allow_unreachable=True, accumulate_grad=True) # allow_unreachable flag RuntimeError: Trying to backward through the graph a second time (or directly access saved tensors after they have already been freed). Saved intermediate values of the graph are freed when you call .backward() or autograd.grad(). Specify retain_graph=True if you need to backward through the graph a second time or if you need to access saved tensors after calling backward.
时间: 2023-06-09 12:04:32 浏览: 490
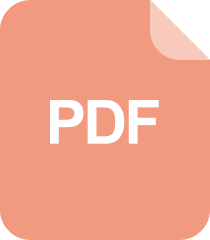
PHP5权威编程阅读学习笔记 附电子书下载
allow_unreachable=True,accumulate_grad=True是深度学习中的两个参数选项。其中,allow_unreachable=True表示允许非法的操作,这主要用于避免特定环境下的错误。而accumulate_grad=True表示要对梯度进行累加操作,这通常用于多个mini-batch进行梯度下降时避免每个mini-batch更新后梯度的丢失。
阅读全文
相关推荐
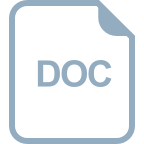
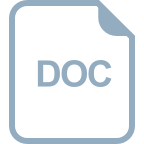















