Python如何根据列的三个关键词去提取结果
时间: 2024-03-20 09:43:52 浏览: 19
可以通过Pandas库中的DataFrame进行处理。假设有一个数据集df,其中有一列为"keywords",要提取其中包含"apple"、"banana"、"orange"的行,可以使用以下代码:
```
keywords = ['apple', 'banana', 'orange']
result = df[df['keywords'].str.contains('|'.join(keywords))]
```
其中,`str.contains()`函数用于判断字符串中是否包含关键词,`'|'.join(keywords)`用于将关键词列表以"|"拼接成一个正则表达式,表示匹配其中任意一个关键词。最终筛选出的结果存储在`result`中。
相关问题
python提取excel每行多个关键词并写入最后一列
### 回答1:
可以使用Python的pandas库来读取Excel文件,并使用apply函数遍历每一行,提取关键词并写入最后一列。具体代码如下:
```python
import pandas as pd
# 读取Excel文件
df = pd.read_excel('your_excel_file.xlsx')
# 定义提取关键词的函数
def extract_keywords(row):
# 假设关键词在第1列到第5列
keywords = []
for i in range(1, 6):
keyword = str(row[i]).strip() # 去除空格
if keyword:
keywords.append(keyword)
return ','.join(keywords)
# 应用函数并写入最后一列
df['关键词'] = df.apply(extract_keywords, axis=1)
# 保存Excel文件
df.to_excel('your_output_file.xlsx', index=False)
```
注意:需要将代码中的`your_excel_file.xlsx`替换为你的Excel文件名,将`your_output_file.xlsx`替换为你的输出文件名。
### 回答2:
要使用Python提取Excel每行中的多个关键词并将其写入到最后一列,你可以使用openpyxl库来操作Excel文件。首先,你需要安装openpyxl库,使用以下命令进行安装:
```
pip install openpyxl
```
接下来,你可以使用以下代码实现这个功能:
```python
import openpyxl
# 打开Excel文件
workbook = openpyxl.load_workbook('your_file.xlsx')
# 选择工作表
sheet = workbook.active
# 遍历每一行数据
for row in sheet.iter_rows(min_row=2, values_only=True): # 从第二行开始遍历,跳过标题行
keywords = [] # 存储关键词的列表
# 提取关键词,假设关键词在前三列
for i in range(3):
if row[i]:
keywords.append(row[i])
# 将关键词写入到最后一列
row[-1] = ', '.join(keywords)
# 保存修改后的Excel文件
workbook.save('your_file_modified.xlsx')
```
在这段代码中,我们首先使用`load_workbook`函数打开Excel文件,然后选择想要操作的工作表。使用`iter_rows`方法遍历每一行数据,`values_only=True`参数表示返回每个单元格的值而不是单元格对象。接下来,在每一行中提取关键词,假设关键词在前三列,存储到一个列表中。然后,使用`join`函数将关键词列表中的关键词拼接成一个字符串,并将其写入到最后一列。最后,使用`save`方法保存修改后的Excel文件。注意,你需要将代码中的文件名`your_file.xlsx`替换为你实际使用的Excel文件名。
### 回答3:
在Python中提取Excel每行中的多个关键词并将其写入最后一列,可以使用openpyxl库来实现。下面是一个简单的实现示例:
```
import openpyxl
# 打开Excel文件
wb = openpyxl.load_workbook('input.xlsx')
ws = wb.active
# 遍历每一行
for row in ws.iter_rows(min_row=2, values_only=True): # 从第二行开始遍历,values_only参数用于获取单元格的值而非公式
keywords = []
for cell_value in row[:-1]: # 遍历每一列(除了最后一列)
# 处理每个单元格的值,提取关键词并加入到关键词列表中
# 这里可以根据具体的需求使用字符串处理、正则表达式等方法提取关键词
# 这里仅作示例,提取包含大写字母的单词作为关键词
for word in cell_value.split():
if word.isupper():
keywords.append(word)
# 将关键词写入最后一列
keywords_str = ' '.join(keywords) # 将关键词列表拼接为以空格分隔的字符串
ws.cell(row=row[0].row, column=ws.max_column, value=keywords_str)
# 保存并关闭Excel文件
wb.save('output.xlsx')
wb.close()
```
上述代码中,我们首先使用openpyxl库打开Excel文件,并获取活动工作表。然后,我们使用`iter_rows`函数遍历每一行,其中`min_row=2`表示从第二行开始遍历,`values_only=True`表示获取单元格的值而不是公式。对于每一行,我们将遍历除了最后一列之外的每一列的值,并做相应的处理来提取关键词,这里仅作为示例提取包含大写字母的单词作为关键词。最后,我们将提取到的关键词拼接为以空格分隔的字符串,并将其写入最后一列。最后,保存并关闭Excel文件。
python文本关键词提取
Python中的文本关键词提取可以使用以下几种方法:
1.基于频率的关键词提取
最简单的关键词提取方式是基于频率的方法。通过统计每个词在文本中出现的频率,选取出现频率最高的词作为关键词。可以用Python中的nltk库来实现,具体步骤如下:
```
import nltk
from nltk.corpus import stopwords
from nltk.tokenize import word_tokenize
text = "The quick brown fox jumps over the lazy dog. The quick brown fox is very fast."
