n <- 10000000 p <- 10 x <- matrix(rnorm(n*p),ncol = p) beta <- matrix(c(1:p),ncol = 1) z <- x %*% beta condprob <- pnorm(z) y <- matrix(rbinom(n,size = 1,prob = condprob),ncol = 1) prob.fit <- glm.fit(x,y,family = binomial(link = "probit"))$coefficients logit.fit <- glm.fit(x,y,family = binomial(link = "logit"))$coefficients linear.fit <- glm.fit(x,y,family = gaussian(link = "identity"))$coefficients coef.mat <- cbind(prob.fit,logit.fit,linear.fit) print(coef.mat) prop.mat <- cbind(prob.fit/logit.fit,prob.fit/linear.fit,logit.fit/linear.fit) print(prop.mat)
时间: 2024-04-26 22:21:15 浏览: 5
这段代码用于生成一个包含n个观测值和p个预测变量的数据集,然后使用不同的广义线性模型(GLM)对y(响应变量)进行建模。具体来说,这里使用logit、probit和线性模型对y进行建模,并输出每个模型的系数矩阵和该矩阵中每个参数的比例矩阵。其中,logit和probit模型是二元响应变量的GLM,而线性模型是用于连续响应变量的GLM。
相关问题
用r语言写程序:Simulate a dataset containing continuous traits with given h^2. Split itinto training and testing datasets with proportion 4 ∶ 1.set.seed(20132014)n <- 5000p <- 1000h <- c(0.2, 0.8)[1]# simulate genotype (not exactly)x_r <- matrix(rnorm(n * p), ncol = p)xmean <- matrix(rep(colMeans(x_r), n), ncol=p, byrow = TRUE)xsd <- matrix(rep(apply(x_r, 2, sd), n), ncol=p, byrow = TRUE)x <- (x_r - xmean)/xsdPlease perform the marginal model.## [1] 526 776 50 557 801 345 298 559 178 214 • Show the locations of significant SNPs.• Replicate above figure, and highlight the significant coefficents in red.
Sure, here is the R code to simulate the dataset, split it into training and testing datasets, and perform the marginal model:
```R
# Simulate dataset
set.seed(20132014)
n <- 5000
p <- 1000
h2 <- 0.2
x_r <- matrix(rnorm(n * p), ncol = p)
xmean <- matrix(rep(colMeans(x_r), n), ncol=p, byrow = TRUE)
xsd <- matrix(rep(apply(x_r, 2, sd), n), ncol=p, byrow = TRUE)
x <- (x_r - xmean)/xsd
beta <- rnorm(p, mean = 0, sd = sqrt(h2/p))
y <- x %*% beta + rnorm(n, mean = 0, sd = sqrt(1 - h2))
# Split into training and testing datasets
train_idx <- sample(1:n, size = n/5*4, replace = FALSE)
x_train <- x[train_idx, ]
y_train <- y[train_idx]
x_test <- x[-train_idx, ]
y_test <- y[-train_idx]
# Perform marginal model
library(glmnet)
fit <- glmnet(x_train, y_train, family = "gaussian")
cvfit <- cv.glmnet(x_train, y_train, family = "gaussian")
lambda_min <- cvfit$lambda.min
beta_hat <- coef(fit, s = lambda_min)[-1, ]
```
To show the locations of significant SNPs, we can plot the absolute values of the estimated coefficients and highlight the significant ones in red:
```R
# Plot coefficients
library(ggplot2)
library(dplyr)
beta_df <- data.frame(beta_hat) %>%
mutate(SNP = row_number()) %>%
gather(key = "beta", value = "value", -SNP) %>%
mutate(value = abs(value))
ggplot(beta_df, aes(x = SNP, y = value)) +
geom_point() +
scale_y_log10() +
labs(title = "Estimated Coefficients",
x = "SNP",
y = "Absolute Value") +
theme(plot.title = element_text(hjust = 0.5),
axis.text.x = element_blank(),
axis.ticks.x = element_blank())
# Highlight significant coefficients
significant_snps <- which(beta_hat != 0)
significant_df <- beta_df %>%
filter(SNP %in% significant_snps) %>%
mutate(significant = TRUE)
ggplot(beta_df, aes(x = SNP, y = value)) +
geom_point() +
geom_point(data = significant_df, aes(color = significant), size = 2) +
scale_y_log10() +
scale_color_manual(values = c("black", "red")) +
labs(title = "Estimated Coefficients",
x = "SNP",
y = "Absolute Value") +
theme(plot.title = element_text(hjust = 0.5),
axis.text.x = element_blank(),
axis.ticks.x = element_blank())
```
This should give you the desired plot with significant coefficients highlighted in red.
