函数实现。
5. 对二值化图像进行形态学开运算操作,使用 `cv2.morphologyEx()` 函数实现。
6. 使用 Scikit-image 中的 `ndi.distance_transform_edt()` 函数计算距离变换图像,表示像素到离它们最近的背景像素的距离。
7. 使用 Scikit-image 中的 `peak_local_max()` 函数从距离变换图像中寻找峰值点,作为细胞的中心点。
8. 使用 Scikit-image 中的 `watershed()` 函数基于距离变换图像和峰值点进行分水岭分割,将细胞区域分割出来。
9. 对于每个细胞区域,计算其面积,并存储在 `labels_area` 列表中。
10. 统计 `labels_area` 列表中的元素个数,即为细胞数量,存储在 `cell_num` 变量中。
11. 将 `(file_path, cell_num)` 存储在一个新的 DataFrame 中,并将该 DataFrame 追加到 `df` 中。
12. 打印 `df`。
13. 如果作为脚本运行,则遍历指定路径下的所有图像文件,调用 `cell_counter()` 函数,并将结果存储在一个名为 `1.xlsx` 的 Excel 文件中。
该函数的实现比较复杂,涉及到了多个图像处理库的函数。其中,Scikit-image 是一个常用的图像处理库,提供了许多常用函数和算法,可以方便地实现许多图像处理任务。
from skimage.morphology import skeletonize_3d from skimage import data # Load the 3D data points data = data.binary_blobs(length=100, blob_size_fraction=0.1, n_dim=3) # Convert the data points to a binary image binary_image = data.astype(bool) # Generate the distance map distance_map = ndimage.distance_transform_edt(binary_image) # Threshold the distance map thresholded_distance_map = (distance_map > 0) & (distance_map < 10) # Skeletonize the thresholded distance map skeleton = skeletonize_3d(thresholded_distance_map)对这段代码采用matplotlib进行可视化
好的,您可以尝试使用以下代码来可视化这段代码生成的三维骨架图像:
```python
import matplotlib.pyplot as plt
from mpl_toolkits.mplot3d.art3d import Poly3DCollection
fig = plt.figure()
ax = fig.add_subplot(111, projection='3d')
# Plot the binary image
x, y, z = binary_image.nonzero()
ax.scatter(x, y, z, zdir='z', c='red')
# Plot the skeleton
verts, faces, _, _ = measure.marching_cubes_lewiner(skeleton, 0)
mesh = Poly3DCollection(verts[faces], alpha=0.1)
face_color = [0.5, 0.5, 1]
mesh.set_facecolor(face_color)
ax.add_collection3d(mesh)
ax.set_xlim(0, binary_image.shape[0])
ax.set_ylim(0, binary_image.shape[1])
ax.set_zlim(0, binary_image.shape[2])
plt.show()
```
这段代码使用了matplotlib库中的3D可视化功能,将原始的二进制图像和骨架图像在三维空间中显示出来。其中,红色的点表示二进制图像中的非零点,蓝色的线条表示骨架图像。
阅读全文
相关推荐
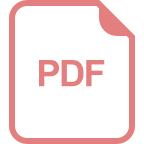


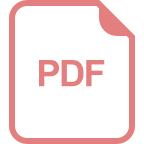
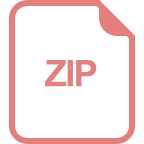
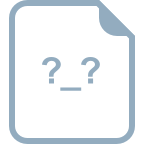
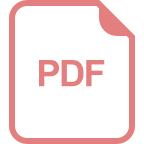
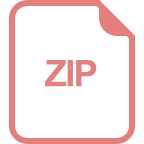
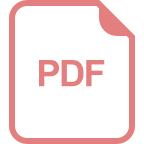
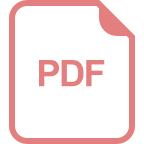