stop_words = set(stopwords.words('english'))
# 分词
words = word_tokenize(text)
# 去除停用词
words = [word for word in words if word.lower() not in stop_words]
# 构建频率分布
freq_dist = nltk.FreqDist(words)
# 打印前20个关键词及其频次
for word, frequency in freq_dist.most_common(20):
print(u'{}:{}'.format(word, frequency))
```
2.基于TF-IDF的关键词提取
TF-IDF是一种基于词频和文档频率的算法,用于评估文本重要程度。在文本关键词提取中,可以使用TF-IDF算法来提取关键词。可以用Python中的scikit-learn库来实现,具体步骤如下:
```
import pandas as pd
from sklearn.feature_extraction.text import TfidfVectorizer
text = ["The quick brown fox jumps over the lazy dog. The quick brown fox is very fast.",
"The lazy dog is very slow. The quick brown fox is very fast."]
# 初始化TF-IDF向量化器
tfidf_vectorizer = TfidfVectorizer(stop_words='english')
# 计算TF-IDF矩阵
tfidf_matrix = tfidf_vectorizer.fit_transform(text)
# 获取特征名
feature_names = tfidf_vectorizer.get_feature_names()
# 构建TF-IDF矩阵的数据框
tfidf_df = pd.DataFrame(tfidf_matrix.toarray(), columns=feature_names)
# 打印TF-IDF矩阵中的前20个关键词及其TF-IDF值
for i, row in tfidf_df.iterrows():
print(f"\nDocument {i+1}:")
print(row.nlargest(20))
```
3.基于LDA的关键词提取
LDA(Latent Dirichlet Allocation)是一种主题模型,可以将文本看作是由多个主题组成的。在文本关键词提取中,可以使用LDA算法来提取文本的主题以及与主题相关的关键词。可以用Python中的gensim库来实现,具体步骤如下:
```
import nltk
from gensim.models import LdaModel
from gensim.corpora import Dictionary
text = [["The", "quick", "brown", "fox", "jumps", "over", "the", "lazy", "dog."],
["The", "quick", "brown", "fox", "is", "very", "fast."],
["The", "lazy", "dog", "is", "very", "slow."]]
# 将单词转化为数字ID
documents = [Dictionary(text) for text in texts]
# 将文本转化为BoW向量
corpus = [dictionary.doc2bow(text) for text in texts]
# 训练LDA模型
lda_model = LdaModel(corpus=corpus, id2word=dictionary, num_topics=3)
# 打印LDA模型中的每个主题
for i in range(lda_model.num_topics):
print(f"Topic {i+1}:")
print(lda_model.print_topic(i))
```
以上三种方法提取出的文本关键词都可以在后续的文本分析任务中发挥重要作用。
相关推荐
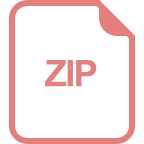
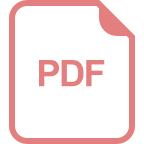
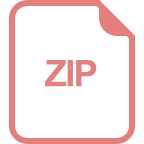












