请用matlab编写一段lstm代码
时间: 2024-01-16 13:05:27 浏览: 65
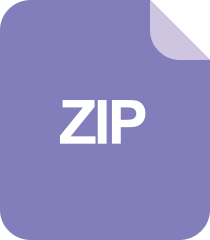
lstm-matlab代码
以下是一个简单的LSTM代码示例:
% 定义输入数据和标签
X = rand(100, 10);
Y = rand(100, 1);
% 定义LSTM网络参数
input_size = size(X, 2);
hidden_size = 64;
output_size = 1;
num_layers = 1;
% 定义LSTM网络结构
lstm_net = lstmLayer(hidden_size, 'OutputMode', 'last');
fc_net = fullyConnectedLayer(output_size);
softmax_net = regressionLayer();
% 将网络层连接起来
layers = [ ...
sequenceInputLayer(input_size)
lstm_net
fc_net
softmax_net
];
% 定义训练选项
options = trainingOptions('adam', ...
'MaxEpochs', 10, ...
'MiniBatchSize', 32, ...
'InitialLearnRate', 0.01, ...
'GradientThreshold', 1, ...
'Verbose', true);
% 训练LSTM网络
net = trainNetwork(X, Y, layers, options);
阅读全文
相关推荐
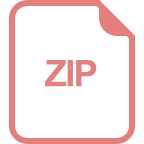


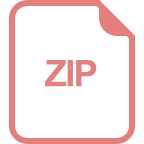


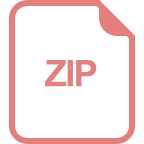
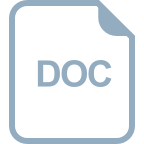
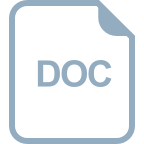
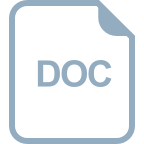
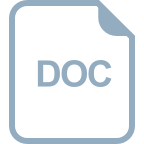
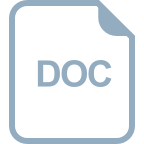
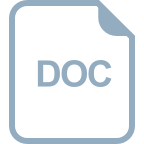
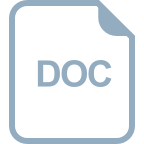


