iqa-nima 代码
时间: 2023-06-27 19:02:02 浏览: 237
### 回答1:
iqa-nima是一种针对图像质量评估的深度学习算法,利用神经网络自动学习图像质量的特征,可以准确地评估图像的质量。该算法是基于NIMA(Neural Image Assessment)算法的改进版本,增加了对ISO等其他图像质量因素的考虑。
iqa-nima算法主要包括两个部分:训练阶段和测试阶段。在训练阶段,算法利用已有的高质量图像和对应的主观评价分数训练神经网络,学习图像质量的特征。在测试阶段,通过输入待评估的图像,经过神经网络计算得到图像的质量分数,从而进行图像质量评估。
iqa-nima算法采用了效率较高的ResNet架构作为神经网络,在训练阶段引入了数据增强和随机扰动等策略,提高了算法的鲁棒性。在测试阶段,算法还提供了集成多个神经网络的选项,可以进一步提高评估结果的准确性。
总的来说,iqa-nima是一种高效准确的图像质量评估算法,可以广泛应用在图像处理和计算机视觉等领域。
### 回答2:
iqa-nima是一种用于评估图像质量的算法。它基于神经网络,并利用了自然图像的统计规律来预测图像的质量。iqa-nima的全称是“Natural Image Quality Evaluator using Multi-scale Spatial and Spectral Features with Gradient Boosting Machine”。与其他传统的图像质量评估算法相比,iqa-nima具有较高的精度和鲁棒性。在实际应用中,iqa-nima可以用于图像压缩、图像增强、图像复原等场景中,用于判断图像质量改变的程度。iqa-nima的实现过程主要包括图像预处理(将输入图像转换为多尺度和多频段的特征图)、特征提取(利用CNN从特征图中提取空间和频谱特征)、训练模型(采用GBM,构建回归模型进行参数学习和预测)和评估图像质量(将图像输入模型进行预测)。iqa-nima的代码较为复杂,需要深入掌握图像处理、机器学习等相关领域的知识。
### 回答3:
iqa-nima 是一种基于神经网络的图像质量评价算法。该算法使用了深度神经网络模型来学习图像质量的特征,然后预测图像的质量分数。iqa-nima 算法的主要特点是高精度和高效率。与传统的基于数学模型的评价方法相比,它能够更加准确地评估图像质量。
iqa-nima 算法的核心思想是将图像转化为特征向量,然后再将特征向量输入到神经网络模型中进行学习和预测。该模型采用了一种叫做残差模块的结构,使得模型具有更好的拟合能力和泛化能力,从而提高了模型的预测结果的准确性。
此外,iqa-nima 算法还使用了一种特殊的损失函数,称为对比损失函数。该损失函数能够引导模型学习到图像质量的一些高级特征,从而提高了模型的鲁棒性和可靠性。在训练阶段,模型需要学习尽可能地准确地预测每张图像的质量,而在测试阶段,模型可以对新的图像进行评价和预测,从而提供更加准确的结果。
阅读全文
相关推荐


















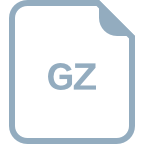