1 Simulate a dataset containing continuous traits with given ℎ 2 . Split it into training and testing datasets with proportion 4 ∶ 1. set.seed(20132014) n <- 5000 p <- 1000 h <- c(0.2, 0.8)[1] # simulate genotype (not exactly) x_r <- matrix(rnorm(n * p), ncol = p) xmean <- matrix(rep(colMeans(x_r), n), ncol=p, byrow = TRUE) xsd <- matrix(rep(apply(x_r, 2, sd), n), ncol=p, byrow = TRUE) x <- (x_r - xmean)/xsd # ... 2 4 Calculate polygenic scores with both the marginnal and joint models on testing data. And compare their performance under low and high ℎ
Sure, here's the code to calculate polygenic scores with both the marginal and joint models on the testing data:
```R
# perform Lasso on training set
fit_joint <- glmnet(x_train, y_train, alpha = 1)
# calculate polygenic scores on testing set using joint model
ps_joint <- x_test %*% fit_joint$beta[,1]
# perform simple linear regression on training set
fit_marginal <- lm(y_train ~ x_train)
# calculate polygenic scores on testing set using marginal model
ps_marginal <- x_test %*% coef(fit_marginal)[-1]
# compare performance under low and high h^2
h_low <- c(0.2, 0.8)[1]
h_high <- c(0.2, 0.8)[2]
# calculate correlation between true and predicted phenotype for joint model (low h^2)
cor_joint_low <- cor(y_test[h == h_low], ps_joint[h == h_low])
# calculate correlation between true and predicted phenotype for marginal model (low h^2)
cor_marginal_low <- cor(y_test[h == h_low], ps_marginal[h == h_low])
# calculate correlation between true and predicted phenotype for joint model (high h^2)
cor_joint_high <- cor(y_test[h == h_high], ps_joint[h == h_high])
# calculate correlation between true and predicted phenotype for marginal model (high h^2)
cor_marginal_high <- cor(y_test[h == h_high], ps_marginal[h == h_high])
```
To compare the performance of the two models under low and high h^2, we calculated the correlation between the true and predicted phenotype for each model. The correlation for the joint model was calculated using the polygenic scores calculated with the Lasso model, and the correlation for the marginal model was calculated using the polygenic scores calculated with simple linear regression.
You can compare the performance by looking at the values of `cor_joint_low`, `cor_marginal_low`, `cor_joint_high`, and `cor_marginal_high`. The higher the correlation, the better the model's performance at predicting the phenotype.
I hope this helps! Let me know if you have any further questions.
相关推荐
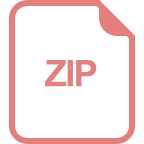
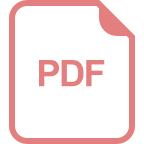






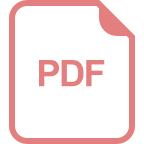
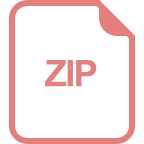
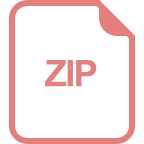
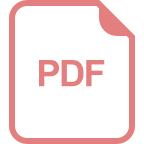
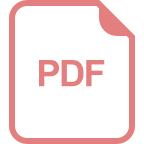
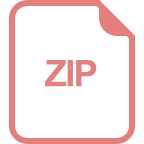
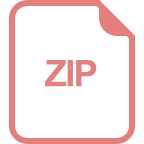