用pytorch实现transformer中的位置编码
时间: 2023-09-16 15:06:32 浏览: 61
以下是使用PyTorch实现Transformer中的位置编码的示例代码:
```
import torch
import torch.nn as nn
import math
class PositionalEncoding(nn.Module):
"""
Positional encoding module for Transformer
"""
def __init__(self, d_model, max_seq_len=200, dropout=0.1):
"""
Args:
d_model (int): The number of expected features in the input
max_seq_len (int): The maximum length of the sequence
dropout (float): The probability of an element to be zeroed
"""
super(PositionalEncoding, self).__init__()
self.dropout = nn.Dropout(p=dropout)
# Compute the positional encodings once in log space.
pe = torch.zeros(max_seq_len, d_model)
position = torch.arange(0, max_seq_len, dtype=torch.float).unsqueeze(1)
div_term = torch.exp(torch.arange(0, d_model, 2).float() * (-math.log(10000.0) / d_model))
pe[:, 0::2] = torch.sin(position * div_term)
pe[:, 1::2] = torch.cos(position * div_term)
pe = pe.unsqueeze(0).transpose(0, 1)
self.register_buffer('pe', pe)
def forward(self, x):
"""
Args:
x: The input sequence of shape (seq_len, batch_size, d_model)
Returns:
The sequence with positional encoding
"""
x = x + self.pe[:x.size(0), :]
return self.dropout(x)
```
在这段代码中,我们定义了一个名为PositionalEncoding的类,该类是Transformer模型的一部分,并用于对输入序列进行位置编码。在__init__方法中,我们首先计算位置编码,即将正弦和余弦函数应用于不同频率的位置。然后,我们将位置编码作为一个buffer注册到模型中,以便在前向传递过程中使用。
在forward方法中,我们将输入序列与位置编码相加,并将结果传递给Dropout层,以便在训练过程中随机丢弃一些元素。最后,我们返回具有位置编码的序列。
相关推荐
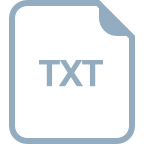
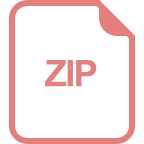
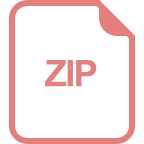














