如何用Python编写主成分分析(PCA)和多元线性回归分析的集成代码示例,要求要有对应的结果输出和可视化?
时间: 2024-11-17 16:28:35 浏览: 43
在Python中,我们可以使用sklearn库来进行主成分分析(PCA)和多元线性回归分析。下面是一个简单的例子,首先导入所需的库,然后生成一些模拟数据,接着进行PCA降维,并用PCA结果作为特征输入到多元线性回归模型中。
```python
# 导入所需库
import numpy as np
import matplotlib.pyplot as plt
from sklearn.decomposition import PCA
from sklearn.linear_model import LinearRegression
from sklearn.model_selection import train_test_split
# 模拟数据
np.random.seed(42)
X = np.random.rand(100, 5) # 100个样本,每个样本有5个特征
y = 3 + 2 * X[:, 0] + 4 * X[:, 1] + 7 * X[:, 2] + 9 * X[:, 3] + 1 * X[:, 4] + np.random.randn(100)
# 划分训练集和测试集
X_train, X_test, y_train, y_test = train_test_split(X, y, test_size=0.2, random_state=42)
# 主成分分析
pca = PCA(n_components=2) # 我们只保留前两个主成分
X_train_pca = pca.fit_transform(X_train)
X_test_pca = pca.transform(X_test)
# 可视化PCA结果
plt.scatter(X_train_pca[:, 0], X_train_pca[:, 1], c=y_train, cmap='viridis')
plt.xlabel('Principal Component 1')
plt.ylabel('Principal Component 2')
plt.title('PCA Visualization of Training Data')
plt.show()
# 创建并训练多元线性回归模型
model = LinearRegression()
model.fit(X_train_pca, y_train)
# 预测并评估
y_pred = model.predict(X_test_pca)
print("Mean Absolute Error (MAE):", mean_absolute_error(y_test, y_pred))
# 输出系数和截距
print("Intercept:", model.intercept_)
print("Coefficient:", model.coef_)
```
在这个示例中,我们先通过PCA将高维数据降维到二维,然后使用降维后的数据进行线性回归。最后,我们会查看预测的MAE来评估模型性能,以及输出回归系数和截距信息。
阅读全文
相关推荐
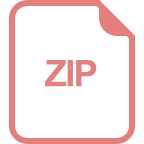
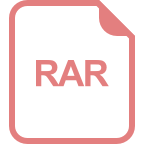
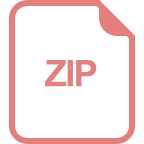
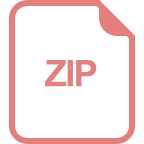
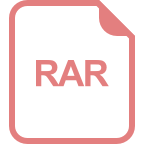
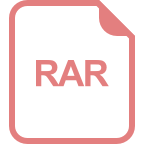
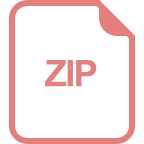
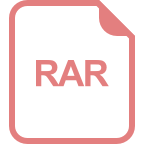
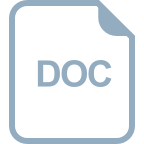
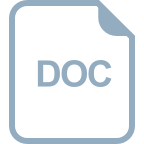
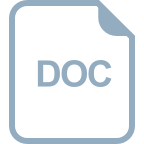
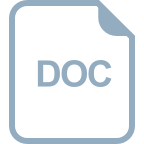
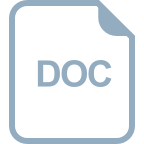
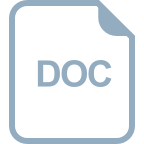
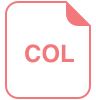
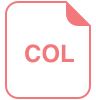
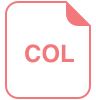

