tensorflow测试代码
时间: 2023-09-03 19:10:48 浏览: 44
以下是一个简单的 TensorFlow 测试代码,用于创建一个简单的神经网络并对 MNIST 数据集进行分类:
```python
import tensorflow as tf
from tensorflow.examples.tutorials.mnist import input_data
# Load MNIST dataset
mnist = input_data.read_data_sets("MNIST_data/", one_hot=True)
# Create placeholders for input images and labels
x = tf.placeholder(tf.float32, [None, 784])
y = tf.placeholder(tf.float32, [None, 10])
# Define variables for weights and biases
W = tf.Variable(tf.zeros([784, 10]))
b = tf.Variable(tf.zeros([10]))
# Define softmax regression model
y_pred = tf.nn.softmax(tf.matmul(x, W) + b)
# Define cross-entropy loss function
cross_entropy = tf.reduce_mean(-tf.reduce_sum(y * tf.log(y_pred), reduction_indices=[1]))
# Define optimizer
train_step = tf.train.GradientDescentOptimizer(0.5).minimize(cross_entropy)
# Initialize variables
init = tf.global_variables_initializer()
# Create session and run the graph
with tf.Session() as sess:
sess.run(init)
# Train the model for 1000 epochs
for i in range(1000):
batch_xs, batch_ys = mnist.train.next_batch(100)
sess.run(train_step, feed_dict={x: batch_xs, y: batch_ys})
# Test the model
correct_prediction = tf.equal(tf.argmax(y_pred, 1), tf.argmax(y, 1))
accuracy = tf.reduce_mean(tf.cast(correct_prediction, tf.float32))
print("Accuracy:", sess.run(accuracy, feed_dict={x: mnist.test.images, y: mnist.test.labels}))
```
这个代码使用 TensorFlow 实现了一个包含一个输入层、一个输出层和一个 softmax 回归模型的简单神经网络。模型被训练来对 MNIST 数据集中的手写数字进行分类,并在测试集上获得了一个准确率。
相关推荐
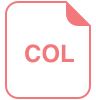
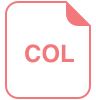
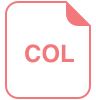
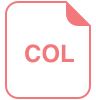









