how to use Covariance in locality preserving projection
时间: 2024-05-21 15:11:14 浏览: 12
Locality Preserving Projection (LPP) is a dimensionality reduction technique that aims to preserve the local structure of the data. It uses the covariance matrix of the data to achieve this goal.
Here are the steps to use covariance in LPP:
1. Compute the covariance matrix of the data. The covariance matrix is a matrix that quantifies the relationship between the different variables in the data. It can be computed using the formula:
Covariance matrix = (1/n) * ((X - μ) * (X - μ)T)
where X is the data matrix, μ is the mean of the data, and n is the number of data points.
2. Compute the affinity matrix. The affinity matrix is a matrix that quantifies the similarity between different data points. It can be computed using the formula:
Affinity matrix = exp(-||xi - xj||^2 / σ^2)
where xi and xj are two data points, ||.|| is the Euclidean distance between them, and σ is a parameter that controls the scale of the affinity matrix.
3. Compute the graph Laplacian. The graph Laplacian is a matrix that measures the smoothness of the data on the graph defined by the affinity matrix. It can be computed using the formula:
Graph Laplacian = D - W
where D is a diagonal matrix with the degree of each node on the diagonal, and W is the affinity matrix.
4. Compute the eigenvectors of the graph Laplacian. The eigenvectors of the graph Laplacian represent the new coordinates of the data in the reduced-dimensional space.
5. Select the k eigenvectors corresponding to the k smallest eigenvalues. These eigenvectors represent the k-dimensional subspace that preserves the local structure of the data.
6. Project the data onto the k-dimensional subspace. The projected data is obtained by multiplying the data matrix with the selected eigenvectors.
In summary, covariance is used in LPP to compute the affinity matrix, which is then used to compute the graph Laplacian. The eigenvectors of the graph Laplacian are used to project the data onto a lower-dimensional subspace that preserves the local structure of the data.
相关推荐
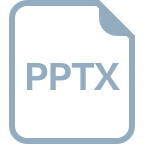
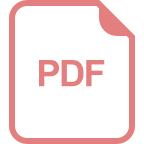
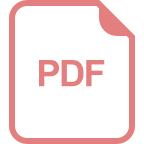














