def MEAN_Spot(opt): # channel 1 inputs1 = layers.Input(shape=(42,42,1)) conv1 = layers.Conv2D(3, (5,5), padding='same', activation='relu', kernel_regularizer=l2(0.001))(inputs1) bn1 = layers.BatchNormalization()(conv1) pool1 = layers.MaxPooling2D(pool_size=(3, 3), padding='same', strides=(3,3))(bn1) do1 = layers.Dropout(0.3)(pool1) # channel 2 inputs2 = layers.Input(shape=(42,42,1)) conv2 = layers.Conv2D(3, (5,5), padding='same', activation='relu', kernel_regularizer=l2(0.001))(inputs2) bn2 = layers.BatchNormalization()(conv2) pool2 = layers.MaxPooling2D(pool_size=(3, 3), padding='same', strides=(3,3))(bn2) do2 = layers.Dropout(0.3)(pool2) # channel 3 inputs3 = layers.Input(shape=(42,42,1)) conv3 = layers.Conv2D(8, (5,5), padding='same', activation='relu', kernel_regularizer=l2(0.001))(inputs3) bn3 = layers.BatchNormalization()(conv3) pool3 = layers.MaxPooling2D(pool_size=(3, 3), padding='same', strides=(3,3))(bn3) do3 = layers.Dropout(0.3)(pool3) # merge 1 merged = layers.Concatenate()([do1, do2, do3]) # interpretation 1 merged_conv = layers.Conv2D(8, (5,5), padding='same', activation='relu', kernel_regularizer=l2(0.1))(merged) merged_pool = layers.MaxPooling2D(pool_size=(2, 2), padding='same', strides=(2,2))(merged_conv) flat = layers.Flatten()(merged_pool) flat_do = layers.Dropout(0.2)(flat) # outputs outputs = layers.Dense(1, activation='linear', name='spot')(flat_do) #Takes input u, v, os model = keras.models.Model(inputs=[inputs1, inputs2, inputs3], outputs=[outputs]) model.compile( loss={'spot':'mse'}, optimizer=opt, metrics={'spot':tf.keras.metrics.MeanAbsoluteError()}, ) return model 如何加入CBAM-ResNet模块
时间: 2023-08-30 19:06:53 浏览: 81
要加入CBAM-ResNet模块,可以按照以下步骤进行:
1.导入需要的库和模块:
```python
from tensorflow.keras import layers
from tensorflow.keras.regularizers import l2
```
2.定义CBAM模块:
```python
def cbam_block(cbam_feature, ratio=8):
# channel attention
cbam_feature_shape = cbam_feature.shape
avg_pool = layers.GlobalAveragePooling2D()(cbam_feature) # shape=(batch_size, channels)
dense1 = layers.Dense(cbam_feature_shape[-1]//ratio, activation='relu', kernel_initializer='he_normal', use_bias=True, bias_initializer='zeros')(avg_pool)
dense2 = layers.Dense(cbam_feature_shape[-1], activation='sigmoid', kernel_initializer='he_normal', use_bias=True, bias_initializer='zeros')(dense1)
reshaped_dense = layers.Reshape((1,1,cbam_feature_shape[-1]))(dense2)
cbam_feature = layers.multiply([cbam_feature, reshaped_dense])
# spatial attention
max_pool = layers.GlobalMaxPooling2D()(cbam_feature)
max_pool = layers.Reshape((1,1,cbam_feature_shape[-1]))(max_pool)
avg_pool = layers.GlobalAveragePooling2D()(cbam_feature)
avg_pool = layers.Reshape((1,1,cbam_feature_shape[-1]))(avg_pool)
concat = layers.Concatenate(axis=1)([max_pool, avg_pool])
cbam_feature = layers.Conv2D(filters=1, kernel_size=3, strides=1, padding='same', activation='sigmoid', kernel_initializer='he_normal', use_bias=False)(concat)
