forest = RandomForestClassifier(max_depth=6, min_samples_leaf=10, n_estimators=1000) def muti_score(model): # warnings.filterwarnings('ignore') accuracy = cross_val_score(model, X_train, Y_train, scoring='accuracy', cv=5) precision = cross_val_score(model, X_train, Y_train, scoring='precision', cv=5) recall = cross_val_score(model, X_train, Y_train, scoring='recall', cv=5) f1_score = cross_val_score(model, X_train, Y_train, scoring='f1', cv=5) auc = cross_val_score(model, X_train, Y_train, scoring='roc_auc', cv=5) print("准确率:",accuracy.mean()) print("精确率:",precision.mean()) print("召回率:",recall.mean()) print("F1_score:",f1_score.mean()) print("AUC:",auc.mean()) muti_score(forest)代码解释
时间: 2023-06-04 22:09:03 浏览: 108
forest = RandomForestClassifier(max_depth=6, min_samples_leaf=10, n_estimators=1000) 这句话定义了一个名为forest的随机森林分类器,其最大深度为6,每个叶节点上至少有10个样本,使用1000棵决策树。
def muti_score(model) 是一个自定义函数,其参数为一个机器学习模型,函数本身的功能则需要看具体实现。
相关问题
model = RandomForestClassifier(max_depth=3, n_estimators=10, min_samples_leaf=10, random_state=1) model.fit(X_train, y_train)
这是一个使用随机森林算法进行分类的模型,其中max_depth表示决策树的最大深度,n_estimators表示森林中树的数量,min_samples_leaf表示每个叶子节点至少需要包含的样本数量,random_state是一个随机种子,用于控制随机性。模型使用fit函数对训练集进行拟合,X_train是训练集的特征数据,y_train是训练集的标签数据。
rf_model = RandomForestRegressor(n_estimators=100, max_depth=20, random_state=42)
`RandomForestRegressor` 是 Scikit-Learn 库中的一个类,用于构建随机森林回归模型。随机森林是一种集成学习方法,通过结合多个决策树的预测结果来提高模型的准确性和稳定性。
以下是你提供的代码中各个参数的解释:
```python
rf_model = RandomForestRegressor(n_estimators=100, max_depth=20, random_state=42)
```
1. **n_estimators=100**: 这个参数指定了要使用的决策树的数量。在这个例子中,我们使用了100棵决策树。更多的树通常可以提高模型的性能,但也会增加计算成本。
2. **max_depth=20**: 这个参数限制了每棵决策树的最大深度。深度越大,模型可能会越复杂,但同时也更容易过拟合。在这个例子中,每棵树的最大深度被设置为20。
3. **random_state=42**: 这个参数设置了随机数生成器的种子,以确保每次运行代码时都能得到相同的结果。这对于调试和比较不同模型的性能非常有用。
下面是一个简单的示例,展示如何使用这个模型进行训练和预测:
```python
from sklearn.ensemble import RandomForestRegressor
from sklearn.model_selection import train_test_split
from sklearn.datasets import make_regression
# 生成一些示例数据
X, y = make_regression(n_samples=1000, n_features=10, noise=0.1, random_state=42)
# 将数据集分为训练集和测试集
X_train, X_test, y_train, y_test = train_test_split(X, y, test_size=0.2, random_state=42)
# 创建随机森林回归模型
rf_model = RandomForestRegressor(n_estimators=100, max_depth=20, random_state=42)
# 训练模型
rf_model.fit(X_train, y_train)
# 使用模型进行预测
y_pred = rf_model.predict(X_test)
# 输出预测结果
print(y_pred)
```
阅读全文
相关推荐
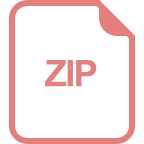
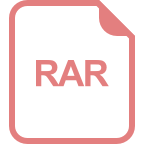
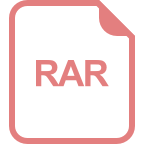













