x,y = datasets.make_classification(n_samples=100,n_features=2,n_classes=2,n_redundant=0,random_state=7816)什么意思,怎样将 x,y修改成此目录下的样本
时间: 2024-05-27 14:13:08 浏览: 16
这是一个生成二元分类数据集的函数,其中n_samples为样本数量,n_features为特征数量,n_classes为分类数量,n_redundant为多余特征数量,random_state为随机数种子。
要将x和y修改成此目录下的样本,需要先将目录下的样本读入,并按照相同的数据结构进行处理,然后再将x和y覆盖。但是,我并不知道此目录下的样本是什么,所以无法提供更具体的指导。
相关问题
2) 基于模拟数据分别使用KMeans聚类、DBSCAN算法对其进行聚类。用到如下知识点: sklearn.datasets.make_classification(n_samples=100, n_features=20, *, n_informative=2, n_redundant=2, n_repeated=0, n_classes=2, n_clusters_per_class=2, weights=None, flip_y=0.01, class_sep=1.0, hypercube=True
好的,你的问题是关于使用KMeans聚类和DBSCAN算法对模拟数据进行聚类的方法。那么我先来解释一下这两种算法的基本原理。
KMeans算法是一种常见的无监督学习算法,它的基本思想是将数据集划分为K个簇,使得簇内的点尽可能地相似,簇间的点尽可能地不同。它的过程是这样的:首先随机选取K个样本作为初始的聚类中心,然后将每个样本分配到距离最近的聚类中心所在的簇中,接着重新计算每个簇的中心点,直到聚类中心不再发生变化或达到指定的最大迭代次数为止。
DBSCAN算法是一种基于密度的聚类算法,它的基本思想是将高密度的区域划分为一个簇,低密度的区域作为噪声点。它的过程是这样的:首先随机选取一个未被访问的点作为核心点,然后找出所有与该点距离不超过指定半径的点,若这些点的数量不少于指定阈值,则将它们全部加入到同一个簇中,并以它们为新的核心点继续执行上述操作,直到所有的点都被访问过为止。
接下来,我来为你讲解如何使用这两种算法对模拟数据进行聚类。我们可以使用sklearn中的make_classification函数生成模拟数据。具体的代码如下:
```python
from sklearn.datasets import make_classification
X, y = make_classification(n_samples=100, n_features=20, n_informative=2, n_redundant=2,
n_classes=2, n_clusters_per_class=2, class_sep=1.0, random_state=42)
```
其中,n_samples表示生成的样本数量,n_features表示每个样本的特征数量,n_informative表示有用的特征数量,n_redundant表示冗余的特征数量,n_classes表示生成的类别数量,n_clusters_per_class表示每个类别中簇的数量,class_sep表示相邻两个簇之间的距离。
接下来,我们可以使用KMeans算法对数据进行聚类,代码如下:
```python
from sklearn.cluster import KMeans
kmeans = KMeans(n_clusters=2, random_state=42)
kmeans.fit(X)
y_pred = kmeans.predict(X)
```
其中,n_clusters表示需要聚类的簇的数量,random_state表示随机种子。
我们也可以使用DBSCAN算法对数据进行聚类,代码如下:
```python
from sklearn.cluster import DBSCAN
dbscan = DBSCAN(eps=0.5, min_samples=5)
dbscan.fit(X)
y_pred = dbscan.labels_
```
其中,eps表示指定半径的大小,min_samples表示指定阈值的大小。
最后,我们可以使用可视化工具将聚类结果可视化出来,代码如下:
```python
import matplotlib.pyplot as plt
plt.scatter(X[:, 0], X[:, 1], c=y_pred)
plt.show()
```
其中,X[:, 0]表示第一个特征,X[:, 1]表示第二个特征,c=y_pred表示以聚类结果为颜色值。
希望这些内容能够对你有所帮助!
# 随机生成数据集 X, y = make_classification(n_samples=100, n_features=10, n_classes=5, random_state=42,n_clusters_per_class=2,n_informative=5)
好的,这是一个使用 scikit-learn 库中的 make_classification 函数生成的数据集,包含100个样本,10个特征和5个类别。每个类别有2个簇,其中有5个特征是信息性特征。下面是代码实现:
```python
from sklearn.datasets import make_classification
X, y = make_classification(n_samples=100, n_features=10, n_classes=5, random_state=42, n_clusters_per_class=2, n_informative=5)
```
其中,n_samples 表示样本数,n_features 表示特征数,n_classes 表示类别数,random_state 是随机种子,n_clusters_per_class 表示每个类别有几个簇,n_informative 表示有几个信息性特征。函数返回的 X 是样本特征矩阵,y 是样本标签向量。
相关推荐
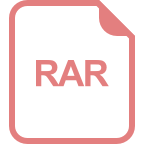
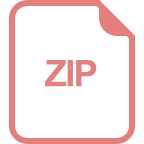













