kernel k-means matlab
时间: 2023-04-29 11:05:56 浏览: 75
kernel k-means 是用于聚类分析的一种算法,Matlab 是一种用于数学计算和科学数据可视化的软件。在 Matlab 中,你可以使用 kernel k-means 算法来对数据进行聚类分析。
相关问题
Simple Multiple Kernel K-means和Multiple Kernel K-means有什么区别与优点
Simple Multiple Kernel K-means (SMKK) 和 Multiple Kernel K-means (MKK) 都是基于多核学习的聚类算法,它们的主要区别和优点如下:
区别:
1. SMKK 是使用多个核函数来对数据进行表示,并且通过交替最小化目标函数的方式来进行聚类,而 MKK 则是使用多个核函数对数据进行不同的变换,然后将变换后的数据送入单个聚类算法进行聚类。
2. SMKK 聚类算法更加简单,计算效率更高,但是 MKK 的聚类性能更加稳定,而且在一些特殊的数据集上表现更好。
优点:
1. 使用多个核函数可以克服单个核函数的局限性,提高数据的表现能力,从而提高聚类的性能。
2. 在多核学习中,可以使用不同的核函数来表示数据的不同方面,从而提高聚类的鲁棒性和准确性。
3. 多核学习可以在不同的数据类型和数据结构上进行,具有较强的通用性和适应性。
总之,SMKK 和 MKK 都是基于多核学习的聚类算法,它们在聚类过程中采用了不同的策略和方法,但都利用了多核学习来提高聚类的性能。选择哪一种算法需要根据具体的任务需求和数据特征来进行选择。
Simple Multiple Kernel K-means和多核k聚类有什么区别
Simple Multiple Kernel K-means (SMKK) 和多核k聚类 (MKC) 是两种不同的聚类算法。
SMKK 是一种基于多核学习的聚类算法,它利用多个核函数来对数据进行表示,并且通过交替最小化目标函数的方式来进行聚类。SMKK 旨在通过使用多个核函数来克服单个核函数的局限性,从而提高聚类的性能。
MKC 也是一种基于多核学习的聚类算法,但它与 SMKK 的不同之处在于,MKC 利用多个核函数来对数据进行不同的变换,然后将变换后的数据送入单个聚类算法(如 k-means)进行聚类。MKC 旨在通过使用多个核函数来提高数据的表现能力,从而提高聚类的性能。
因此,SMKK 和 MKC 在聚类过程中采用了不同的策略和方法,但都利用了多核学习来提高聚类的性能。
相关推荐
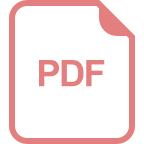
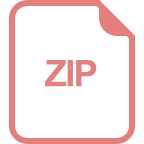












