The Non-IID Data Quagmire of Decentralized Machine Learning
时间: 2024-05-28 16:11:51 浏览: 11
Decentralized machine learning is a promising approach to train models on distributed data without the need for data sharing. However, one of the major challenges in this approach is dealing with non-IID (non-independent and identically distributed) data.
When data is non-IID, it means that the data samples across different participants have different statistical properties. This can happen when the data is collected from different sources, or when different participants have different data collection processes.
In decentralized machine learning, non-IID data can lead to several problems. For example, it can cause communication overheads, as participants need to exchange more data to train the model. It can also lead to slower convergence rates, as the model may struggle to generalize to new data that is different from the training data.
To overcome these challenges, researchers have proposed several techniques, such as data normalization, data augmentation, and model personalization. These techniques aim to make the data more IID-like and improve the performance of decentralized machine learning.
Overall, dealing with non-IID data is a significant challenge in decentralized machine learning, but with the right techniques and approaches, it is possible to overcome this quagmire and achieve accurate and efficient training on distributed data.
相关推荐
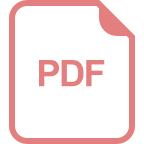
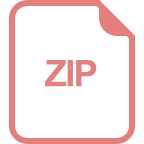
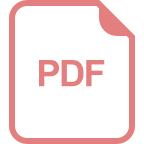














