多分支神经网络怎样根据权重梯度变化动态调整权重,给出代码
时间: 2024-03-19 08:46:06 浏览: 11
在多分支神经网络中,动态调整权重的方法与单一神经网络基本相同。以下是一个简单的多分支神经网络梯度下降的代码实现:
```
import numpy as np
# 定义激活函数
def sigmoid(x):
return 1 / (1 + np.exp(-x))
# 定义损失函数
def loss_function(y_pred, y_true):
return -np.mean(y_true * np.log(y_pred) + (1 - y_true) * np.log(1 - y_pred))
# 定义梯度下降函数
def gradient_descent(X, y, num_hidden_units, learning_rate=0.01, num_iterations=1000):
# 初始化权重和偏置
num_input_units = X.shape[1]
num_output_units = y.shape[1]
W1 = np.random.randn(num_input_units, num_hidden_units)
b1 = np.zeros(num_hidden_units)
W2 = np.random.randn(num_hidden_units, num_output_units)
b2 = np.zeros(num_output_units)
# 迭代训练
for i in range(num_iterations):
# 前向传播
z1 = np.dot(X, W1) + b1
h1 = sigmoid(z1)
z2 = np.dot(h1, W2) + b2
y_pred = sigmoid(z2)
# 反向传播
dz2 = y_pred - y
dW2 = np.dot(h1.T, dz2) / X.shape[0]
db2 = np.mean(dz2, axis=0)
dh1 = np.dot(dz2, W2.T)
dz1 = dh1 * h1 * (1 - h1)
dW1 = np.dot(X.T, dz1) / X.shape[0]
db1 = np.mean(dz1, axis=0)
# 更新权重和偏置
W2 -= learning_rate * dW2
b2 -= learning_rate * db2
W1 -= learning_rate * dW1
b1 -= learning_rate * db1
# 打印损失函数值
if i % 100 == 0:
print("Iteration %d, loss = %f" % (i, loss_function(y_pred, y)))
return W1, b1, W2, b2
```
在这个例子中,我们使用一个简单的两层多分支神经网络来演示梯度下降的过程。`gradient_descent` 函数中,我们首先初始化权重和偏置,然后对神经网络进行迭代训练,每次迭代中根据前向传播和反向传播计算梯度,并根据学习率和梯度信息更新权重和偏置。最后,我们输出训练过程中损失函数的值,可以看到在迭代过程中损失函数逐渐减小,说明神经网络的拟合效果逐渐变好。需要根据具体情况选择合适的方法,或者结合多种方法来解决训练数据不平衡的问题。
相关推荐
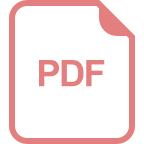
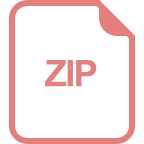














