用yolov5定位车辆位置代码
时间: 2024-09-21 14:09:44 浏览: 36
YOLOv5(You Only Look Once version 5)是一个流行的开源目标检测模型,它主要用于实时图像和视频中物体的位置预测。在Python中,使用YOLOv5定位车辆位置的基本流程包括加载预训练模型、读取图片或视频帧、进行预测并解析结果。
以下是使用YOLOv5 Python API(如`yolov5`库)简单定位车辆位置的一个示例代码片段:
```python
# 导入必要的库
import cv2
from yolov5.yolov5 import YOLOv5
# 加载预训练模型
model = YOLOv5('yolov5s') # 可以替换为'yolov5m', 'yolov5l', 'yolov5x'等版本
# 读取图像
img_path = 'path_to_your_image.jpg'
image = cv2.imread(img_path)
# 进行预测
results = model.detect(image)
# 解析结果,找到车辆位置(通常返回的是边界框坐标)
for box in results:
x1, y1, x2, y2 = box['bbox']
vehicle_position = (int(x1), int(y1), int(x2-x1), int(y2-y1)) # 返回左上角(x1, y1)和右下角(x2, y2)坐标
print("Vehicle position:", vehicle_position)
相关问题
用yolov5定位视频中车辆位置代码
YOLOv5(You Only Look Once version 5)是一种流行的实时目标检测算法,用于在图像或视频中定位物体。在Python中,使用YOLOv5进行视频中车辆位置的定位通常需要以下几个步骤:
1. 安装库:首先安装`torch`, `pyyaml`, 和 `yolov5` 等必要的依赖项。你可以使用pip命令:
```
pip install torch torchvision fastai
pip install https://raw.githubusercontent.com/ultralytics/yolov5/master/requirements.txt
```
2. 加载模型:下载预训练的YOLOv5模型,如`yolov5s`, `yolov5m`, 或者`yolov5l`等,然后加载模型到内存中:
```python
from yolov5 import YOLOv5
yolo = YOLOv5('s') # 's', 'm', or 'l' for different model sizes
```
3. 执行预测:读取视频帧,并对每一帧应用模型进行检测:
```python
import cv2
cap = cv2.VideoCapture('your_video.mp4')
while True:
success, frame = cap.read()
if not success:
break
results = yolo.detect(frame)
boxes = results['boxes'] # 车辆的边界框
labels = results['labels'][results['scores'] > threshold] # 保留置信度高的车辆标签
scores = results['scores'][results['scores'] > threshold]
# 可视化结果(如果需要)
for box, label, score in zip(boxes, labels, scores):
# 将坐标从YOLO的输出转换为OpenCV可识别的格式
x1, y1, x2, y2 = [int(i) for i in box]
cv2.rectangle(frame, (x1, y1), (x2, y2), color=(0, 255, 0), thickness=2)
cv2.putText(frame, f"Vehicle ({label}) - {score:.2f}", (x1, y1 - 10), cv2.FONT_HERSHEY_SIMPLEX, 0.5, (0, 255, 0), 2)
cv2.imshow("Video", frame)
key = cv2.waitKey(1)
if key == ord('q'):
break
```
其中,`threshold`可以根据需要调整,以过滤掉低置信度的预测。
yolov8提取车辆速度代码
YOLOv8本身是一个目标检测框架,主要用于检测图像中的物体并给出位置和类别信息,而不是直接用于计算车辆的速度。如果你想要从YOLOv8的结果中分析车辆速度,通常需要结合其他信息,例如时间戳或连续帧之间的距离估计。
首先,你需要从YOLOv8的输出中获取到车辆的坐标信息(通常是bounding box),然后可以假设在一个固定的时间间隔内(如一帧的时间),通过比较相邻帧中同一辆车的位置变化,利用位移公式(车辆速度 = 距离 / 时间)来估算车辆的速度。这通常涉及到一些计算机视觉和机器学习之外的数据处理步骤。
以下是大概的伪代码示例:
```python
import cv2
# 假设yolov8返回的是每帧的物体检测结果列表
detections = yolov8(frame)
# 选择车辆类别(如果有多类)
vehicle_detections = [det for det in detections if det['class'] == 'car']
if vehicle_detections:
prev_box = None
current_time = time.time()
for detection in vehicle_detections:
x, y, w, h = detection['bbox']
# 计算当前车框相对于上一帧的位置变化
if prev_box is not None:
dx, dy = (x - prev_box[0], y - prev_box[1])
distance = np.sqrt(dx**2 + dy**2) * w * h # 假设w*h是单位面积下的像素数
dt = current_time - prev_time # 上一帧到当前帧的时间差
# 更新车辆速度和上一帧信息
speed = distance / dt if dt > 0 else 0
prev_box = (x, y, w, h)
prev_time = current_time
```
注意,这个过程依赖于实际的应用场景和数据质量,因为真实世界中可能存在车辆静止、运动速度过慢或YOLOv8定位误差等情况。为了获得准确的速度,可能还需要结合传感器数据或其他强化技术。
阅读全文
相关推荐
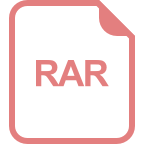
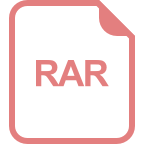
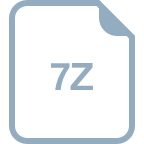
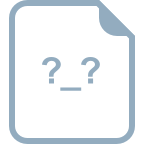
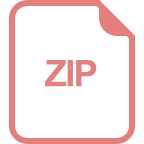
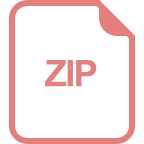
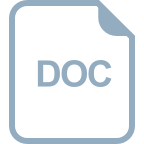
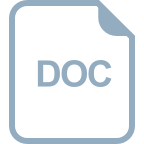

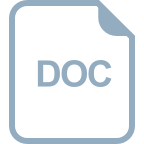
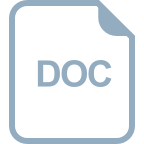
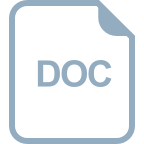
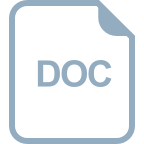
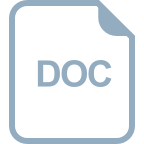
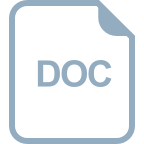
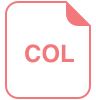
