data.drop_duplicates(subset = ‘columns1')
时间: 2024-05-27 15:08:51 浏览: 132
data.drop_duplicates(subset='columns1')是一个Python Pandas库中的函数,它用于删除DataFrame对象中的重复行。参数subset指定要检查重复值的列名或列名列表。在执行此函数时,将保留第一个与重复值匹配的行,并删除其余的重复行。这个函数可以帮助我们清理数据集中的重复数据,以便更好地进行分析和建模。
相关问题
tokens = [token for token in tokens if ((token not in stopwords) and len(token) > 1)] return tokens #当地址是根路径时,就调用下面的函数 @app.route('/') def index(): df = pd.read_csv("./球鞋.csv", encoding='gbk', header=None) # 数据清洗 df.columns = ["评论内容", 'user'] df.drop_duplicates(subset="评论内容", inplace=True) df.dropna(inplace=True) # 创建一个空的语料库列表 corpus = [] for text in df['评论内容'].values: # 预处理函数 preprocess_text() 的实现未知 tokens = preprocess_text(text) corpus.append(tokens) # 绘制词云图 dict_ = pd.DataFrame(_flatten(corpus)).value_counts().to_dict() dict_ = {i[0]: dict_[i] for i in dict_} data=[{"name":i,"value":dict_[i]} for i in dict_] print(data) return render_template("index.html",data=data) #启动web服务器 if __name__ == '__main__': app.run()
这段代码是一个使用 Flask 框架搭建的 web 服务器,读取了一个名为 "球鞋.csv" 的文件,进行数据清洗并生成词云图展示在网页上。具体步骤如下:
1. 使用 pandas 库读取 csv 文件,并给列名命名为 "评论内容" 和 "user"。
2. 对 "评论内容" 列进行去重和去空值操作。
3. 定义了一个预处理函数 preprocess_text(),用于对每个评论内容进行分词、去停用词等操作,返回一个 token 列表。
4. 遍历 "评论内容" 列,对每个评论内容调用 preprocess_text() 函数,将处理后的 token 列表添加到 corpus 列表中。
5. 对 corpus 列表进行扁平化处理,并使用 pandas 库的 value_counts() 函数统计每个词出现的次数,返回一个词频字典。
6. 将词频字典转换成一个格式为 [{"name":i,"value":dict_[i]}] 的列表,用于在网页上展示词云图。
7. 在网页上渲染 index.html 模板,并将词云图数据传递给模板。
8. 启动 web 服务器,监听客户端请求。
在收视行为信息数据中存在跨夜的记录数据,如开始观看时间与结束观看时间为05-12 23:45与05-13 00:31。这样的数据的记录需要分为两条记录,便于后面用户画像的构建。# 空值和重复值处理 media_data = media_data.dropna(subset=['owner_code']) media_data = media_data.drop_duplicates() # 时间格式转换 media_data['origin_time'] = pd.to_datetime(media_data['origin_time']) media_data['end_time'] = pd.to_datetime(media_data['end_time']) # 将 owner_code 列转换为字符串类型 media_data['owner_code'] = media_data['owner_code'].astype(str) # 将 owner_name 不包含 EA级、EB级、EC级、ED级、EE级 的数据保留 media_data = media_data[~media_data['owner_name'].isin(['EA级', 'EB级', 'EC级', 'ED级', 'EE级'])] # 删除 owner_code 为 2.0、9.0、10.0 的数据 media_data = media_data[~media_data['owner_code'].isin(['2.0', '9.0', '10.0'])] # 去掉 origin_time 和 end_time 相同的数据 media_data = media_data[media_data['origin_time'] != media_data['end_time']] # 保留用户同一时间观看的第一条数据 media_data = media_data.drop_duplicates(subset=['phone_no', 'origin_time', 'end_time'], keep='first')要怎么继续写
继续上面的数据处理代码,将跨夜的记录数据拆分为两条记录,可以按照以下步骤进行:
1. 创建一个空的DataFrame,用于存储拆分后的记录数据。
2. 遍历原始数据集media_data的每一行数据。
3. 对于每一行数据,判断开始观看时间和结束观看时间是否跨夜。
4. 如果跨夜,则将该行数据拆分为两条记录,一条记录的结束观看时间为当天的23:59:59,另一条记录的开始观看时间为次日的00:00:00。
5. 将拆分后的记录数据添加到新的DataFrame中。
6. 最后,将新的DataFrame与原始数据集media_data合并,得到包含拆分后记录的数据集。
下面是代码示例:
```python
import pandas as pd
# 创建空的DataFrame
split_data = pd.DataFrame(columns=media_data.columns)
# 遍历每一行数据
for index, row in media_data.iterrows():
# 判断开始观看时间和结束观看时间是否跨夜
if row['origin_time'].date() != row['end_time'].date():
# 拆分为两条记录
row1 = row.copy()
row1['end_time'] = pd.to_datetime(row['origin_time'].date()) + pd.DateOffset(hours=23, minutes=59, seconds=59)
row2 = row.copy()
row2['origin_time'] = pd.to_datetime(row['end_time'].date()) + pd.DateOffset(days=1)
# 添加到新的DataFrame中
split_data = split_data.append([row1, row2], ignore_index=True)
# 合并拆分后的记录数据和原始数据集
media_data = pd.concat([media_data, split_data])
# 重新排序索引
media_data = media_data.reset_index(drop=True)
```
这样,你就可以将跨夜的记录数据拆分为两条记录,并且将其添加到原始数据集中。同时,保留了其他的数据处理步骤。
阅读全文
相关推荐
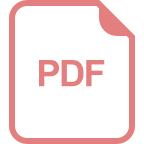
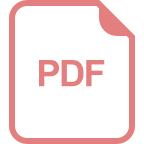
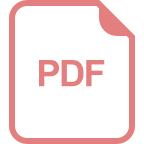





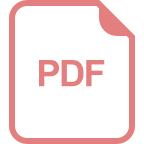
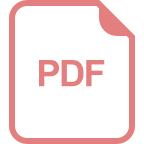
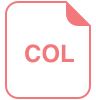
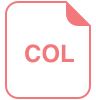
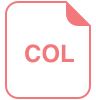



