matlab主成分分析出图代码
时间: 2024-05-22 22:08:12 浏览: 134
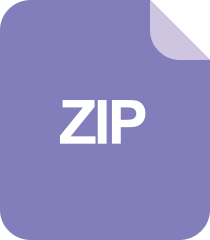
主成分分析法的MATLAB代码实现
在Matlab中使用主成分分析(PCA)进行数据降维和可视化,可以使用以下代码:
```
% 首先,加载数据
load fisheriris
% 将数据矩阵标准化
X = zscore(meas);
% 进行主成分分析
[coeff,score,latent] = pca(X);
% 绘制贡献度曲线,以可视化主成分的贡献度
pareto(latent)
xlabel('Principal Component')
ylabel('Variance Explained (%)')
% 绘制前两个主成分的散点图,以可视化数据在二维空间中的分布情况
gscatter(score(:,1),score(:,2),species,'rgb','osd');
xlabel('1st Principal Component')
ylabel('2nd Principal Component')
```
上面的代码将鸢尾花数据集进行了主成分分析,并绘制了贡献度曲线和前两个主成分的散点图,其中每种花的样本用不同的颜色和符号表示。
阅读全文
相关推荐
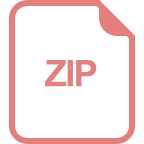
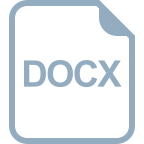














