orch.cuda.OutOfMemoryError: CUDA out of memory.
时间: 2023-11-05 20:49:58 浏览: 97
您好!对于CUDA内存不足的错误,有几种可能的解决方案:
1. 减少模型的尺寸:如果您正在使用一个较大的模型,可以尝试使用较小的模型来减少内存使用量。
2. 减少批处理大小:较大的批处理大小会占用更多的内存。您可以尝试减小批处理大小,以减少内存需求。
3. 使用混合精度训练:使用半精度浮点数(half precision)进行训练可以减少内存使用。您可以尝试使用PyTorch的amp(Automatic Mixed Precision)工具包来实现混合精度训练。
4. 释放无用的张量:请确保在使用完张量后及时释放它们,并通过调用`torch.cuda.empty_cache()`来清理缓存。
5. 增加GPU内存:如果您的GPU内存确实不足以容纳模型和数据,请考虑更换具有更大内存容量的GPU。
希望以上解决方案能够帮助您解决CUDA内存不足的问题!如有其他问题,请随时提问。
相关问题
orch.cuda.OutOfMemoryError: CUDA out of memory. Tried to allocate 34.00 MiB
orch.cuda.OutOfMemoryError: CUDA out of memory. Tried to allocate 34.00 MiB是由于在使用PyTorch运行时,尝试在GPU上分配超出可用内存容量的内存导致的错误。为了解决这个问题,可以尝试以下方法:
1. 减少模型的大小:可以通过选择更小的模型,减少模型的层数或通道数等方式来降低内存需求。
2. 减少批量大小:尝试减少输入数据的批量大小,以降低每次前向计算所需的内存。可以尝试调整`batch_size`参数的值。
3. 释放不需要的显存:在某些情况下,可能存在一些不需要的显存被占用。可以使用`torch.cuda.empty_cache()`来释放不需要的显存。
4. 启用混合精度训练:可以尝试启用混合精度训练,使用半精度浮点数(`torch.float16`)代替默认的单精度浮点数(`torch.float32`)。这可以显著降低内存使用,但可能会影响模型的训练效果。
5. 调整PyTorch内存管理设置:可以尝试调整PyTorch的内存管理设置,例如通过设置`max_split_size_mb`来避免内存碎片化。可以在PyTorch的文档中查找有关内存管理和`PYTORCH_CUDA_ALLOC_CONF`的详细信息。
这些方法可以帮助您解决`orch.cuda.OutOfMemoryError: CUDA out of memory.`的问题,并确保您的模型在GPU上顺利运行。<span class="em">1</span><span class="em">2</span><span class="em">3</span>
#### 引用[.reference_title]
- *1* [torch.cuda.OutOfMemoryError: CUDA out of memory.](https://blog.csdn.net/yyxz18281455491/article/details/130869597)[target="_blank" data-report-click={"spm":"1018.2226.3001.9630","extra":{"utm_source":"vip_chatgpt_common_search_pc_result","utm_medium":"distribute.pc_search_result.none-task-cask-2~all~insert_cask~default-1-null.142^v93^chatsearchT3_1"}}] [.reference_item style="max-width: 33.333333333333336%"]
- *2* [torch.cuda.OutOfMemoryError: CUDA out of memory. Tried to allocate 400.00 MiB (GPU 0; 6.00 GiB ...](https://blog.csdn.net/mjmald/article/details/131923220)[target="_blank" data-report-click={"spm":"1018.2226.3001.9630","extra":{"utm_source":"vip_chatgpt_common_search_pc_result","utm_medium":"distribute.pc_search_result.none-task-cask-2~all~insert_cask~default-1-null.142^v93^chatsearchT3_1"}}] [.reference_item style="max-width: 33.333333333333336%"]
- *3* [node-orch:兰花.js](https://download.csdn.net/download/weixin_42175971/19940119)[target="_blank" data-report-click={"spm":"1018.2226.3001.9630","extra":{"utm_source":"vip_chatgpt_common_search_pc_result","utm_medium":"distribute.pc_search_result.none-task-cask-2~all~insert_cask~default-1-null.142^v93^chatsearchT3_1"}}] [.reference_item style="max-width: 33.333333333333336%"]
[ .reference_list ]
orch::jit::script::Module module;
`torch::jit::script::Module` 是 PyTorch C++ API 中的一个类,用于表示一个 TorchScript 模型。具体来说,`Module` 由一些用于描述模型结构和参数的 `torch::jit::ClassType` 对象和一些用于执行模型的 `torch::jit::Function` 对象组成。在 C++ 中,我们可以通过以下方式声明一个 `Module` 对象:
```c++
#include <torch/script.h>
torch::jit::script::Module module;
```
在这个例子中,我们使用了 `#include <torch/script.h>` 来包含 PyTorch C++ API 的头文件。创建 `Module` 对象后,我们可以使用 `module.load()` 方法从磁盘上加载一个 TorchScript 模型,也可以使用 `module.forward(input)` 方法对输入数据进行前向计算,得到模型的输出结果。
阅读全文
相关推荐
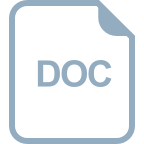
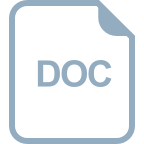







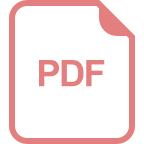
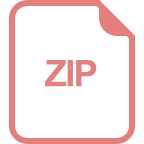
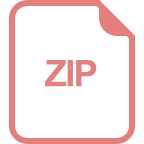




