matlab中DQN算法
时间: 2023-11-30 22:41:24 浏览: 229
以下是MATLAB中实现DQN算法的基本步骤:
1. 定义神经网络模型:DQN算法使用深度神经网络来估计Q值函数。在MATLAB中,可以使用Deep Learning Toolbox来定义神经网络模型。
2. 初始化经验回放缓冲区:DQN算法使用经验回放缓冲区来存储智能体的经验。在MATLAB中,可以使用replayBuffer对象来实现经验回放缓冲区。
3. 初始化智能体:在MATLAB中,可以使用rlDQNAgent对象来初始化DQN智能体。需要指定神经网络模型、动作空间、状态空间、经验回放缓冲区等参数。
4. 训练智能体:在MATLAB中,可以使用train函数来训练DQN智能体。需要指定训练的轮数、每轮的步数、训练数据来源等参数。
5. 测试智能体:在MATLAB中,可以使用sim函数来测试DQN智能体。需要指定测试的轮数、每轮的步数、测试数据来源等参数。
以下是一个简单的MATLAB代码示例,用于实现DQN算法:
```matlab
% 定义神经网络模型
statePath = [
imageInputLayer([4 1 1],'Normalization','none','Name','state')
fullyConnectedLayer(24,'Name','fc1')
reluLayer('Name','relu1')
fullyConnectedLayer(24,'Name','fc2')
reluLayer('Name','relu2')
fullyConnectedLayer(2,'Name','output')];
actionPath = [
imageInputLayer([1 1 1],'Normalization','none','Name','action')
fullyConnectedLayer(24,'Name','fc3')];
concatPath = concatenationLayer(1,2,'Name','concat');
outputPath = [
fullyConnectedLayer(24,'Name','fc4')
reluLayer('Name','relu3')
fullyConnectedLayer(1,'Name','output')];
criticNetwork = layerGraph();
criticNetwork = addLayers(criticNetwork,statePath);
criticNetwork = addLayers(criticNetwork,actionPath);
criticNetwork = addLayers(criticNetwork,concatPath);
criticNetwork = addLayers(criticNetwork,outputPath);
criticNetwork = connectLayers(criticNetwork,'relu2','concat/in1');
criticNetwork = connectLayers(criticNetwork,'fc3','concat/in2');
% 初始化经验回放缓冲区
buf = replayBuffer(1e6);
% 初始化智能体
agent = rlDQNAgent(criticNetwork,agentOptions);
% 训练智能体
trainOpts = rlTrainingOptions(...
'MaxEpisodes',5000, ...
'MaxStepsPerEpisode',500, ...
'Verbose',false, ...
'Plots','training-progress', ...
'StopTrainingCriteria','AverageReward', ...
'StopTrainingValue',480);
trainResults = train(agent,env,trainOpts);
% 测试智能体
simOpts = rlSimulationOptions('MaxSteps',500);
simResults = sim(agent,env,simOpts);
```
相关推荐
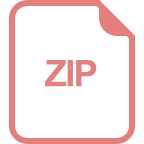













