训练完数据集后,如何用pytorch分类测试数据集中的图片
时间: 2023-11-22 08:51:55 浏览: 62
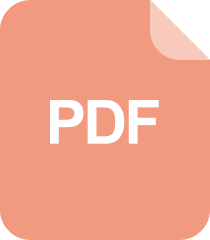
使用PyTorch将文件夹下的图片分为训练集和验证集实例

在使用 PyTorch 进行分类测试之前,需要做一些准备工作,包括:
1. 安装 PyTorch 库
2. 下载适当的数据集
3. 准备数据集并加载到 PyTorch 中
4. 定义模型
5. 定义训练过程和优化器
6. 加载模型
7. 对测试数据集进行分类并输出结果
下面是一个简单的代码示例,演示如何使用 PyTorch 进行分类测试:
```
import torch
import torchvision
import torchvision.transforms as transforms
# 定义数据预处理
transform = transforms.Compose(
[transforms.ToTensor(),
transforms.Normalize((0.5, 0.5, 0.5), (0.5, 0.5, 0.5))])
# 加载测试数据集
testset = torchvision.datasets.CIFAR10(root='./data', train=False,
download=True, transform=transform)
testloader = torch.utils.data.DataLoader(testset, batch_size=4,
shuffle=False, num_workers=2)
# 定义模型
class Net(torch.nn.Module):
def __init__(self):
super(Net, self).__init__()
self.conv1 = torch.nn.Conv2d(3, 6, 5)
self.pool = torch.nn.MaxPool2d(2, 2)
self.conv2 = torch.nn.Conv2d(6, 16, 5)
self.fc1 = torch.nn.Linear(16 * 5 * 5, 120)
self.fc2 = torch.nn.Linear(120, 84)
self.fc3 = torch.nn.Linear(84, 10)
def forward(self, x):
x = self.pool(torch.nn.functional.relu(self.conv1(x)))
x = self.pool(torch.nn.functional.relu(self.conv2(x)))
x = x.view(-1, 16 * 5 * 5)
x = torch.nn.functional.relu(self.fc1(x))
x = torch.nn.functional.relu(self.fc2(x))
x = self.fc3(x)
return x
net = Net()
# 加载模型
PATH = './cifar_net.pth'
net.load_state_dict(torch.load(PATH))
# 分类测试数据集
correct = 0
total = 0
with torch.no_grad():
for data in testloader:
images, labels = data
outputs = net(images)
_, predicted = torch.max(outputs.data, 1)
total += labels.size(0)
correct += (predicted == labels).sum().item()
print('Accuracy of the network on the 10000 test images: %d %%' % (
100 * correct / total))
```
在上面的代码中,我们使用 CIFAR-10 数据集作为测试数据集,定义了一个简单的卷积神经网络模型,加载了预训练好的模型,并对测试数据集进行了分类。最后输出了模型在测试数据集上的准确率。
阅读全文
相关推荐
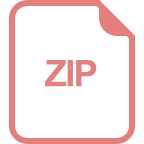
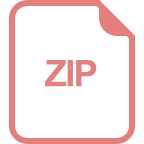















