基于深度学习的文本分类系统(完整代码+数据)bert+rnn textcnn fastcnn bert
时间: 2023-09-28 08:01:51 浏览: 141
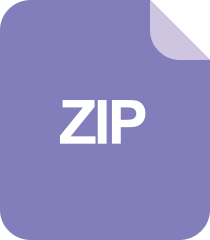
人工智能-深度学习-文本分类-使用Bert,ERNIE,进行中文文本分类

基于深度学习的文本分类系统常用的包括BERT、RNN、TextCNN、FastCNN等模型。下面将为您提供完整代码和数据。
首先,我们需要导入相关的库:tensorflow、keras、numpy等。
```python
import tensorflow as tf
from tensorflow.keras.preprocessing.text import Tokenizer
from tensorflow.keras.preprocessing.sequence import pad_sequences
from tensorflow.keras.layers import Dense, Embedding, LSTM, Conv1D, GlobalMaxPooling1D, Concatenate
from tensorflow.keras.models import Sequential
import numpy as np
```
接下来,我们准备训练数据和测试数据。假设已经准备好了train_texts和train_labels作为训练集的文本和标签,test_texts和test_labels作为测试集的文本和标签。
```python
train_texts = [...] # 训练集文本
train_labels = [...] # 训练集标签
test_texts = [...] # 测试集文本
test_labels = [...] # 测试集标签
```
然后,我们需要对文本进行预处理,将其转换为数值表示。这里我们使用Tokenizer将文本转换为单词索引序列。
```python
tokenizer = Tokenizer()
tokenizer.fit_on_texts(train_texts)
train_sequences = tokenizer.texts_to_sequences(train_texts)
test_sequences = tokenizer.texts_to_sequences(test_texts)
vocab_size = len(tokenizer.word_index) + 1 # 词汇表大小
```
接着,我们需要将序列填充为相同的长度,这里我们采用max_len作为填充长度。
```python
max_len = 100 # 填充长度
train_data = pad_sequences(train_sequences, maxlen=max_len)
test_data = pad_sequences(test_sequences, maxlen=max_len)
```
现在,我们可以构建基于RNN的文本分类模型了。
```python
model = Sequential()
model.add(Embedding(vocab_size, 100, input_length=max_len))
model.add(LSTM(128))
model.add(Dense(1, activation='sigmoid'))
model.compile(loss='binary_crossentropy', optimizer='adam', metrics=['accuracy'])
model.fit(train_data, train_labels, validation_data=(test_data, test_labels), epochs=10, batch_size=64)
```
如果您想使用TextCNN或FastCNN模型进行文本分类,可以参考以下代码:
```python
filters = 100
kernel_size = 3
model = Sequential()
model.add(Embedding(vocab_size, 100, input_length=max_len))
model.add(Conv1D(filters, kernel_size, activation='relu', padding='valid'))
model.add(GlobalMaxPooling1D())
model.add(Dense(1, activation='sigmoid'))
model.compile(loss='binary_crossentropy', optimizer='adam', metrics=['accuracy'])
model.fit(train_data, train_labels, validation_data=(test_data, test_labels), epochs=10, batch_size=64)
```
最后,对于BERT模型,您可以使用Hugging Face提供的transformers库。您可以根据自己的需求选择相应的BERT模型,例如bert-base-uncased、bert-large-uncased等。
```python
from transformers import BertTokenizer, TFBertModel
tokenizer = BertTokenizer.from_pretrained('bert-base-uncased')
train_encodings = tokenizer(train_texts, truncation=True, padding=True)
test_encodings = tokenizer(test_texts, truncation=True, padding=True)
train_dataset = tf.data.Dataset.from_tensor_slices((
dict(train_encodings),
train_labels
))
test_dataset = tf.data.Dataset.from_tensor_slices((
dict(test_encodings),
test_labels
))
model = TFBertModel.from_pretrained('bert-base-uncased')
input_ids = tf.keras.layers.Input(shape=(None,), dtype=tf.int32)
attention_mask = tf.keras.layers.Input(shape=(None,), dtype=tf.int32)
outputs = model(input_ids, attention_mask=attention_mask)
output = outputs[0][:, 0, :]
output = Dense(1, activation='sigmoid')(output)
model = tf.keras.models.Model(inputs=[input_ids, attention_mask], outputs=output)
model.compile(optimizer='adam',
loss='binary_crossentropy',
metrics=['accuracy'])
model.fit(train_dataset.shuffle(1000).batch(16), epochs=10, batch_size=16)
```
以上是基于深度学习的文本分类系统的完整代码和数据示例,您可以根据需要进行修改和调整。注意,BERT模型的训练可能需要较长的时间和更大的计算资源。
阅读全文
相关推荐
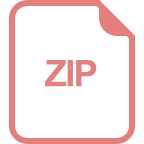
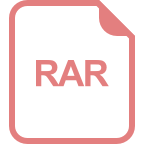
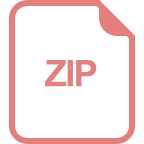
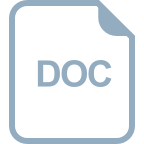
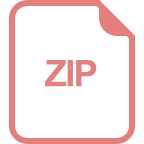
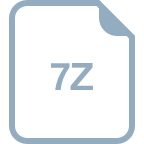
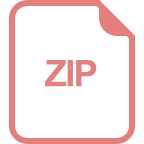
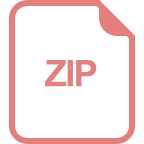
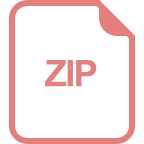
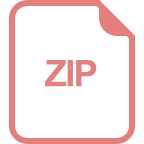
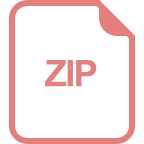
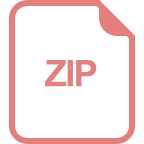
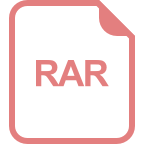
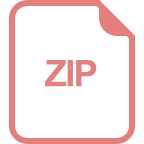
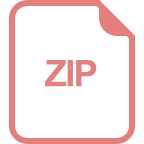
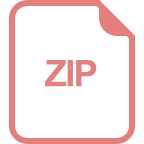
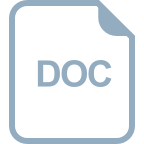