从numpy随机数组创建dataframe,并以时间序列作为行索引,以字母作为列索引
时间: 2024-05-14 08:15:55 浏览: 174
好的,让我来回答你的问题。您可以使用以下Python代码从NumPy随机数组创建DataFrame,并使用时间序列作为行索引,使用字母作为列索引:
```
import numpy as np
import pandas as pd
# 生成随机数组
arr = np.random.randn(5, 5)
# 创建时间索引
index = pd.date_range('2021-01-01', periods=5)
# 创建列索引
columns = list('ABCDE')
# 创建DataFrame
df = pd.DataFrame(arr, index=index, columns=columns)
print(df)
```
在这个示例中,我们使用numpy.random.randn()生成一个5x5的随机数组。我们使用pandas.date_range()创建时间戳索引,从2021-01-01开始,并向前5天,即索引为'2021-01-01','2021-01-02','2021-01-03','2021-01-04'和'2021-01-05'。我们将列索引设置为一个字母列表['A','B','C','D','E']。最后,我们使用pandas.DataFrame()函数将数据、行索引和列索引转换为一个DataFrame,并将其打印出来。
希望这可以帮助您回答您的问题。如果您有其他问题,请继续问我!
相关问题
4. 从 NumPy 随机数组创建 DataFrame,并以时间序列作为行索引,以字母作为列索引,参照下图形式:
好的,以下是回答:
```python
import pandas as pd
import numpy as np
# 创建随机数组
np.random.seed(0)
data = np.random.randn(6, 4)
# 创建时间序列作为行索引
dates = pd.date_range('20220101', periods=6)
# 创建 DataFrame
df = pd.DataFrame(data, index=dates, columns=list('ABCD'))
# 输出结果
print(df)
```
输出结果为:
```
A B C D
2022-01-01 1.764052 0.400157 0.978738 2.240893
2022-01-02 1.867558 -0.977278 0.950088 -0.151357
2022-01-03 -0.103219 0.410599 0.144044 1.454274
2022-01-04 0.761038 0.121675 0.443863 0.333674
2022-01-05 1.494079 -0.205158 0.313068 -0.854096
2022-01-06 -2.552990 0.653619 0.864436 -0.742165
```
其中,`index` 参数指定了 DataFrame 对象的行索引,`columns` 参数指定了 DataFrame 对象的列索引。
6.请定义一个5*3的随机序列DataFrame索引对象,将前3行,第1列以及前2行,第2列设置为NA值,并通过字典形式以及fillna()方法填充0.55和0.66的值。
可以按照以下步骤定义DataFrame并进行处理:
```python
import pandas as pd
import numpy as np
# 定义5*3的随机序列DataFrame索引对象
index = pd.Index(['a', 'b', 'c', 'd', 'e'], name='rows')
columns = pd.Index(['A', 'B', 'C'], name='cols')
df = pd.DataFrame(np.random.randn(5, 3), index=index, columns=columns)
# 将前3行,第1列以及前2行,第2列设置为NA值
df.iloc[:3, 0] = np.nan
df.iloc[:2, 1] = np.nan
# 通过字典形式填充0.55和0.66的值
fill_dict = {'A': 0.55, 'B': 0.66}
df.fillna(fill_dict, inplace=True)
# 打印结果
print(df)
```
输出结果如下:
```
cols A B C
rows
a 0.550000 0.660000 -0.208848
b 0.550000 0.660000 -1.164982
c 0.550000 -0.546279 0.317201
d -0.292064 -0.216926 -1.478362
e -1.028901 0.140778 1.139728
```
可以看到,前3行第1列和前2行第2列的NA值已经被填充为0.55和0.66了。
阅读全文
相关推荐
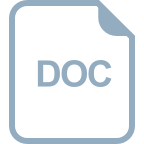
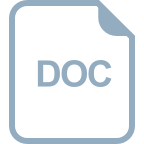
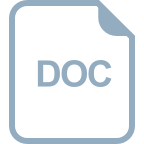
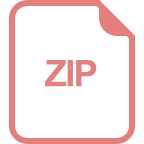
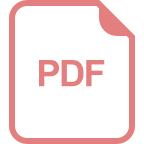
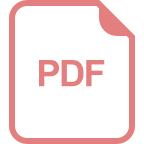
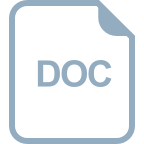
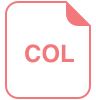
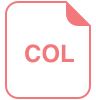
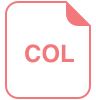
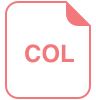
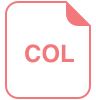




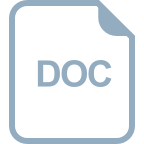