raise NonExistingLayerError("InputLayer(x, name='a') --> Input(name='a')(x)" + __log__) tensorlayer.layers.deprecated.NonExistingLayerError: InputLayer(x, name='a') --> Input(name='a')(x) Hint: 1) downgrade TF and TL from version 2.x to 1.x. 2) check the documentation of TF and TL version 2.x
时间: 2024-04-29 20:19:49 浏览: 285
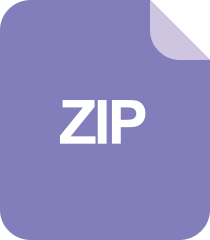
py_logging_usage:如何使用Python日志记录!
这个错误提示是由TensorLayer库中的一个函数抛出的,它表示在使用TensorLayer库中的某个函数时出现了错误。
从错误信息中可以看出,这个错误是因为在使用TensorLayer的InputLayer函数时,给定的参数不正确导致的。根据提示,你可以尝试通过以下方法解决这个问题:
1. 降级 TensorFlow 和 TensorLayer 版本,从 2.x 降至 1.x 版本。
2. 检查 TensorFlow 和 TensorLayer 版本 2.x 的文档,查看函数参数的正确用法。
你可以根据你的具体情况选择其中一种解决方法来解决这个问题。
阅读全文
相关推荐
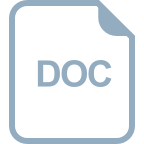
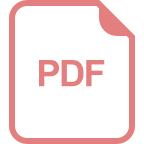
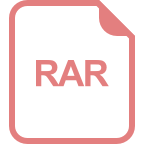
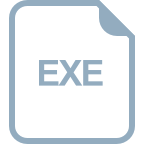
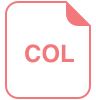
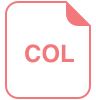
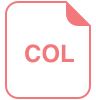
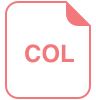
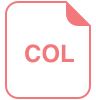
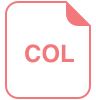
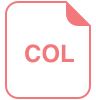
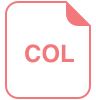
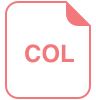
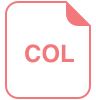
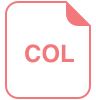
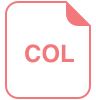
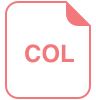