cbam_feature = layers.multiply([cbam_feature, cbam_feature])
return cbam_feature
```
3.在ResNet模型中加入CBAM模块:
```python
def CBAM_ResNet(opt):
# define input tensor
input_tensor = layers.Input(shape=(224, 224, 3))
# conv1
x = layers.Conv2D(filters=64, kernel_size=7, strides=2, padding='same', kernel_initializer='he_normal', use_bias=False)(input_tensor)
x = layers.BatchNormalization()(x)
x = layers.Activation('relu')(x)
x = layers.MaxPooling2D(pool_size=3, strides=2, padding='same')(x)
# conv2_x
x = conv_block(x, filters=[64, 64, 256], stage=2, block='a', strides=1)
x = cbam_block(x) # add CBAM module
x = identity_block(x, filters=[64, 64, 256], stage=2, block='b')
x = cbam_block(x) # add CBAM module
x = identity_block(x, filters=[64, 64, 256], stage=2, block='c')
x = cbam_block(x) # add CBAM module
# conv3_x
x = conv_block(x, filters=[128, 128, 512], stage=3, block='a')
x = cbam_block(x) # add CBAM module
x = identity_block(x, filters=[128, 128, 512], stage=3, block='b')
x = cbam_block(x) # add CBAM module
x = identity_block(x, filters=[128, 128, 512], stage=3, block='c')
x = cbam_block(x) # add CBAM module
x = identity_block(x, filters=[128, 128, 512], stage=3, block='d')
x = cbam_block(x) # add CBAM module
# conv4_x
x = conv_block(x, filters=[256, 256, 1024], stage=4, block='a')
x = cbam_block(x) # add CBAM module
x = identity_block(x, filters=[256, 256, 1024], stage=4, block='b')
x = cbam_block(x) # add CBAM module
x = identity_block(x, filters=[256, 256, 1024], stage=4, block='c')
x = cbam_block(x) # add CBAM module
x = identity_block(x, filters=[256, 256, 1024], stage=4, block='d')
x = cbam_block(x) # add CBAM module
x = identity_block(x, filters=[256, 256, 1024], stage=4, block='e')
x = cbam_block(x) # add CBAM module
x = identity_block(x, filters=[256, 256, 1024], stage=4, block='f')
x = cbam_block(x) # add CBAM module
# conv5_x
x = conv_block(x, filters=[512, 512, 2048], stage=5, block='a')
x = cbam_block(x) # add CBAM module
x = identity_block(x, filters=[512, 512, 2048], stage=5, block='b')
x = cbam_block(x) # add CBAM module
x = identity_block(x, filters=[512, 512, 2048], stage=5, block='c')
x = cbam_block(x) # add CBAM module
# average pool
x = layers.GlobalAveragePooling2D()(x)
# output layer
outputs = layers.Dense(units=1000, activation='softmax')(x)
# create model
model = keras.models.Model(inputs=input_tensor, outputs=outputs)
# compile model
model.compile(loss='categorical_crossentropy', optimizer=opt, metrics=['accuracy'])
return model
```
以上就是如何加入CBAM-ResNet模块的步骤,具体实现还需要根据自己的需求进行调整。
阅读全文
相关推荐
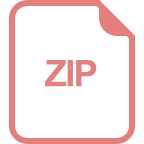
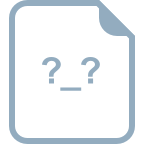
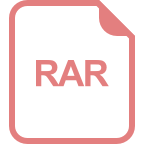









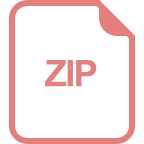
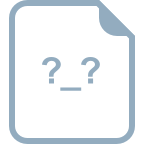
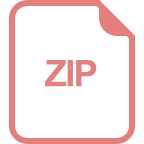
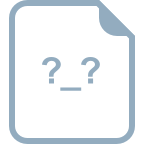