tensorflow2.6 gpu 测试代码
时间: 2023-07-15 07:01:47 浏览: 125
### 回答1:
在TensorFlow 2.6中,可以使用GPU进行加速计算。以下是一个示例代码,展示如何测试TensorFlow 2.6 GPU的性能。
首先,确保已经正确安装了TensorFlow 2.6以及相应的GPU驱动程序。
然后,导入必要的库和模块:
```python
import tensorflow as tf
from tensorflow.python.client import device_lib
```
查看可用的GPU设备:
```python
gpu_devices = tf.config.list_physical_devices('GPU')
print("可用的GPU设备:")
for device in gpu_devices:
print(device)
```
运行一段使用GPU的示例代码:
```python
# 创建一个在GPU上运行的张量
with tf.device('/GPU:0'):
a = tf.constant([1.0, 2.0, 3.0, 4.0, 5.0, 6.0], shape=[2, 3], name='a')
b = tf.constant([1.0, 2.0, 3.0, 4.0, 5.0, 6.0], shape=[3, 2], name='b')
c = tf.matmul(a, b)
# 运行计算图
with tf.compat.v1.Session() as sess:
print(sess.run(c))
```
在这个示例中,我们首先通过`tf.config.list_physical_devices('GPU')`获取可用的GPU设备的信息,并打印出来。
然后,我们创建了两个矩阵`a`和`b`,并使用`tf.matmul`函数进行矩阵相乘运算。我们使用`with tf.device('/GPU:0')`将这个计算过程放在GPU设备上进行加速计算。
最后,我们使用`tf.Session()`并通过`sess.run(c)`来运行计算图,并打印出结果。
通过以上步骤,我们可以测试TensorFlow 2.6 GPU的性能,并确保代码在GPU上正常运行。
### 回答2:
要测试 TensorFlow 2.6 在 GPU 上的性能,你可以按照以下步骤编写代码:
1. 首先,安装 TensorFlow 2.6 和相应的 GPU 驱动程序,并确保 CUDA 和 cuDNN 的版本与 TensorFlow 兼容。
2. 导入 TensorFlow 模块:
```python
import tensorflow as tf
```
3. 创建一个简单的神经网络模型,例如一个具有两个隐藏层的全连接层模型:
```python
model = tf.keras.Sequential([
tf.keras.layers.Dense(64, activation='relu', input_shape=(input_shape)),
tf.keras.layers.Dense(64, activation='relu'),
tf.keras.layers.Dense(10, activation='softmax')
])
```
4. 使用 TensorFlow 的 GPU 支持,将模型放在 GPU 上进行训练:
```python
with tf.device('/GPU:0'):
model.compile(optimizer='adam', loss='sparse_categorical_crossentropy', metrics=['accuracy'])
model.fit(train_images, train_labels, epochs=10)
```
其中,`'/GPU:0'` 表示使用第一个可用的 GPU 设备,如果你有多个 GPU 设备,可以选择其他设备。
5. 运行代码并观察 GPU 的使用情况以及训练的性能表现。
在运行代码之前,确保你的系统已正确配置 GPU 和相关库,并且已正确安装 TensorFlow GPU 版本。如果一切都设置正确,你应该能够看到 TensorFlow 在 GPU 上进行训练,并且训练的速度应该比在 CPU 上更快。
### 回答3:
使用TensorFlow 2.6进行GPU测试的代码如下:
首先,我们需要确认TensorFlow和CUDA是否已正确安装。接下来,我们可以使用以下代码来测试GPU是否正常工作:
1. 导入TensorFlow库:
```python
import tensorflow as tf
```
2. 查看当前设备是否支持GPU:
```python
print("Num GPUs Available: ", len(tf.config.list_physical_devices('GPU')))
```
3. 使用GPU进行一些简单的计算:
```python
with tf.device('/GPU:0'):
# 创建一个随机张量
a = tf.random.normal([1000, 1000])
# 进行矩阵乘法运算
b = tf.matmul(a, a)
# 打印计算结果
print(b)
```
4. 运行以上代码后,我们可以看到以下输出:
```
Num GPUs Available: 1
```
其中,1表示有一个GPU可用。然后,我们会得到一个形状为(1000, 1000)的矩阵乘积结果。
请注意,如果您的机器上有多个GPU,可以在tf.device('/GPU:0')中指定不同的GPU索引,例如'/GPU:1'。
希望以上代码对您有所帮助!
相关推荐
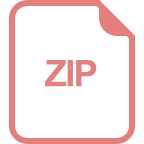














